Axle Temperature Monitoring and Neural Network Prediction Analysis for High-Speed Train under Operation.
SYMMETRY-BASEL(2020)
摘要
Predicting the axle temperature states of the high-speed train under operation in advance and evaluating working states of axle bearings is important for improving the safety of train operation and reducing accident risks. The method of monitoring the axle temperature of a train under operation, combined with the neural network prediction method, was applied. A total of 36 sensors were arranged at key positions such as the axle bearings of the train gearbox and the driving end of the traction motor. The positions of the sensors were symmetrical. Axle temperature measurements over 11 days with more than 38,000 km were obtained. The law of the change of the axle temperature in each section was obtained in different environments. The resultant data from the previous 10 days were used to train the neural network model, and a total of 800 samples were randomly selected from eight typical locations for the prediction of axle temperature over the following 3 min. In addition, the results predicted by the neural network method and the GM (1,1) method were compared. The results show that the predicted temperature of the trained neural network model is in good agreement with the experimental temperature, with higher precision than that of the GM (1,1) method, indicating that the proposed method is sufficiently accurate and can be a reliable tool for predicting axle temperature.
更多查看译文
关键词
high-speed train,axle temperature prediction,neural networks,random sampling
AI 理解论文
溯源树
样例
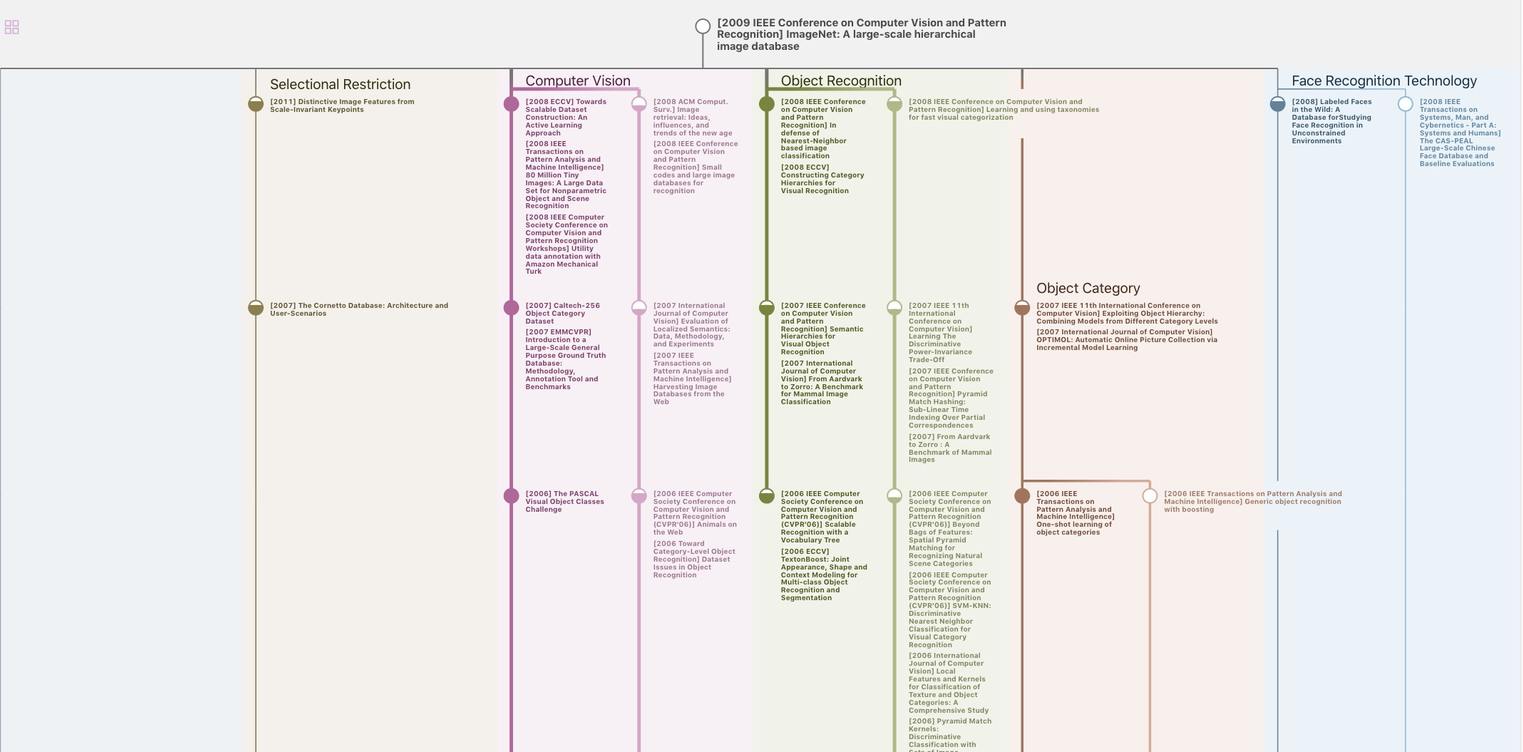
生成溯源树,研究论文发展脉络
Chat Paper
正在生成论文摘要