A Robust Illumination-Invariant Camera System for Agricultural Applications
2021 IEEE/RSJ INTERNATIONAL CONFERENCE ON INTELLIGENT ROBOTS AND SYSTEMS (IROS)(2021)
摘要
Object detection and semantic segmentation are two of the most widely adopted deep learning algorithms in agricultural applications. One of the major sources of variability in image quality acquired outdoors for such tasks is changing lighting conditions that can alter the appearance of the objects or the contents of the entire image. While transfer learning and data augmentation reduce the need for large amount of data to train deep neural networks to some extent, the large variety of cultivars and the lack of shared datasets in agriculture makes wide-scale field deployments difficult. In this paper, we present an active lighting-based camera system that generates robust and uniform images in any lighting conditions. We provide extensive validation experiments to evaluate the consistency in the quality of the images. Metrics for assessing image uniformity like Structural Similarity (SSIM) index and the Peak Signal to Noise Ratio (PSNR) ranged from 83.78 to 93.79 and from 25.30 to 31.78 respectively, showing stability over a day with changing sunlight. The validation stage also showed that the generated images effectively reduce the amount of training samples for object detection using deep neural networks. The camera system was then deployed in real field experiments for counting buds in dormant vines and shoots in early season grape vines as well as for counting apples in orchards. The mean absolute errors obtained when compared to ground truth were 5%, 2.55% and 8.57%, respectively.
更多查看译文
关键词
deep neural networks,robust illumination-invariant camera system,agricultural applications,object detection,semantic segmentation,deep learning algorithms,image quality,lighting conditions,transfer learning,active lighting-based camera system,structural similarity index,data augmentation,peak signal to noise ratio
AI 理解论文
溯源树
样例
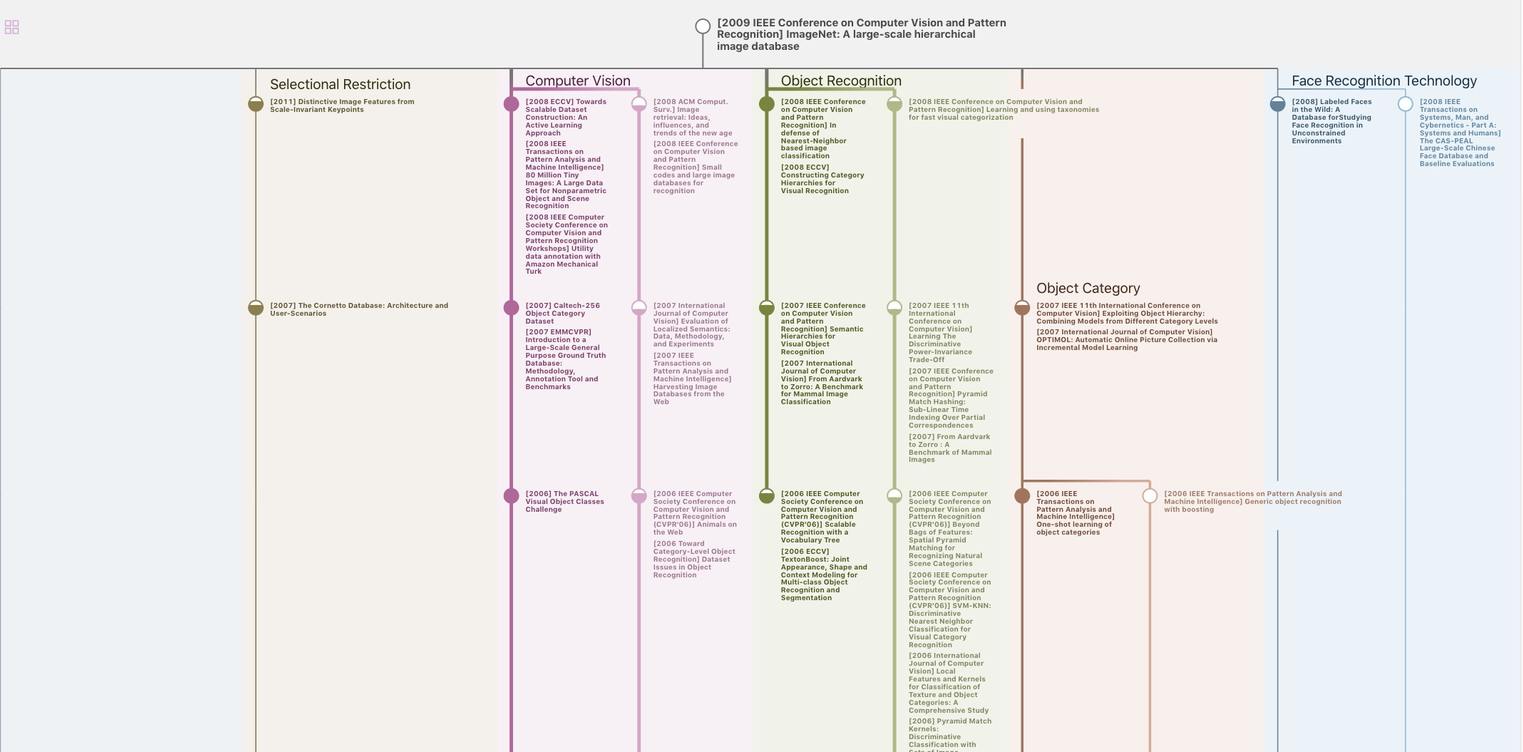
生成溯源树,研究论文发展脉络
Chat Paper
正在生成论文摘要