Productivity forecast for multi-stage fracturing in shale gas wells based on a random forest algorithm
Energy Sources Part A-recovery Utilization and Environmental Effects(2020)
摘要
This study addresses the problem of low-accuracy production forecasting for horizontal shale gas wells by applying a random forest algorithm and considering both the physical reservoir parameters and the engineering fracturing parameters. Based on the interpretation of logging data, drilling data, fracturing data, and sectional production data from the Fuling shale gas field, the random forest algorithm was used to build a regression model, through partial correlation analysis and recursive feature elimination to select the optimal key influencing factors. The study revealed that the layer, cluster spacing, number of clusters, 40/70 mesh low-density ceramsite (medium sand), and total proppant are the key factors influencing sectional production, and they all have different effects on it. The random forest algorithm showed better prediction capacity than the other algorithms. Its coefficient of determination and root-mean-square error on the test set were 0.723 and 0.319, respectively, which indicates that it is an effective approach and has good generalization ability. The prediction results of the productivity regression model based on the random forest algorithm, combined with the trend surface response analysis, were used to obtain the optimal values of the six key fracturing parameters of the unfractured well.
更多查看译文
关键词
Shale gas,production prediction,multi-stage fracturing,random forest,non-parametric statistics
AI 理解论文
溯源树
样例
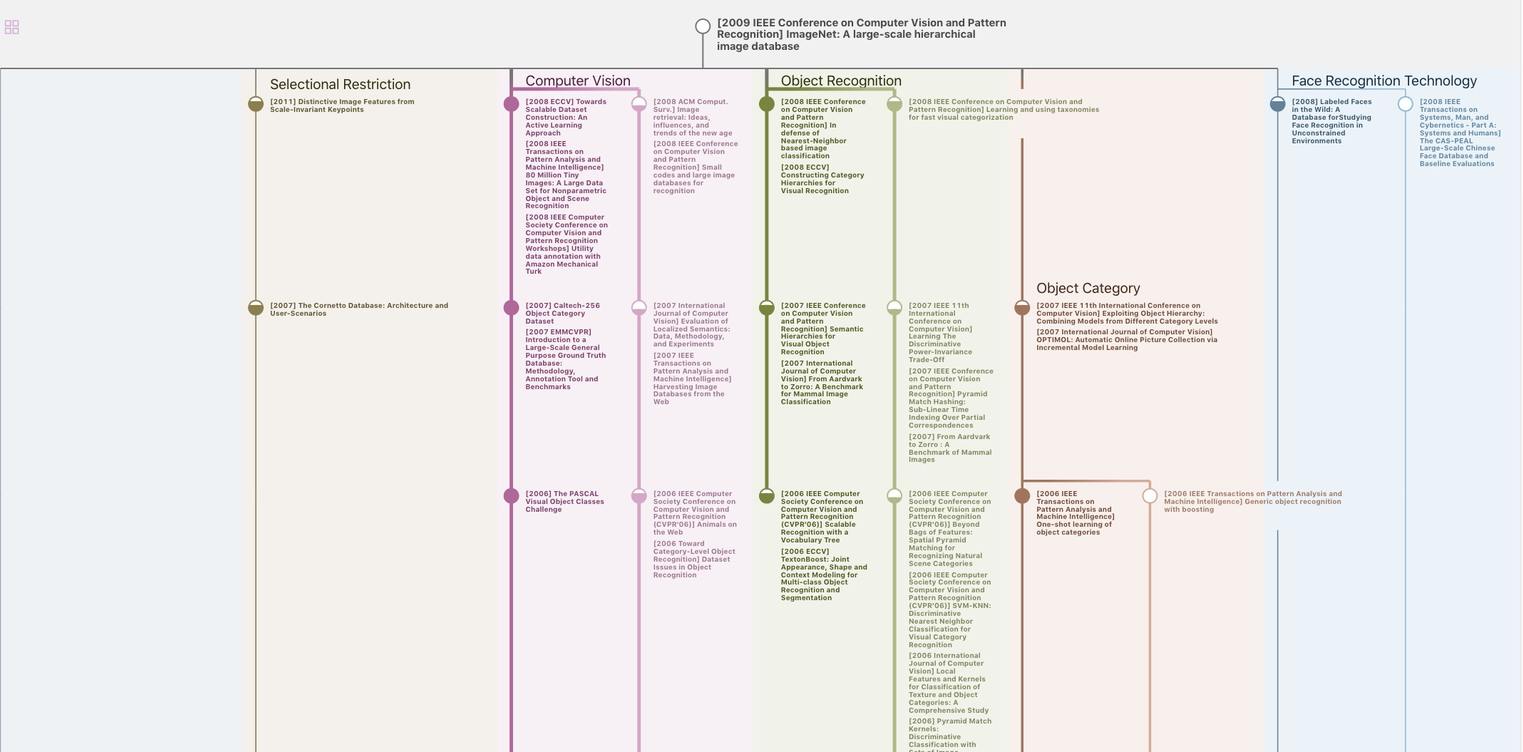
生成溯源树,研究论文发展脉络
Chat Paper
正在生成论文摘要