Hardware-Algorithm Co-Design of a Compressed Fuzzy Active Learning Method
IEEE Transactions on Circuits and Systems I: Regular Papers(2020)
Abstract
Active learning method (ALM) is a powerful fuzzy-based soft computing methodology suitable for various applications such as function modeling, control systems, clustering and classification. Despite considerable advantages, the main computational engine of ALM, ink drop spread (IDS), is memory-intensive, which imposes significant area overheads in the hardware realization of the ALM for real-time applications. In this paper, we propose a compressed model for ALM which greatly alleviates the storage limitations. The proposed approach employs a distinct inference algorithm, enabling a significant reduction in memory utilization from O(N
2
) to O(2N) for a multi-input single-output (MISO) system. Also, the computational costs in both training and inference modes are decreased to only a few additions and multiplications. Furthermore, we develop a memory-efficient digital architecture for the proposed compressed ALM algorithm that can be leveraged for various computing systems through configuring a few registers. Finally, we assess the performance of the proposed approach using various function modeling and classification applications and provide a comparison with conventional ALM and some other well-know approaches. Simulation and hardware implementation results demonstrate that the proposed approach achieves reduced noise sensitivity with 128× reduction in the average memory usage while realizing comparable accuracy compared to the other approaches studied herein.
MoreTranslated text
Key words
Active learning method (ALM),ink drop spread (IDS),compressed ALM,FPGA
AI Read Science
Must-Reading Tree
Example
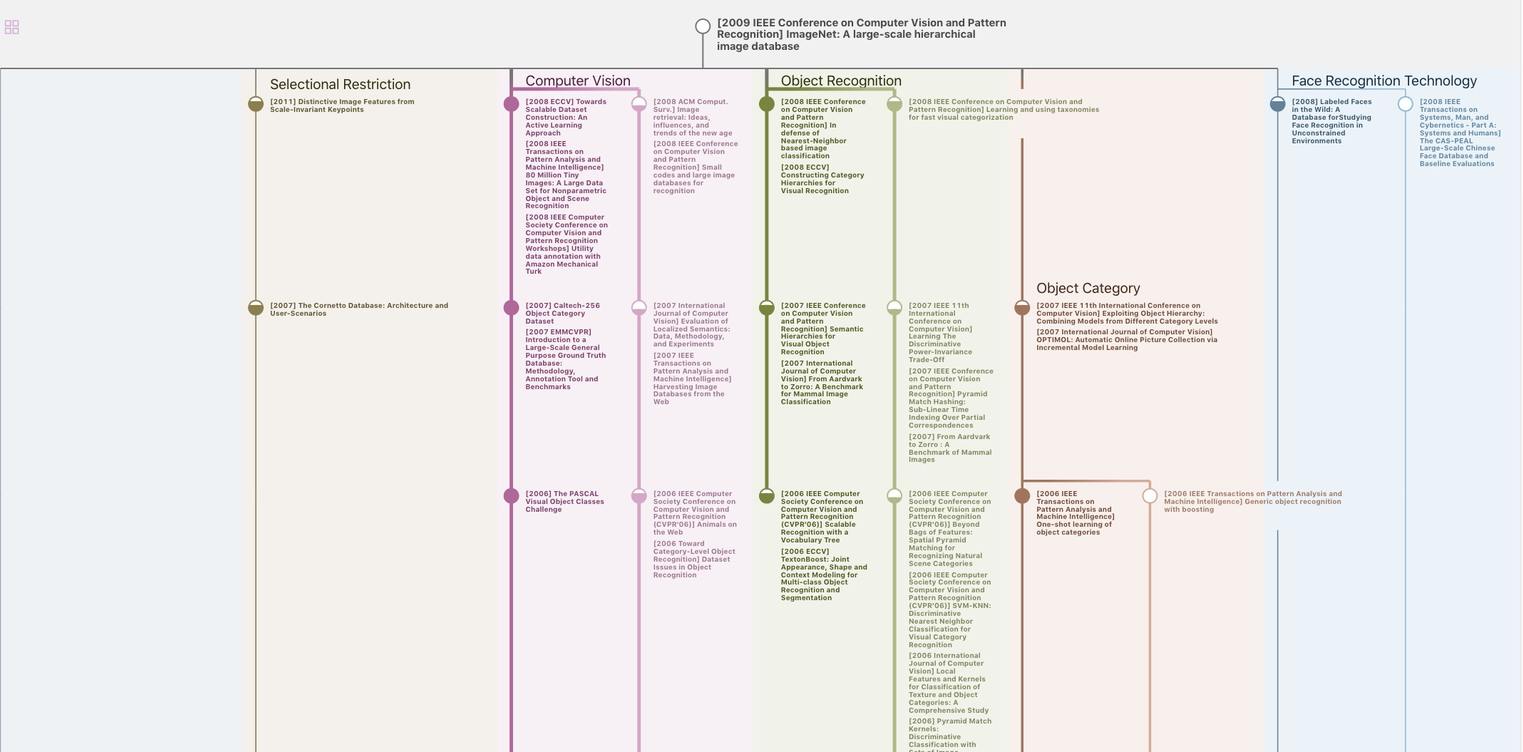
Generate MRT to find the research sequence of this paper
Chat Paper
Summary is being generated by the instructions you defined