Fast Stepwise Regression Based On Multidimensional Indexes
INFORMATION SCIENCES(2021)
摘要
We present an approach to efficiently construct stepwise regression models in a very high dimensional setting using a multidimensional index. The approach is based on an observation that the collections of available predictor variables often remain relatively stable and many models are built based on the same predictors. Example scenarios include data warehouses against which multiple ad hoc analytical models are built or collections of publicly available open data which remain relatively fixed and are used as a source of predictor variables for many models. We propose an approach where the user simply provides a target variable and the algorithm uses a pre-built multidimensional index to automatically select predictors from millions of available variables, yielding results identical to standard stepwise regression, but an order of magnitude faster. The algorithm has been tested on the large statistical database available from Eurostat, and has been demonstrated to produce interpretable and accurate models. We demonstrate experimentally that our approach produces results that are significantly better than other approaches to modeling with ultrahigh dimensional data. Finally, we discuss potential pitfalls such as the presence of highly correlated variables, and show how they can be overcome. (C) 2020 Elsevier Inc. All rights reserved.
更多查看译文
关键词
Stepwise regression, Forward regression, Feature selection, Variable screening, Multidimensional indexing, Open Data
AI 理解论文
溯源树
样例
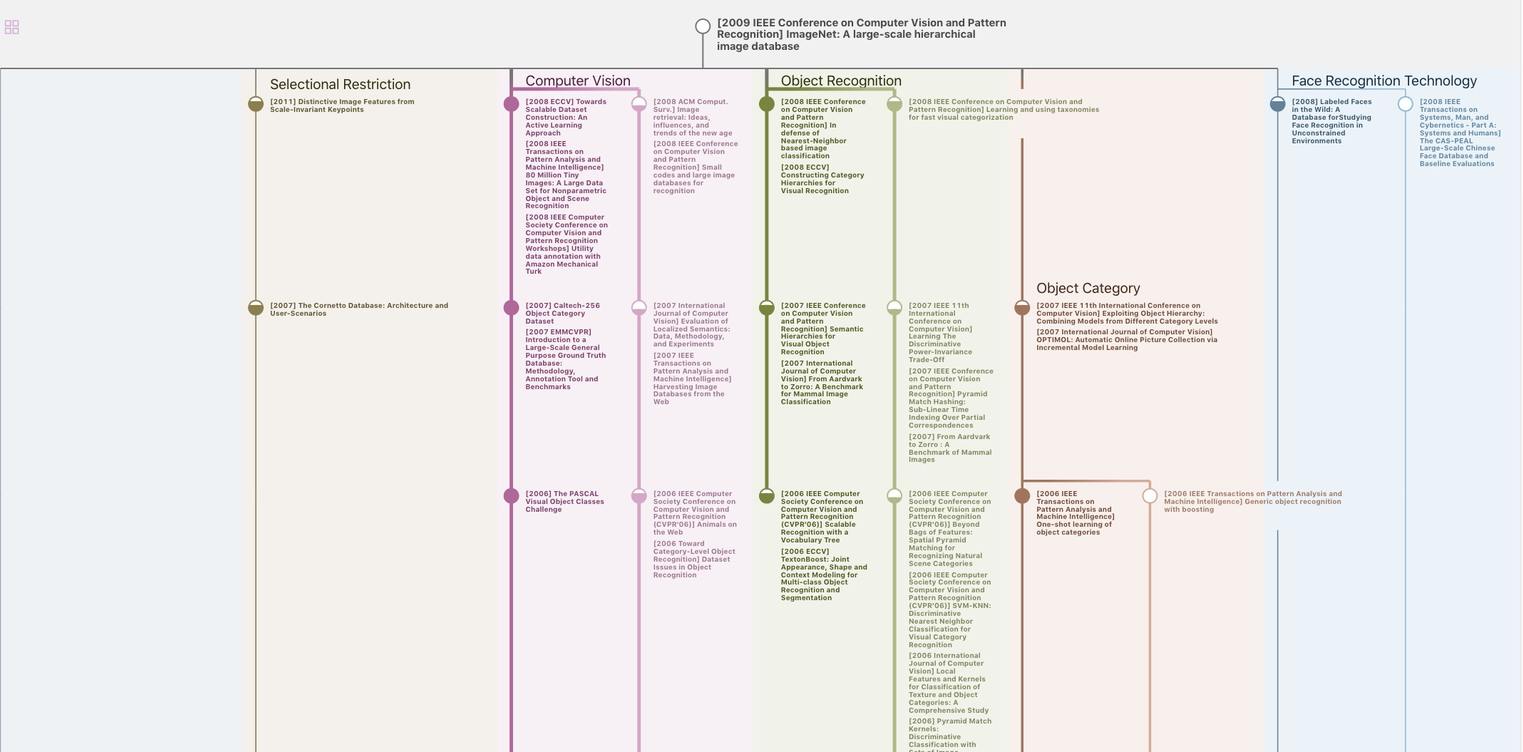
生成溯源树,研究论文发展脉络
Chat Paper
正在生成论文摘要