Reconstructing Human Body Mesh from Point Clouds by Adversarial GP Network.
ACCV (1)(2020)
摘要
We study the problem of reconstructing the template-aligned mesh for human body estimation from unstructured point cloud data. Recently proposed approaches for shape matching that rely on Deep Neural Networks (DNNs) achieve state-of-the-art results with generic point-wise architectures; but in doing so, they exploit much weaker human body shape and surface priors with respect to methods that explicitly model the body surface with 3D templates. We investigate the impact of adding back such stronger shape priors by proposing a novel dedicated human template matching process, which relies on a point-based, deep autoencoder architecture. We encode surface smoothness and shape coherence with a specialized Gaussian Process layer. Furthermore, we enforce global consistency and improve the generalization capabilities of the model by introducing an adversarial training phase. The choice of these elements is grounded on an extensive analysis of DNNs failure modes in widely used datasets like SURREAL and FAUST. We validate and evaluate the impact of our novel components on these datasets, showing a quantitative improvement over state-of-the-art DNN-based methods, and qualitatively better results.
更多查看译文
AI 理解论文
溯源树
样例
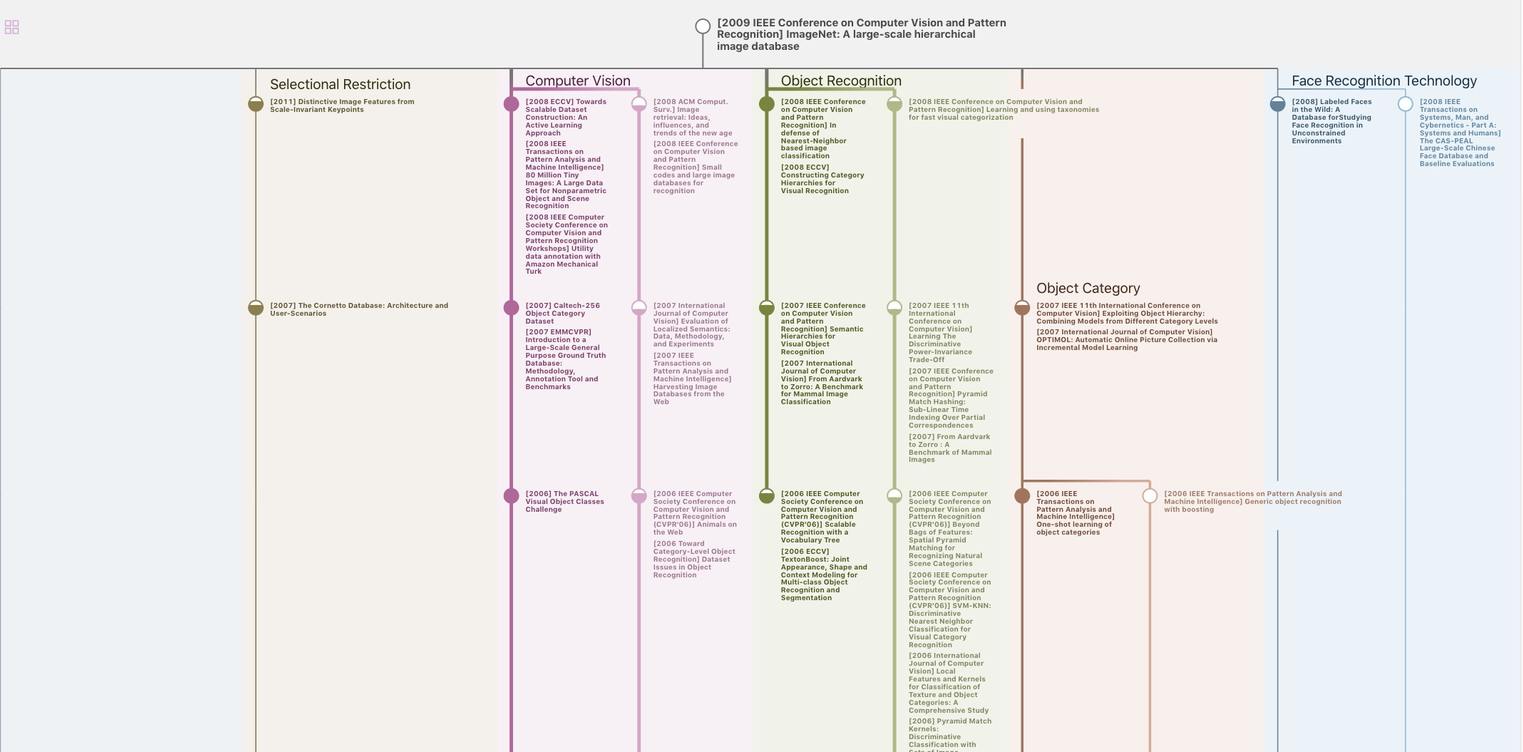
生成溯源树,研究论文发展脉络
Chat Paper
正在生成论文摘要