Depth Estimation from Single Hazy Images with 2-Phase Training
2020 International Conference on Advanced Computer Science and Information Systems (ICACSIS)(2020)
Abstract
Depth estimation is essential for 3D scene understanding of images. Although this task comes naturally for human observers, it is still a challenge for computer vision. In the past, the stereo approach to depth estimation has been well-studied. Nevertheless, the same cannot be said for the monocular approach. Depth estimation from a single image alone is very ambiguous, making it a very ill-posed problem. In recent years, the usage of deep learning approaches has been explored to model the relation between single images and their 3D distance. Due to this problem's complexity, most of these approaches require multiple networks and additional computations to obtain a depth estimate. Furthermore, most depth estimation approaches utilize geometric features. However, in certain conditions the captured images will contain scattering effects. This occurs in images captured in bad weather, fog, or underwater, among others. These images will exhibit low contrast, loss of detail, occlusion issues as well as additive noise. Other works commonly attempt to remove these effects prior to further processing, while we intend to exploit them instead for additional 3D information. This research attempts to learn the relationship between a single hazy image and its depth map using deep networks. We propose a 2-phase training approach for depth estimation from single hazy images, taking advantage of two well-known deep learning architectures, e.g., UNet and Generative Adversarial Network (GAN).
MoreTranslated text
Key words
depth estimation approaches,2-phase training approach,single hazy imaging,computer vision,stereo approach,monocular approach,deep learning approaches,occlusion,additive noise,GAN,generative adversarial network,UNet
AI Read Science
Must-Reading Tree
Example
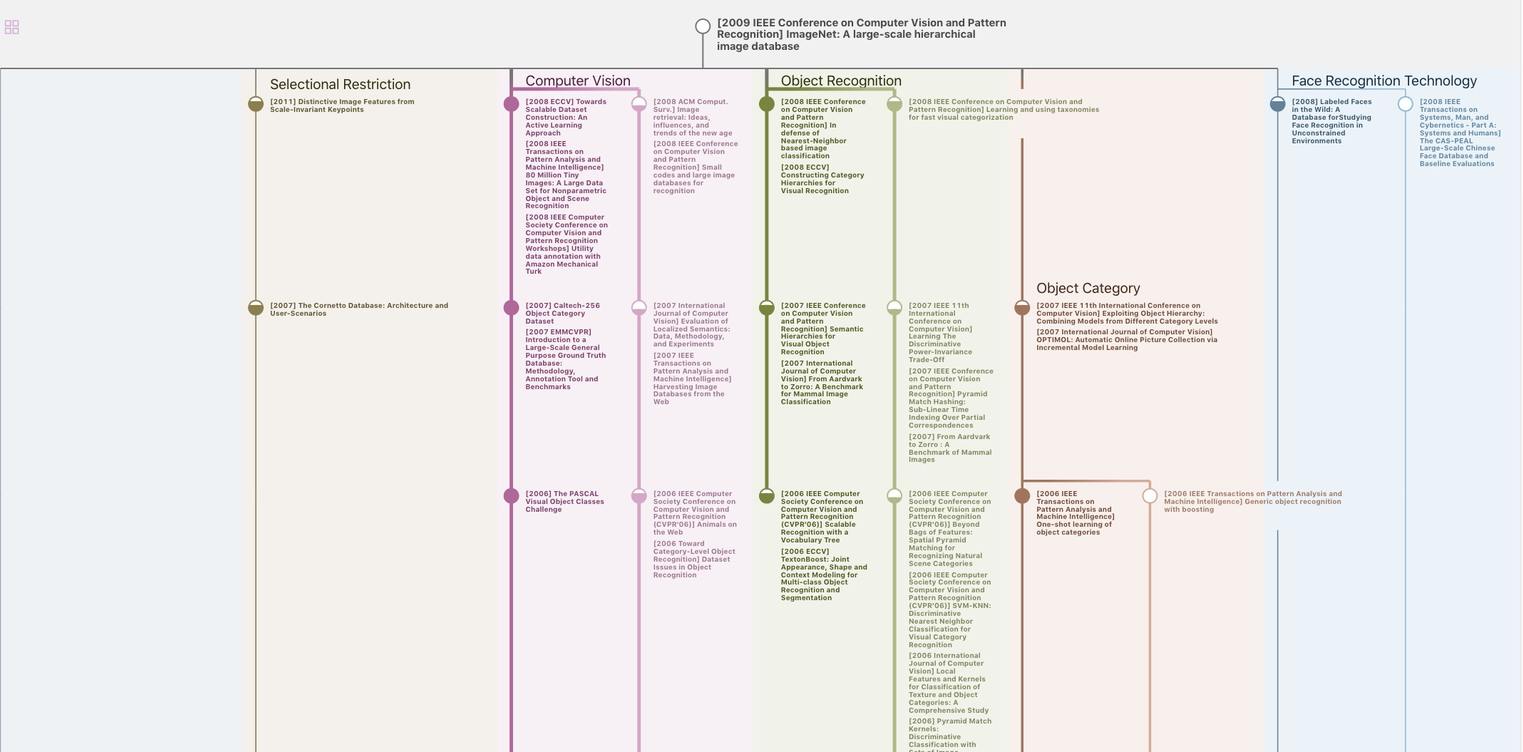
Generate MRT to find the research sequence of this paper
Chat Paper
Summary is being generated by the instructions you defined