Evaluating Annual Spruce Budworm Defoliation Using Change Detection Of Vegetation Indices Calculated From Satellite Hyperspectral Imagery
REMOTE SENSING OF ENVIRONMENT(2021)
摘要
During spruce budworm (SBW; Choristoneura fumiferana (Clem.)) outbreaks, defoliation causes severe tree damage and mortality to spruce-balsam fir (Picea spp.-Abies balsamea (L.) Mill.) forests. Annual defoliation thematic maps provide vital information for forest protection planning against outbreaks, but such data are challenging to accurately collect over large areas during a short (2-3 week) period when damaged foliage peaks. Current operational methods for estimating annual defoliation are aerial surveys, through visual observations by professionals from small aircraft, defining broad defoliation classes which are subjective and spatially can be inaccurate. Remote sensing studies estimating annual defoliation are limited due to lack of ground verification data and difficulty of capturing spectral changes specific to the short detection period. Only one study has used satellite hyperspectral imagery to detect annual SBW defoliation, classifying just two classes of defoliation and using inaccurate aerial survey results for training and validation. In this paper, we introduce a new method to estimate annual SBW defoliation in three classes (light <= 30%, moderate 30-70%, and severe >= 70%), using change detection of vegetation indices (VIs) derived from satellite (EO-1 Hyperion) hyperspectral imagery acquired before and after annual defoliation. Random Forest and Support Vector Machine classifiers were used to classify defoliation, and intensive field measurements of branch-level defoliation per plot were used for accuracy assessment. Field plots, with spatially correlated defoliation within stands, were used to derive an additional 624 plots to obtain sufficient and independent datasets for training and validating the detection results. The top four VIs for detecting annual defoliation, based on Random Forest Total Variable Importance Score and Spearman's rank correlations, were Plant Senescence Reflectance Index, Normalized Difference Water Index, Moisture Stress Index, and Enhanced Vegetation Index. The spectral regions of VIs used to classify defoliation included visible, near-infrared, red-edge, and shortwave infrared. Accuracies of the light, moderate, and severe defoliation classes using our method ranged from 55-85%, 40-76%, and 40-80%, respectively, which were significantly higher than the 32% accuracy of aerial survey defoliation data for the plots.
更多查看译文
关键词
Hyperspectral imagery, Spruce budworm, Defoliation, Random forests, Support vector machine, Change detection, Vegetation indices
AI 理解论文
溯源树
样例
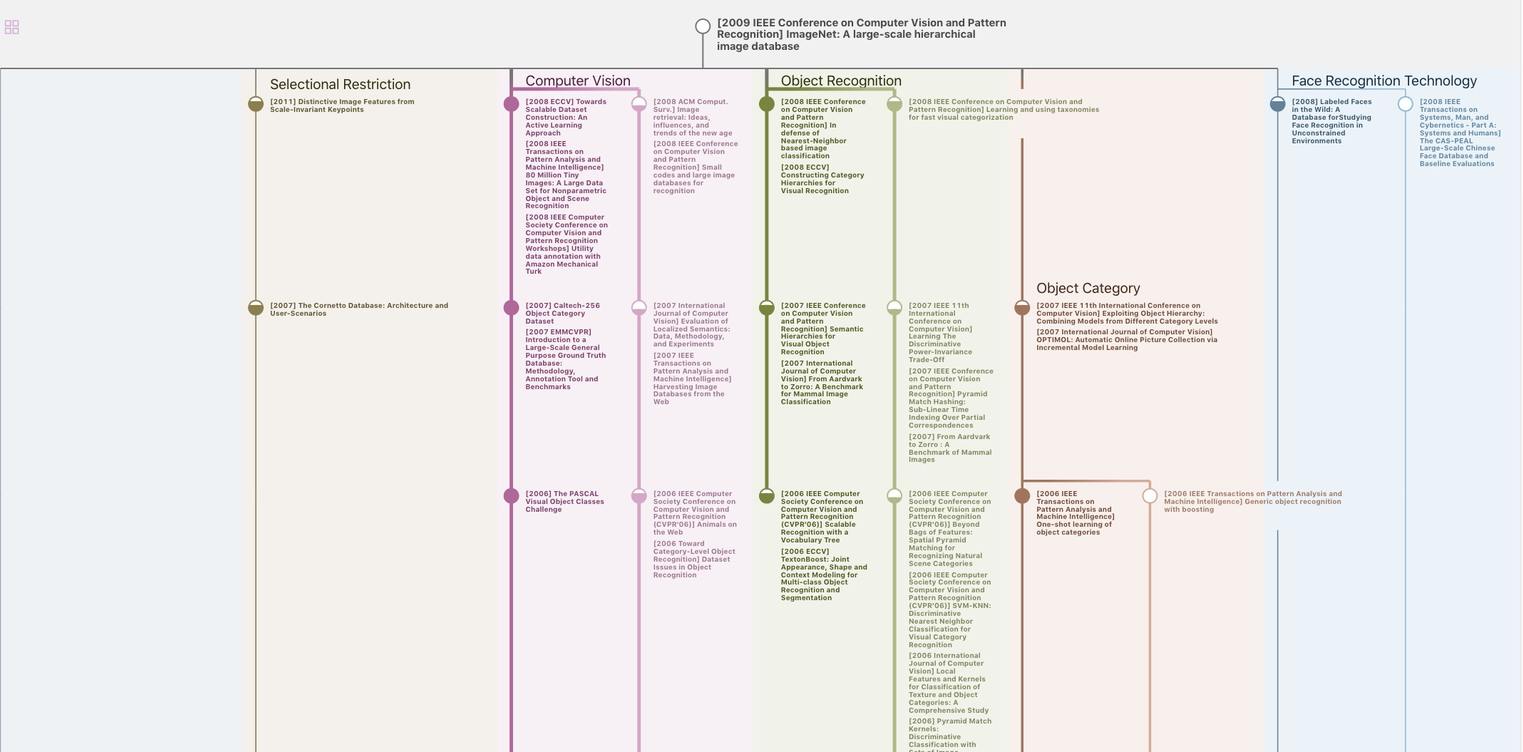
生成溯源树,研究论文发展脉络
Chat Paper
正在生成论文摘要