Robust Loop Closure Detection Integrating Visual-Spatial-Semantic Information Via Topological Graphs And Cnn Features
REMOTE SENSING(2020)
Abstract
Loop closure detection is a key module for visual simultaneous localization and mapping (SLAM). Most previous methods for this module have not made full use of the information provided by images, i.e., they have only used the visual appearance or have only considered the spatial relationships of landmarks; the visual, spatial and semantic information have not been fully integrated. In this paper, a robust loop closure detection approach integrating visual-spatial-semantic information is proposed by employing topological graphs and convolutional neural network (CNN) features. Firstly, to reduce mismatches under different viewpoints, semantic topological graphs are introduced to encode the spatial relationships of landmarks, and random walk descriptors are employed to characterize the topological graphs for graph matching. Secondly, dynamic landmarks are eliminated by using semantic information, and distinctive landmarks are selected for loop closure detection, thus alleviating the impact of dynamic scenes. Finally, to ease the effect of appearance changes, the appearance-invariant descriptor of the landmark region is extracted by a pre-trained CNN without the specially designed manual features. The proposed approach weakens the influence of viewpoint changes and dynamic scenes, and extensive experiments conducted on open datasets and a mobile robot demonstrated that the proposed method has more satisfactory performance compared to state-of-the-art methods.
MoreTranslated text
Key words
loop closure detection, visual SLAM, semantic topology graph, graph matching, CNN features, deep learning
AI Read Science
Must-Reading Tree
Example
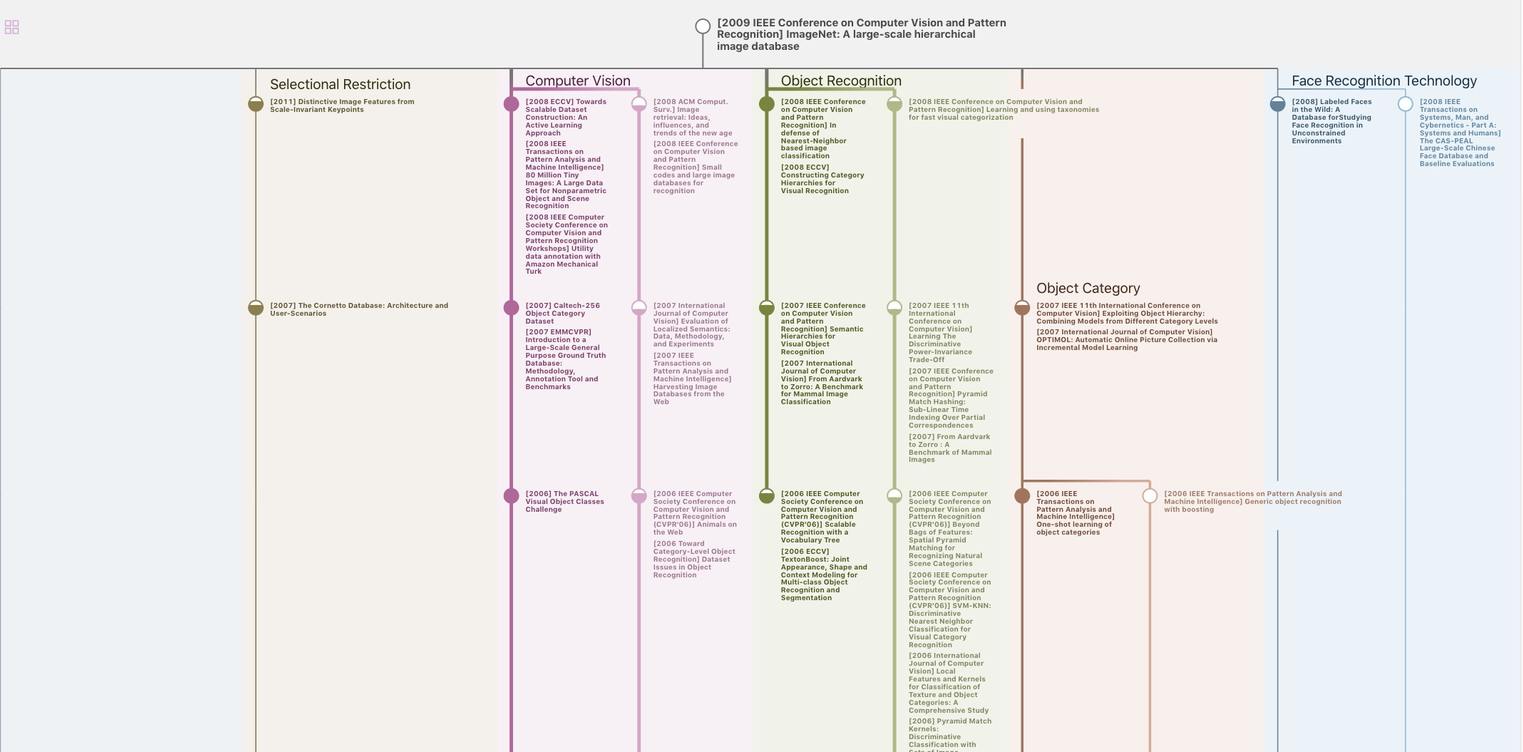
Generate MRT to find the research sequence of this paper
Chat Paper
Summary is being generated by the instructions you defined