Reduced-Rank L1-Norm Principal-Component Analysis With Performance Guarantees
IEEE Transactions on Signal Processing(2021)
摘要
Standard Principal-Component Analysis (PCA) is known to be sensitive to outliers among the processed data. On the other hand, L1-norm-based PCA (L1-PCA) exhibits sturdy resistance against outliers, while it performs similar to standard PCA when applied to nominal or smoothly corrupted data [1]. Exact calculation of the K L1-norm Principal Components (L1-PCs) of a rank-r datamatrix X ∈ℝ
D×N
costs O(N
(r-1)K+1
) [1], [2]. In this work, we present reduced-rank L1-PCA (RR L1-PCA): a hybrid approach that approximates the K L1-PCs of X by the L1-PCs of its L2-norm-based rank-d approximation (d ≤ r), calculable exactly with reduced complexity O(N
(d-1)K+1
). The proposed method combines the denoising capabilities and low computation cost of standard PCA with the outlier-resistance of L1-PCA. RR L1-PCA is accompanied by formal performance guarantees as well as thorough numerical studies that corroborate its computational and corruption resistance merits.
更多查看译文
关键词
Faulty data,L1-norm,matrix analysis,PCA,outliers
AI 理解论文
溯源树
样例
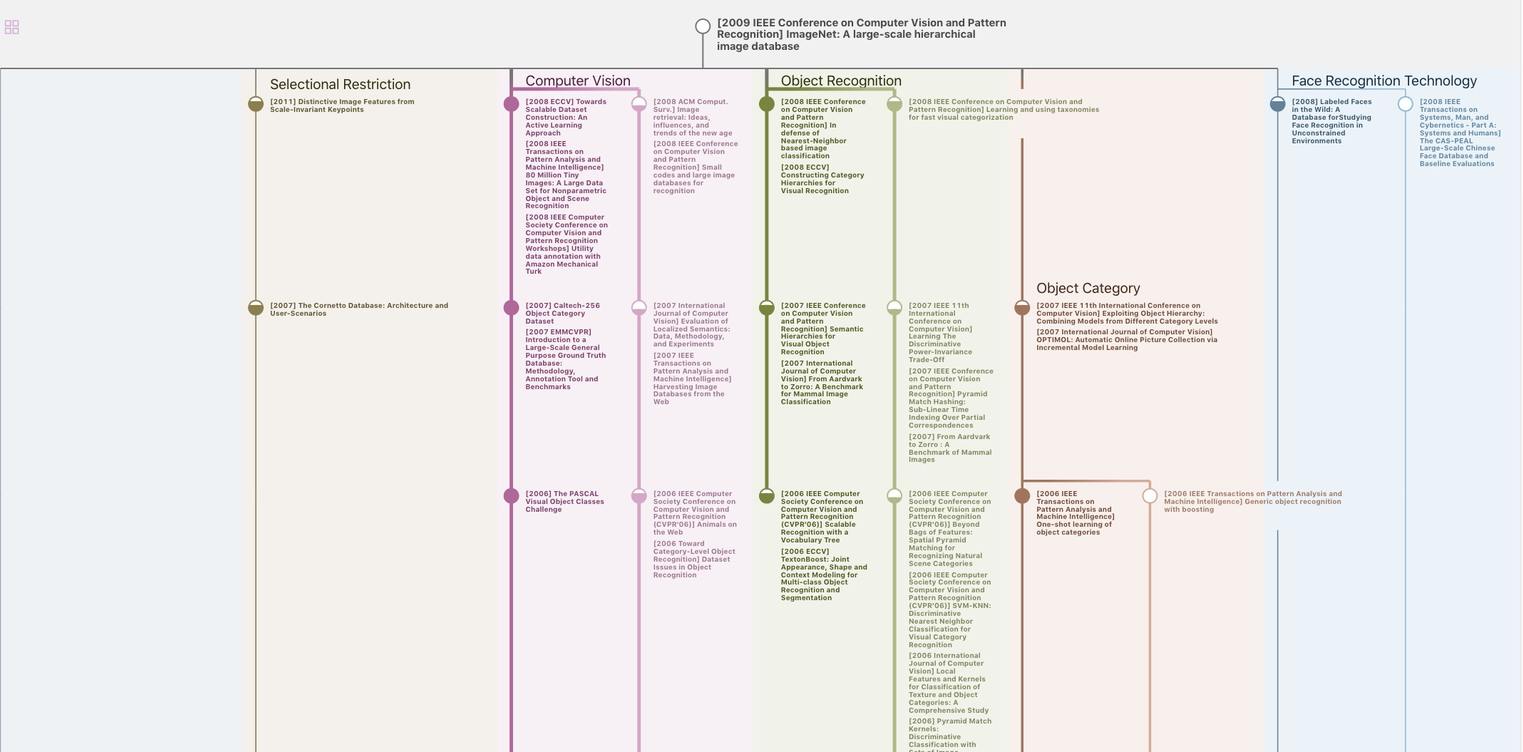
生成溯源树,研究论文发展脉络
Chat Paper
正在生成论文摘要