A Personalized Classifier for Human Motion Activities With Semi-Supervised Learning
Periodicals(2020)
Abstract
AbstractThe motion activities performed by a mobile or wearable device user are characteristic of the user and the accuracy of detection of motion activity context can be improved by incorporating personalized samples of motion activity. But obtaining personalized samples of motion activity from device user is not practically feasible. In this article, a semi-supervised method is presented for improving the classification accuracy of the mobile or wearable device user’s motion activities by deriving a classifier that is personalized to the user starting from a factory-set generalized classifier. The novelty lies in using information theoretic criterion to select personalized data samples from all the personalized data samples collected from the target user. This is useful in improving the motion activity detection accuracy, since each device user has a distinct gait personality as compared to the motion activity data patterns available in a generalized database that are used to train a generalized classifier. The average accuracy for 11 target users of a generalized classifier is 93.11% that increases to 96.50% using the proposed method when the device is used in in-hand mode. It is observed that the accuracy improvement with a personalized classifier over a generalized classifier is greater for those subjects whose accuracy is lower than others with the generalized classifier.
MoreTranslated text
Key words
Data models, Wearable computers, Sensors, Training, Performance evaluation, Adaptation models, Mobile handsets, Information theoretic criteria, generalized classifier, motion activities, mobile or wearable device, personalized classifier, semi-supervised learning
AI Read Science
Must-Reading Tree
Example
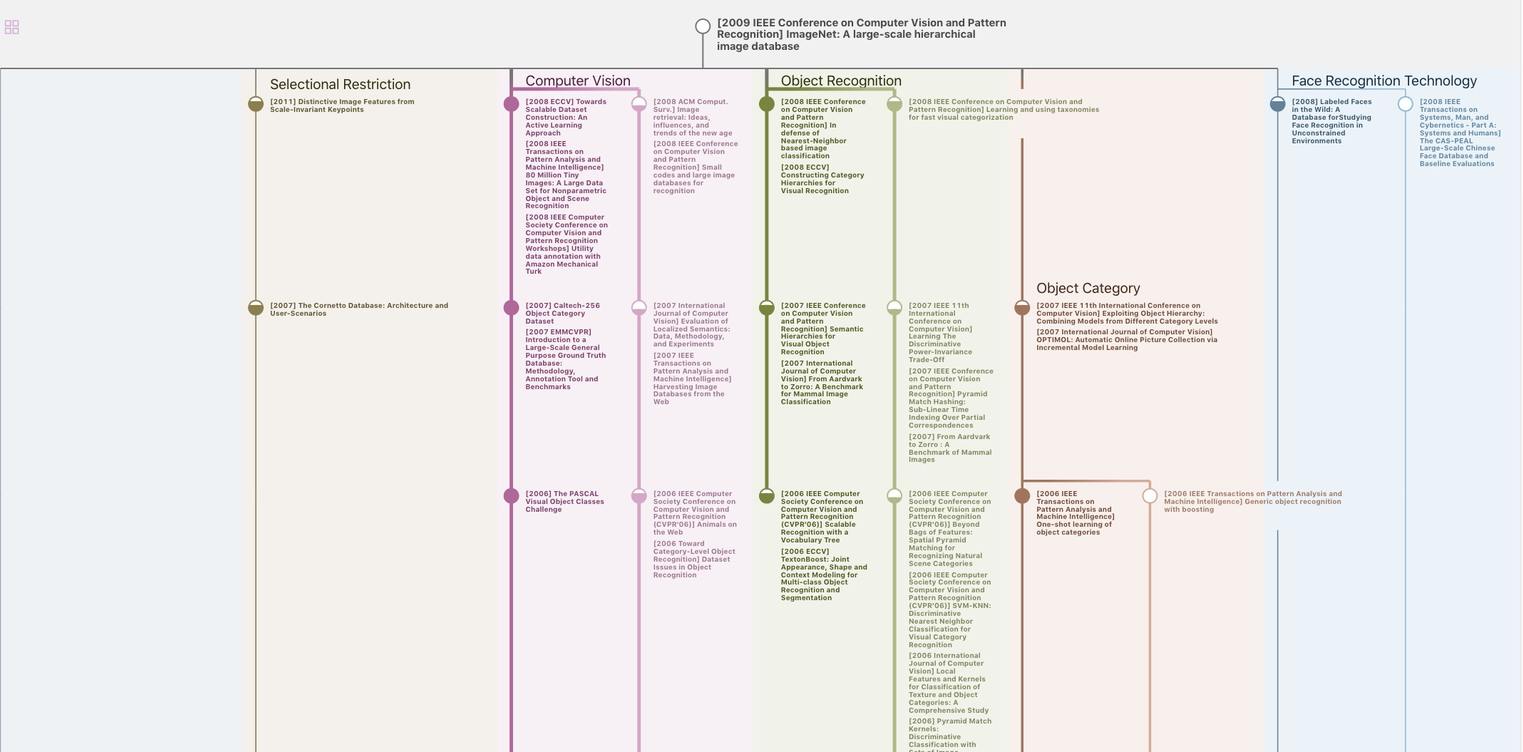
Generate MRT to find the research sequence of this paper
Chat Paper
Summary is being generated by the instructions you defined