Mask R-CNN Refitting Strategy for Plant Counting and Sizing in UAV Imagery.
REMOTE SENSING(2020)
摘要
This work introduces a method that combines remote sensing and deep learning into a framework that is tailored for accurate, reliable and efficient counting and sizing of plants in aerial images. The investigated task focuses on two low-density crops, potato and lettuce. This double objective of counting and sizing is achieved through the detection and segmentation of individual plants by fine-tuning an existing deep learning architecture called Mask R-CNN. This paper includes a thorough discussion on the optimal parametrisation to adapt the Mask R-CNN architecture to this novel task. As we examine the correlation of the Mask R-CNN performance to the annotation volume and granularity (coarse or refined) of remotely sensed images of plants, we conclude that transfer learning can be effectively used to reduce the required amount of labelled data. Indeed, a previously trained Mask R-CNN on a low-density crop can improve performances after training on new crops. Once trained for a given crop, the Mask R-CNN solution is shown to outperform a manually-tuned computer vision algorithm. Model performances are assessed using intuitive metrics such as Mean Average Precision (mAP) from Intersection over Union (IoU) of the masks for individual plant segmentation and Multiple Object Tracking Accuracy (MOTA) for detection. The presented model reaches an mAP of0.418for potato plants and0.660for lettuces for the individual plant segmentation task. In detection, we obtain a MOTA of0.781for potato plants and0.918for lettuces.
更多查看译文
关键词
UAV,crop mapping,image analysis,precision agriculture,deep learning,individual plant segmentation,plant detection,transfer learning
AI 理解论文
溯源树
样例
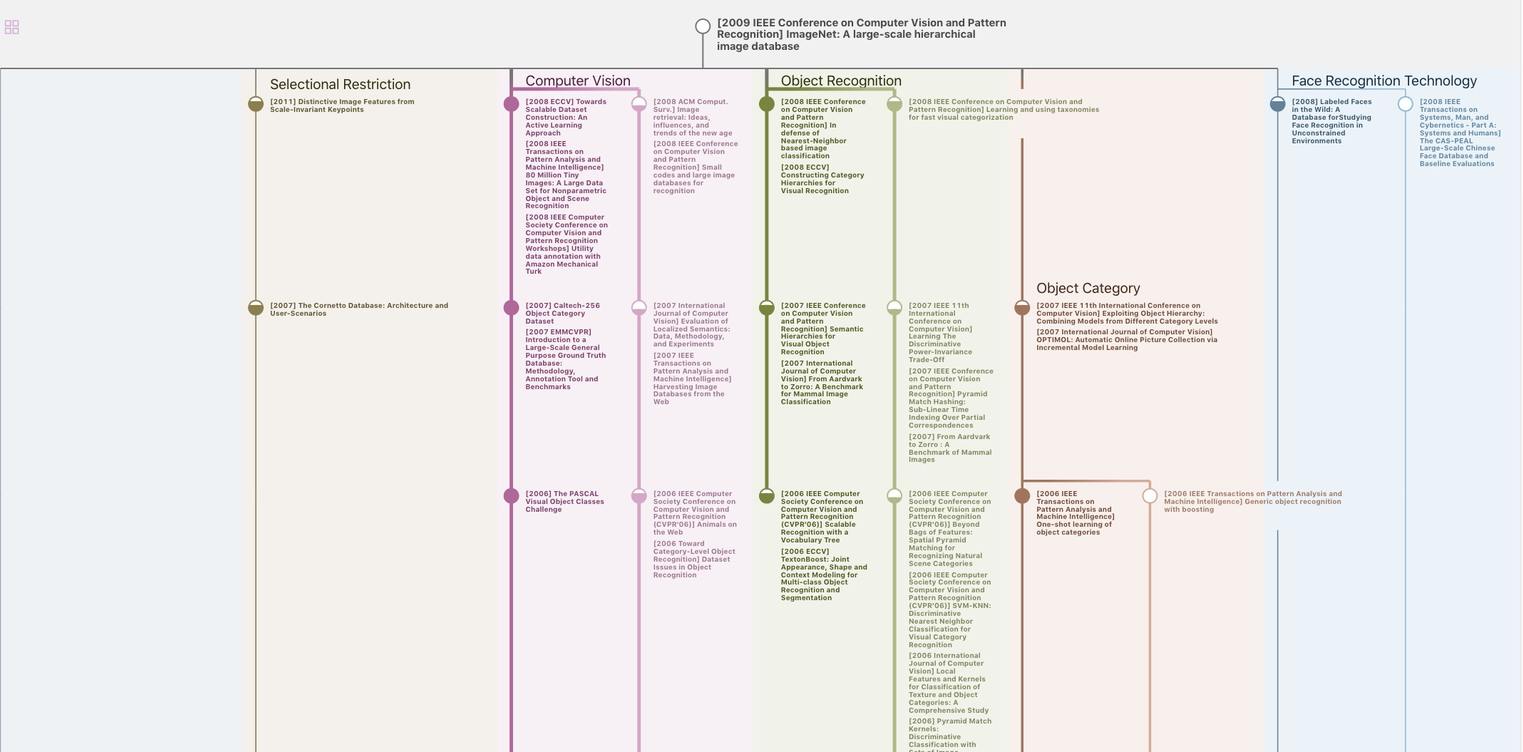
生成溯源树,研究论文发展脉络
Chat Paper
正在生成论文摘要