Benders Decomposition for the Profit Maximizing Capacitated Hub Location Problem with Multiple Demand Classes.
TRANSPORTATION SCIENCE(2020)
摘要
This paper models the profit maximizing capacitated hub location problem with multiple demand classes to determine an optimal hub network structure that allocates available capacities of hubs to satisfy demand for commodities from different market segments. A strong deterministic formulation of the problem is presented, and a Benders reformulation is described to optimally solve large-size instances of the problem. A new two-phase methodology is developed to decompose the Benders subproblem, and two effective separation routines are derived to strengthen the Benders optimality cuts. The algorithm is enhanced by the integration of improved variable-fixing techniques. The deterministic model is further extended by considering uncertainty associated with the demand to develop a two-stage stochastic program. To solve the stochastic version, a Monte Carlo simulation-based algorithm is developed that integrates a sample average approximation scheme with the proposed Benders decomposition algorithm. Novel acceleration techniques are presented to improve the convergence of the algorithm proposed for the stochastic version. The efficiency and robustness of the algorithms are evaluated through extensive computational experiments. Computational results show that largescale instances with up to 500 nodes and three demand classes can be solved to optimality, and that the proposed separation routines generate cuts that provide significant speedups compared with using Pareto-optimal cuts. The developed two-phase methodology for solving the Benders subproblem as well as the variable-fixing and acceleration techniques can be used to solve other discrete location and network design problems.
更多查看译文
关键词
hub location,Benders decomposition,variable fixing,acceleration techniques,sample average approximation
AI 理解论文
溯源树
样例
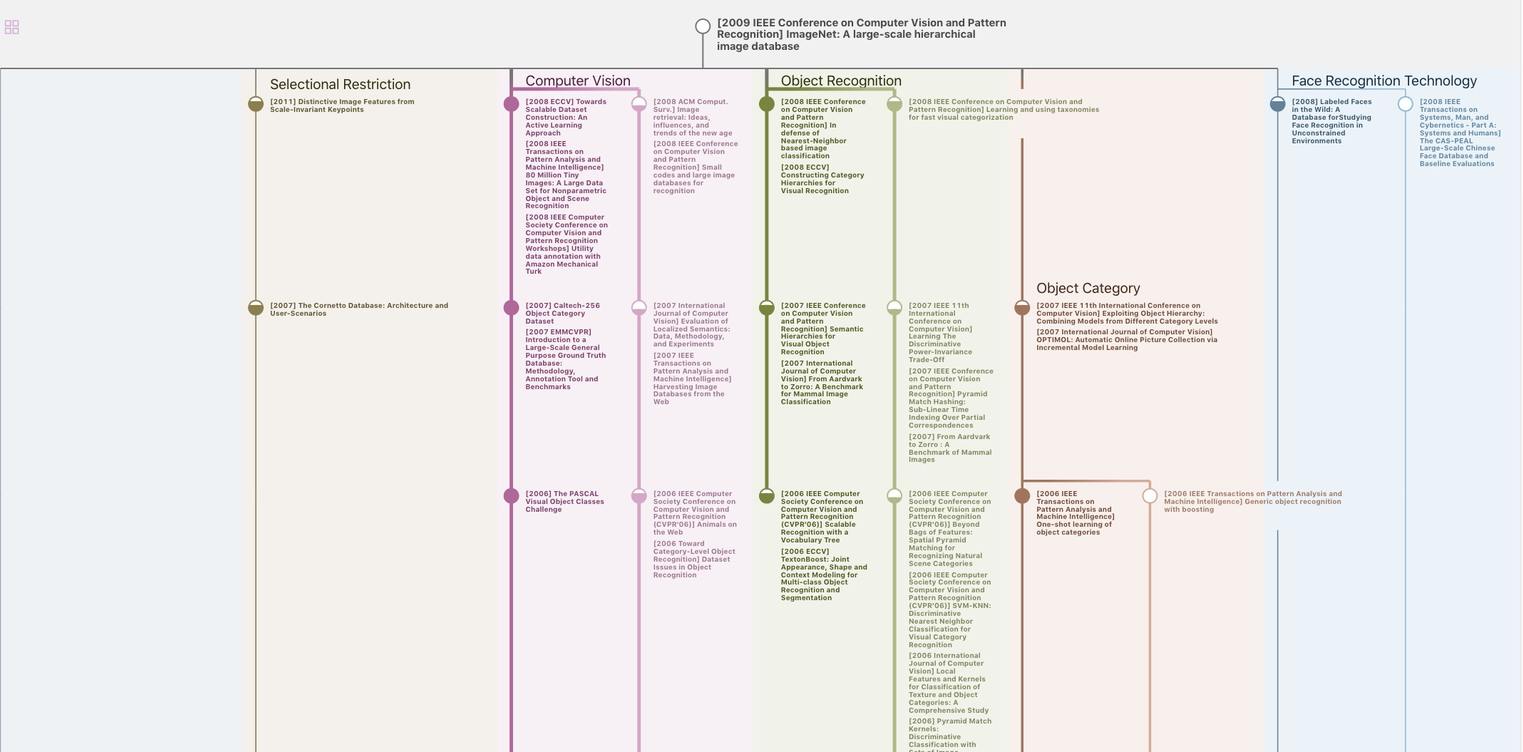
生成溯源树,研究论文发展脉络
Chat Paper
正在生成论文摘要