Building a Predictive Model for Warfarin Dosing Via Machine Learning
European heart journal(2020)
Abstract
Abstract Background Warfarin titration via International Normalised Ratio (INR) monitoring can be a challenge for patients on long term anticoagulation due to its multiple drug interactions, long half life and patient's individual reponse, yet critically important due to its narrow therapeutic index. Machine learning may have a role in learning and replicating existing warfarin prescribing practices, to be potentially incorporated into an automated warfarin titration model. Purpose We aim to explore the feasibility of using machine learning to develop a model that can learn and predict actual warfarin titration practices. Methods A retrospective dataset of 4,247 patients with 48,895 data points of INR values were obtained from our institutional database. Patients who had less than 5 visits recorded, invalid or missing values were excluded. Variables studied included age, warfarin indication, warfarin dose, target INR range, actual INR values, time between titration and time in therapeutic range (TTR as defined by the Rosenthal formula). The machine learning model was developed on an unbiased training data set (1,805 patients), further refined on a handpicked balanced validation set (400 patients), before being evaluated on two balanced test sets of 100 patients each. The test sets were handpicked based on the criteria of TTR (“in vs out of range”) and stability of INR results (“low vs high fluctuation”) (Table 1). Given the time series nature of the data, a Recurrent Neural Network (RNN) was chosen to learn warfarin prescription practices. Long-short term memory (LSTM) cells were further employed to address the problem of time gaps between warfarin titration visits which could result in vanishing gradients. Results A total of 2,163 patients with 42,622 data points were studied (mean age 65±11.7 years, 54.7% male). The mean TTR was 65.4%. The total warfarin dose per week as predicted by the RNN was compared with actual total warfarin dose per week prescribed for each patient in the test sets. The coefficient of determination for the RNN in the “in vs out of range” and “low vs high fluctuation” test sets were 0.941 and 0.962 respectively (Figure 1). Conclusion This proof of concept study demonstrated that a RNN based machine learning model was able to learn and predict warfarin dosage changes with reasonable accuracy. The findings merit further evaluation of the potential use of machine learning in an automated warfarin titration model. Funding Acknowledgement Type of funding source: None
MoreTranslated text
AI Read Science
Must-Reading Tree
Example
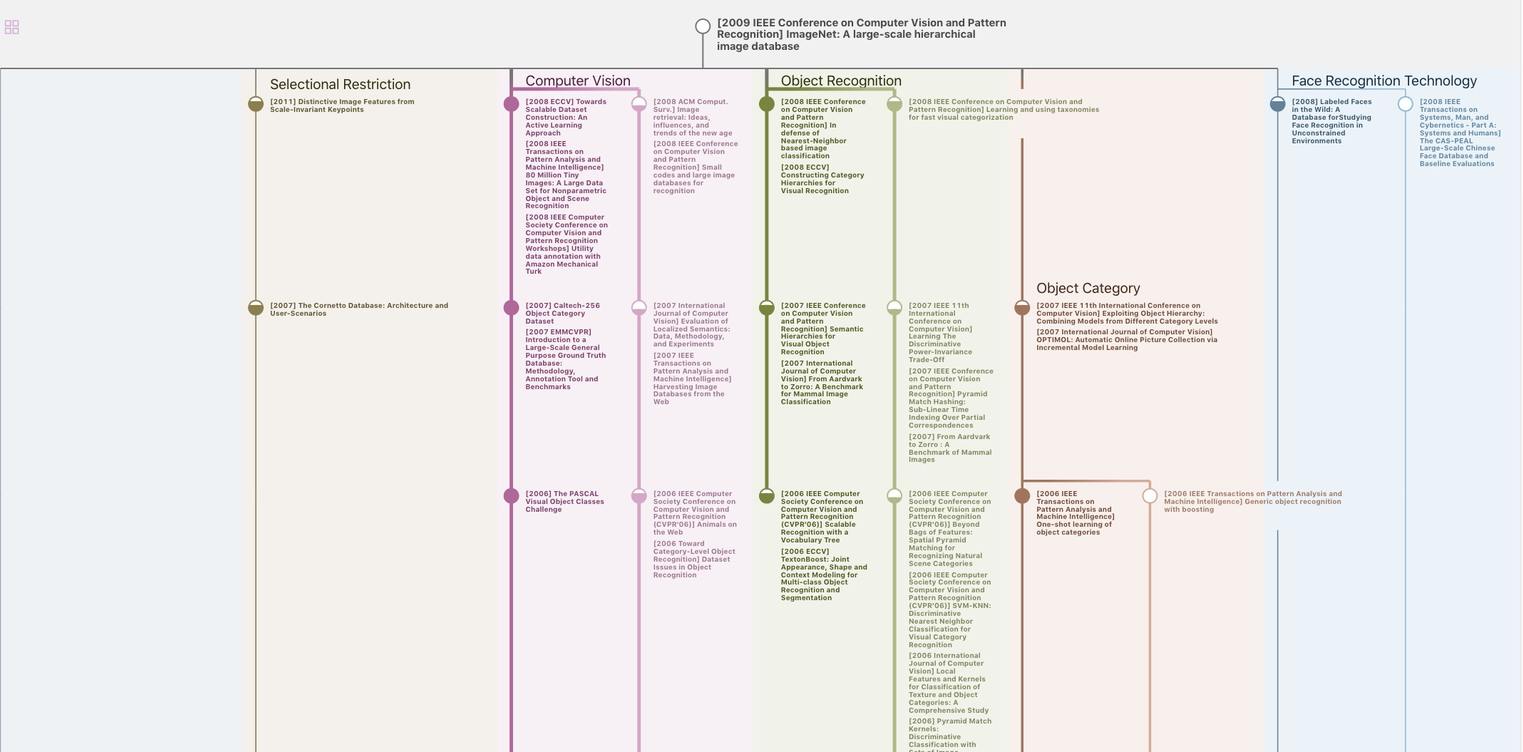
Generate MRT to find the research sequence of this paper
Chat Paper
Summary is being generated by the instructions you defined