PoseFromGraph: Compact 3-D Pose Estimation using Graphs
SIGGRAPH(2020)
摘要
ABSTRACT With the rising need for reliable and real-time pose estimation in resource constrained environments such as smartphones, IoT devices, and head mounts, we need an efficient and compact pose estimation framework. To this end, we propose PoseFromGraph1, a light-weight 3D pose estimation framework. The inputs to PoseFromGraph are: a graph obtained by skeletonizing the 3D meshes using the prairie-fire analogy and the RGB image, and the output is the 3D pose of the object. The introduction of 3D shapes to the architecture makes our model category-agnostic. Unlike computationally expensive multi-view geometry and point-cloud based representations to estimate pose, our approach uses a message passing network to incorporate local neighborhood information at the same time maintaining global shape property in a graph by optimizing a neighborhood preserving objective. PoseFromGraph surpasses the state-of-the-art pose estimation methods in terms of accuracy achieving, 84.43% on the Pascal3D dataset, and at the same time yields 4 × reduction in the space and time complexity. The compact pose estimation models can then be used to facilitate on-device inference in applications in Augmented Reality and Robotics for 3D virtual model overlay.
更多查看译文
AI 理解论文
溯源树
样例
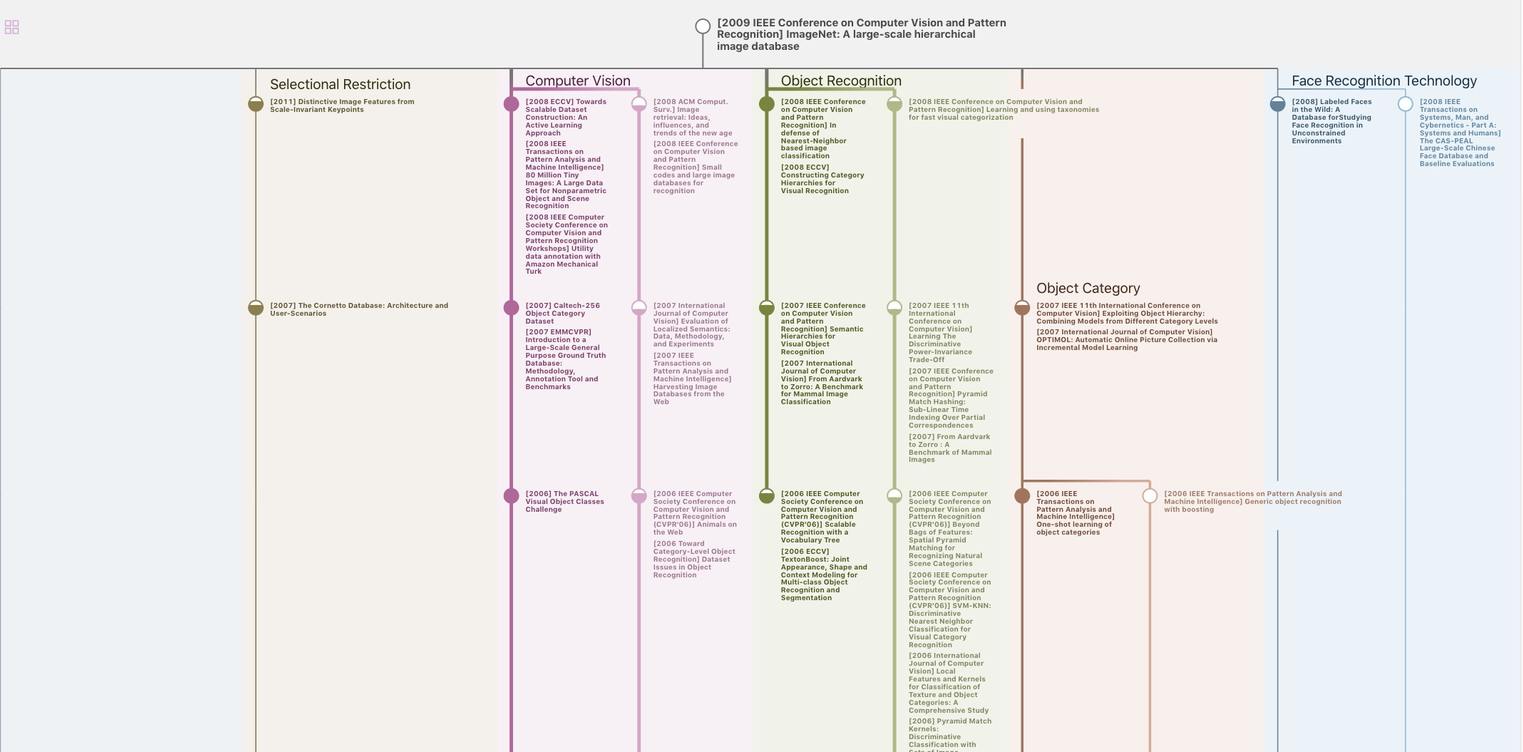
生成溯源树,研究论文发展脉络
Chat Paper
正在生成论文摘要