Reinforcement Learning Based Beamforming Jammer For Unknown Wireless Networks
IEEE ACCESS(2020)
摘要
A jamming attack refers to adversarial activities to cause an interruption of communication among legitimate nodes in a wireless network by transmitting a jamming signal. Among various jamming techniques, network jamming is an attack technique that is performed to maximize the jamming impact on the entire network within the capability of the jammer. In this article, we consider a jamming attack to an unknown wireless network where no a priori network information is provided to the jammer except the radio frequency signal information acquired by overhearing the shared wireless channel. To increase the impact of a jamming attack on an unknown network, we propose a reinforcement learning based beamforming attack strategy. In the proposed attack, a jammer learns the beam direction and angle width to maximize the impact of jamming using the multi-armed bandit technique. As a reward for reinforcement learning, we develop a new metric that can quantitatively evaluate the impact of a jamming attack by measuring the statistical change of the channel busy times before and after each attack. Through extensive simulations, we evaluate the performance of the proposed jamming strategy in an unknown wireless network.
更多查看译文
关键词
Jamming, Array signal processing, Wireless networks, Reinforcement learning, Transmitting antennas, Network topology, Degradation, Jamming, beamforming, unknown networks, learning, multi armed bandits
AI 理解论文
溯源树
样例
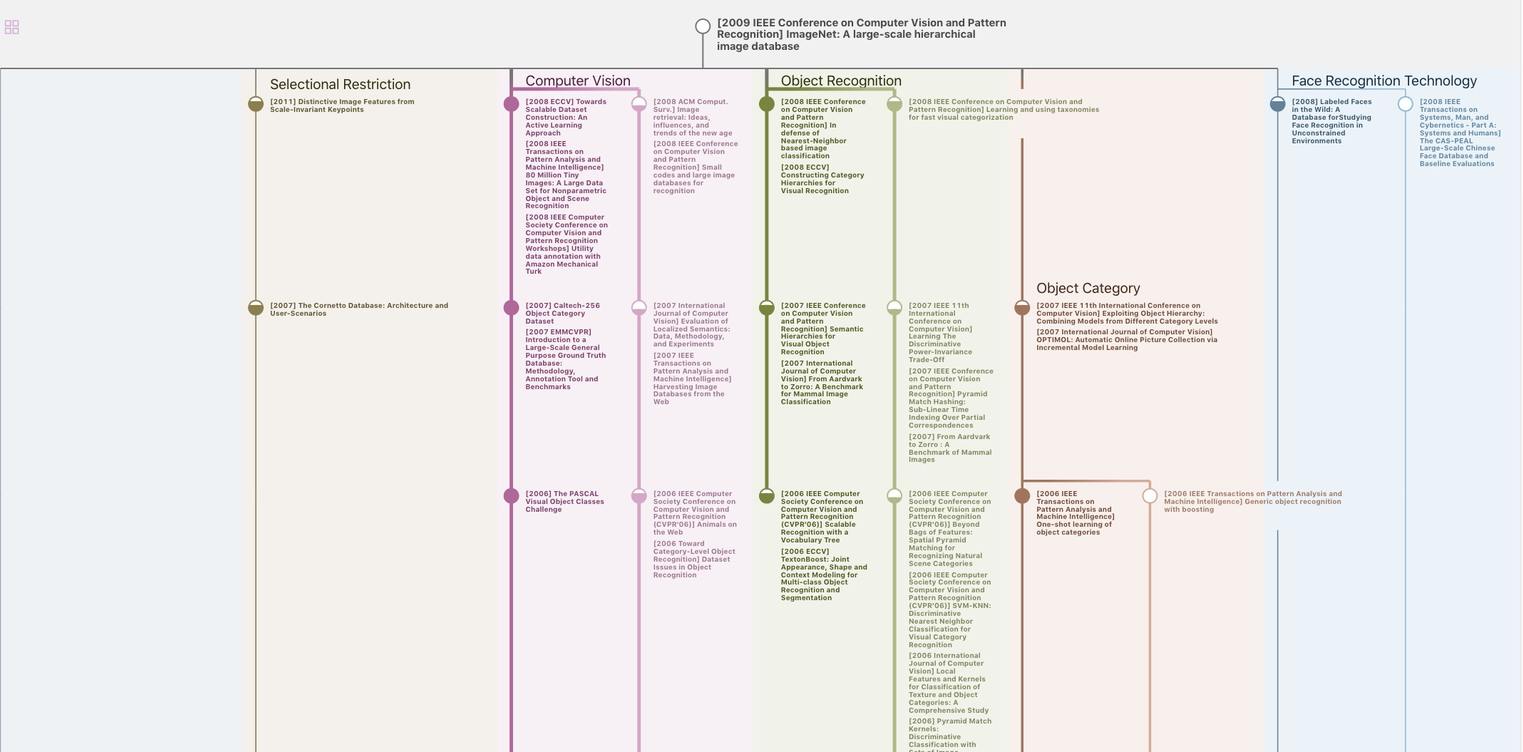
生成溯源树,研究论文发展脉络
Chat Paper
正在生成论文摘要