Generalization performance of Lagrangian support vector machine based on Markov sampling
Journal of Statistical Planning and Inference(2021)
摘要
In this paper, we first establish the generalization bounds of Lagrangian Support Vector Machines (LSVM) based on uniformly ergodic Markov chain (u.e.M.c.) samples. As an application, we also obtain the generalization bounds of LSVM based on strongly mixing sequence, independent and identically distributed (i.i.d.) samples, respectively. The fast learning rates of LSVM for u.e.M.c., strongly mixing sequence and i.i.d. samples are established. We also propose a new LSVM algorithm based on Markov sampling (LSVM̲MS) and show the learning performance of LSVM̲MS for UCI datasets. The experimental results show that the LSVM̲MS can improve obviously the learning performance of the classical LSVM algorithm. If the sampling and training total time is a main concern, the LSVM̲MS algorithm is the preferred method compared the known SVM algorithm based on Markov sampling.
更多查看译文
关键词
Lagrangian support vector machines (LSVM),Markov sampling,Strongly mixing,i.i.d.,Generalization bound
AI 理解论文
溯源树
样例
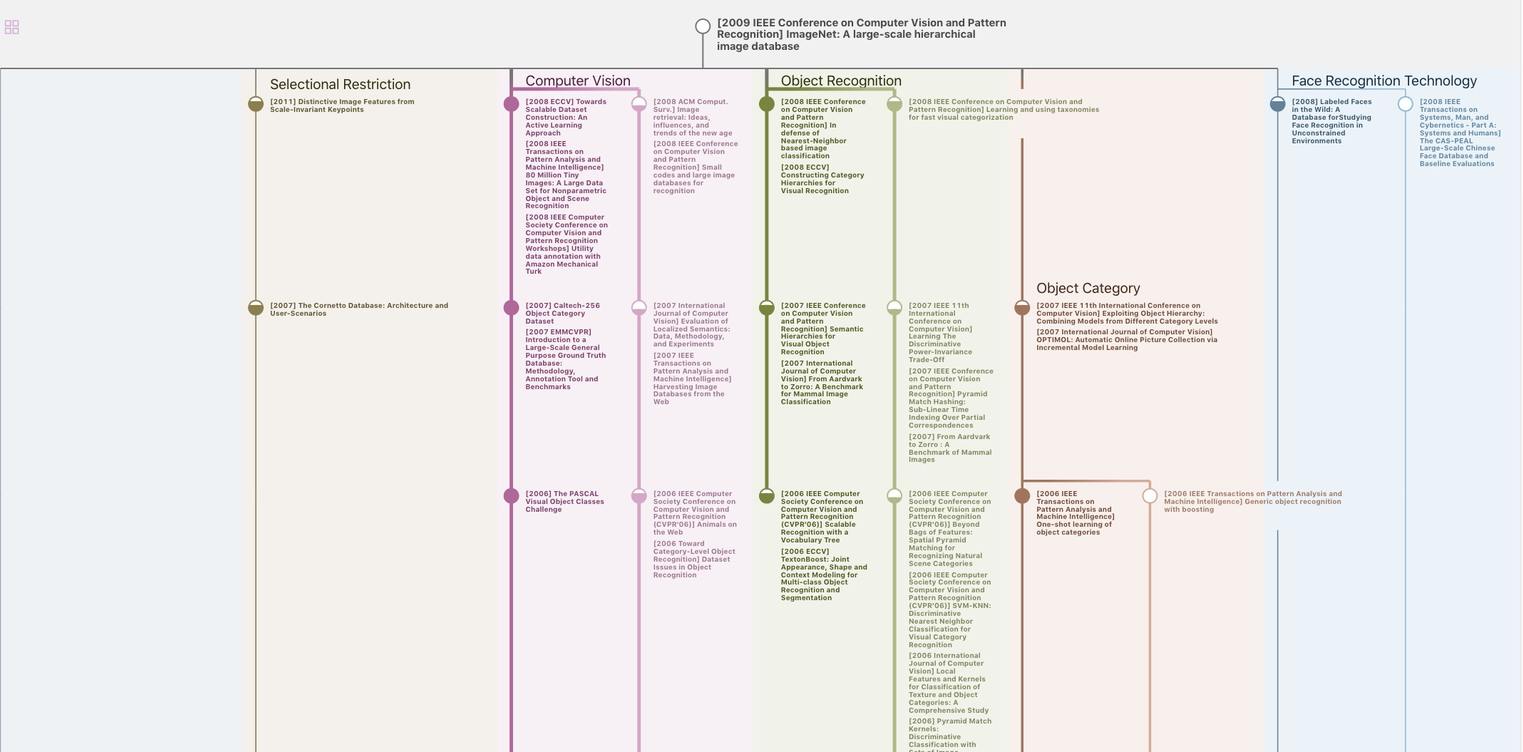
生成溯源树,研究论文发展脉络
Chat Paper
正在生成论文摘要