Automatic Modulation Classification Based On Hierarchical Recurrent Neural Networks With Grouped Auxiliary Memory
IEEE ACCESS(2020)
摘要
As a valuable topic in wireless communication systems, automatic modulation classification has been studied for many years. In recent years, recurrent neural networks (RNNs), such as long short-term memory (LSTM), have been used in this area and have achieved good results. However, these models often suffer from the vanishing gradient problem when the temporal depth and spatial depth increases, which diminishes the ability to latch long-term memories. In this paper, we propose a new hierarchical RNN architecture with grouped auxiliary memory to better capture long-term dependencies. The proposed model is compared with LSTM and gated recurrent unit (GRU) on the RadioML 2016.10a dataset, which is widely used as a benchmark in modulation classification. The results show that the proposed network yields a higher average classification accuracy under varying signal-to-noise ratio (SNR) conditions ranging from 0 dB to 20 dB, even with much fewer parameters. The performance superiority is also confirmed using a dataset with variable lengths of signals.
更多查看译文
关键词
Modulation,Feature extraction,Signal to noise ratio,Recurrent neural networks,Training,Standards,Biological neural networks,Automatic modulation classification (AMC),recurrent neural networks (RNNs),hierarchical recurrent structure,long-term memory
AI 理解论文
溯源树
样例
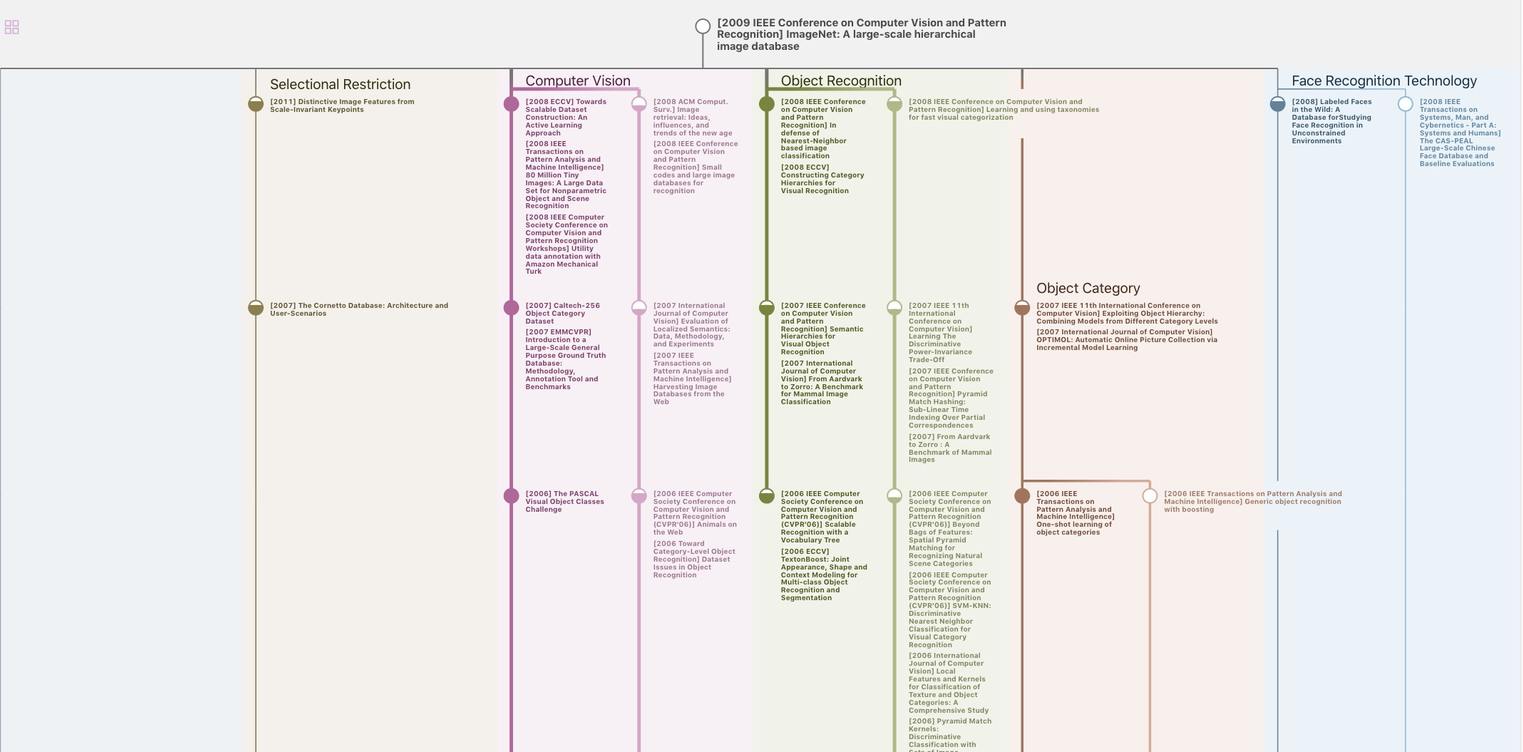
生成溯源树,研究论文发展脉络
Chat Paper
正在生成论文摘要