Privacy-Preserving Computation Offloading for Parallel Deep Neural Networks Training.
IEEE Trans. Parallel Distributed Syst.(2021)
摘要
Deep neural networks (DNNs) have brought significant performance improvements to various real-life applications. However, a DNN training task commonly requires intensive computing resources and a huge data collection, which makes it hard for personal devices to carry out the entire training, especially for mobile devices. The federated learning concept has eased this situation. However, it is still an open problem for individuals to train their own DNN models at an affordable price. In this article, we propose an alternative DNN training strategy for resource-limited users. With the help of an untrusted server, end users can offload their DNN training tasks to the server in a privacy-preserving manner. To this end, we study the possibility of the separation of a DNN. Then we design a differentially private activation algorithm for end users to ensure the privacy of the offloading after model separation. Furthermore, to meet the rising demand for federated learning, we extend the offloading solution to parallel DNN models training with a secure model weights aggregation scheme for the privacy concern. Experimental results prove the feasibility of computation offloading solutions for DNN models in both solo and parallel modes.
更多查看译文
关键词
Deep neural network,federated learning,computation offloading,data privacy,model parallelism
AI 理解论文
溯源树
样例
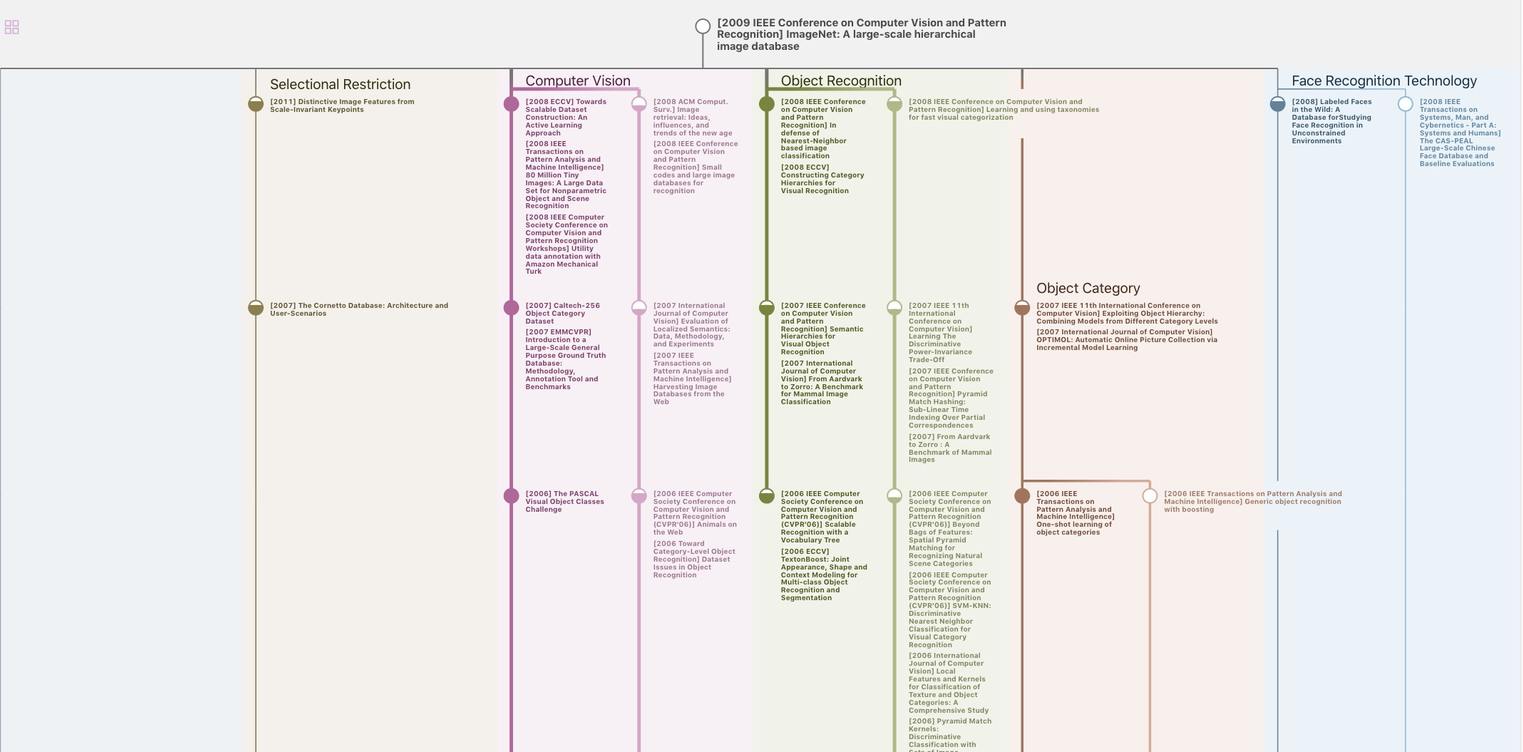
生成溯源树,研究论文发展脉络
Chat Paper
正在生成论文摘要