On Optimization Techniques for the Construction of an Exponential Estimate for Delayed Recurrent Neural Networks.
SYMMETRY-BASEL(2020)
摘要
This work is devoted to the modeling and investigation of the architecture design for the delayed recurrent neural network, based on the delayed differential equations. The usage of discrete and distributed delays makes it possible to model the calculation of the next states using internal memory, which corresponds to the artificial recurrent neural network architecture used in the field of deep learning. The problem of exponential stability of the models of recurrent neural networks with multiple discrete and distributed delays is considered. For this purpose, the direct method of stability research and the gradient descent method is used. The methods are used consequentially. Firstly we use the direct method in order to construct stability conditions (resulting in an exponential estimate), which include the tuple of positive definite matrices. Then we apply the optimization technique for these stability conditions (or of exponential estimate) with the help of a generalized gradient method with respect to this tuple of matrices. The exponential estimates are constructed on the basis of the Lyapunov-Krasovskii functional. An optimization method of improving estimates is offered, which is based on the notion of the generalized gradient of the convex function of the tuple of positive definite matrices. The search for the optimal exponential estimate is reduced to finding the saddle point of the Lagrange function.
更多查看译文
关键词
recurrent neural network,delayed differential equations,exponential estimation,optimization method,generalized gradient
AI 理解论文
溯源树
样例
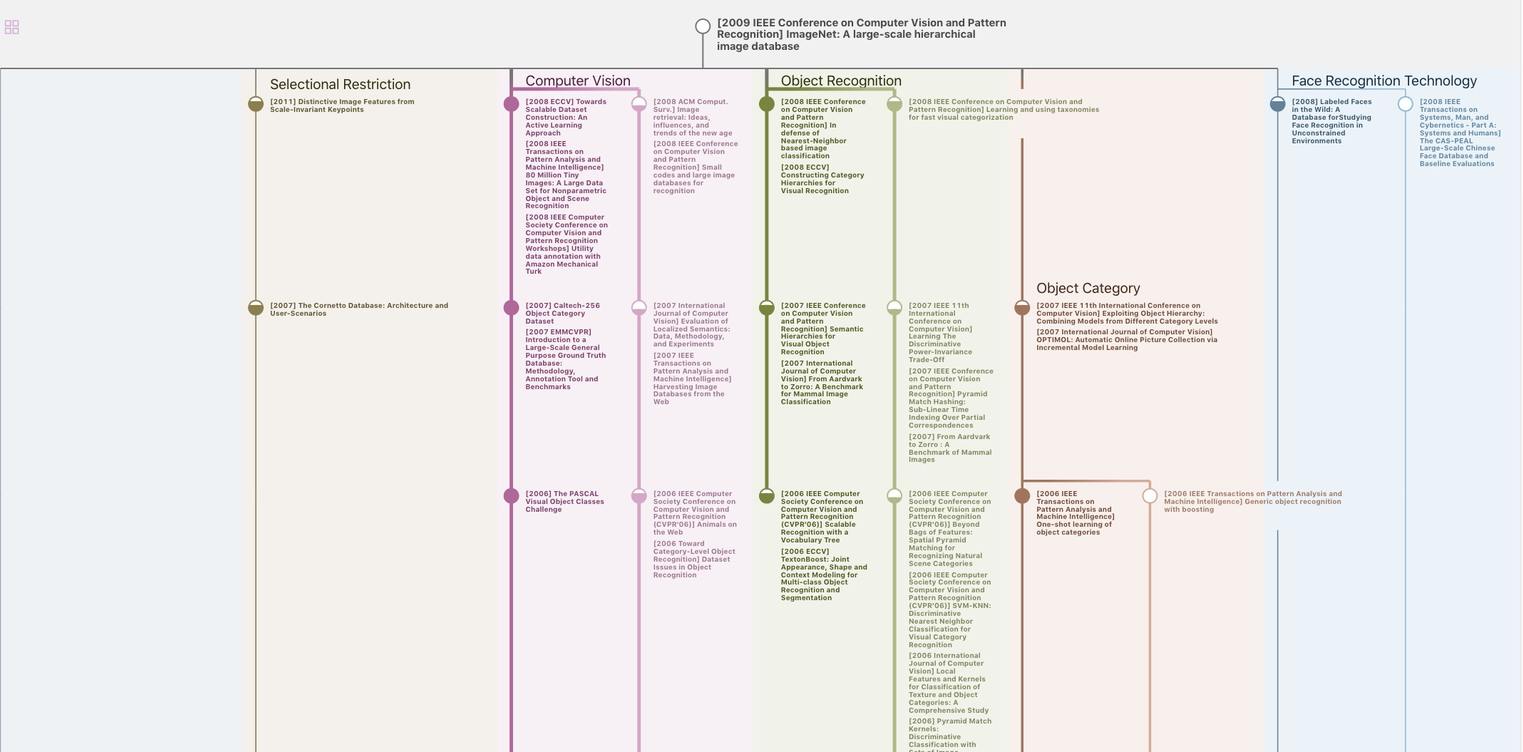
生成溯源树,研究论文发展脉络
Chat Paper
正在生成论文摘要