Brain Tumor Segmentation using an Encoder-Decoder Network with a Multiscale Feature Module
PROCEEDINGS OF THE XXII 2020 IEEE INTERNATIONAL AUTUMN MEETING ON POWER, ELECTRONICS AND COMPUTING (ROPEC 2020), VOL 4(2020)
摘要
The correct identification of brain tumors in multisequence images is a very challenging task and of high relevance in the correct treatment of patients affected by this illness. In this paper, we present a segmentation neural network approach named FPM-BrainSeg. FPM-BrainSeg incorporates important features on its architecture such as residual connections, anisotropic convolutions, instance normalization, and a Feature Pooling Module (FPM) that increases the field of view of the extracted features. We compare the performance of FPM-BrainSeg with three state-of-the-art segmentation networks trained over a common Graphic Processing Unit (GPU) of the GTX family, by using the BraTS 2018 dataset. The performance obtained with FPM-BrainSeg surpassed the other neural models. The features extracted by the FPM increase the ability of the network to segment the different tumor regions as demonstrated in the visualization of the activation layers. Furthermore, a second comparison of our approach with state-of-the-art models (as presented in their original papers), demonstrate that our architecture attained competitive performance.
更多查看译文
关键词
brain tumor segmentation,encoder-decoder network,segmentation neural network,FPM-BrainSeg,Feature Pooling Module,residual connections,anisotropic convolutions,instance normalization,feature extraction,graphic processing unit,GTX family
AI 理解论文
溯源树
样例
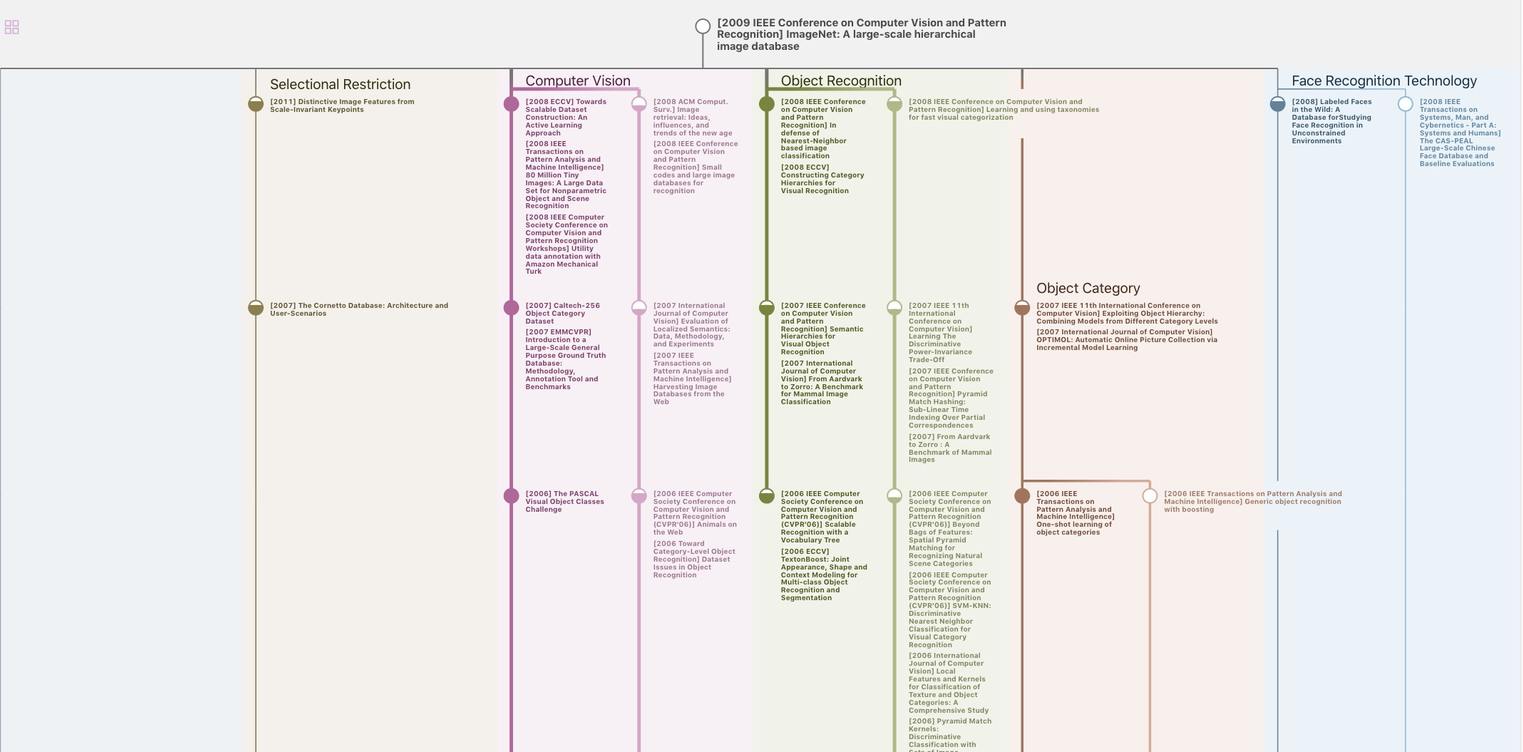
生成溯源树,研究论文发展脉络
Chat Paper
正在生成论文摘要