An Ordered Search With A Large Margin Classifier For Feature Selection
APPLIED SOFT COMPUTING(2021)
摘要
In this paper we propose a novel feature selection method for binary classification problems based on an ordered search process to explore the space of feature subsets. The method, called Admissible Ordered Search (AOS), uses a monotone evaluation function based on margin values calculated by a large margin classifier with an arbitrary L-p norm. This includes large margin classifiers with the L-infinity norm, which minimize the L-1 norm and are very useful in feature selection, since they produce sparse solutions. An important contribution of this paper is the development of the projected margin concept. This value is computed as the maximal margin vector projected into a lower-dimensional subspace and it is used as an upper bound for the hypothesis value. This enables great economy in runtime and consequently efficiency in the search process as a whole. AOS was tested on several problems and its results were compared to other feature selection methods. The experiments demonstrate the competitive performance of the proposed method in terms of generalization power and computational efficiency.(c) 2020 Elsevier B.V. All rights reserved.
更多查看译文
关键词
Feature selection, Ordered search, Large margin classifier, Projected margin, Wrapper method
AI 理解论文
溯源树
样例
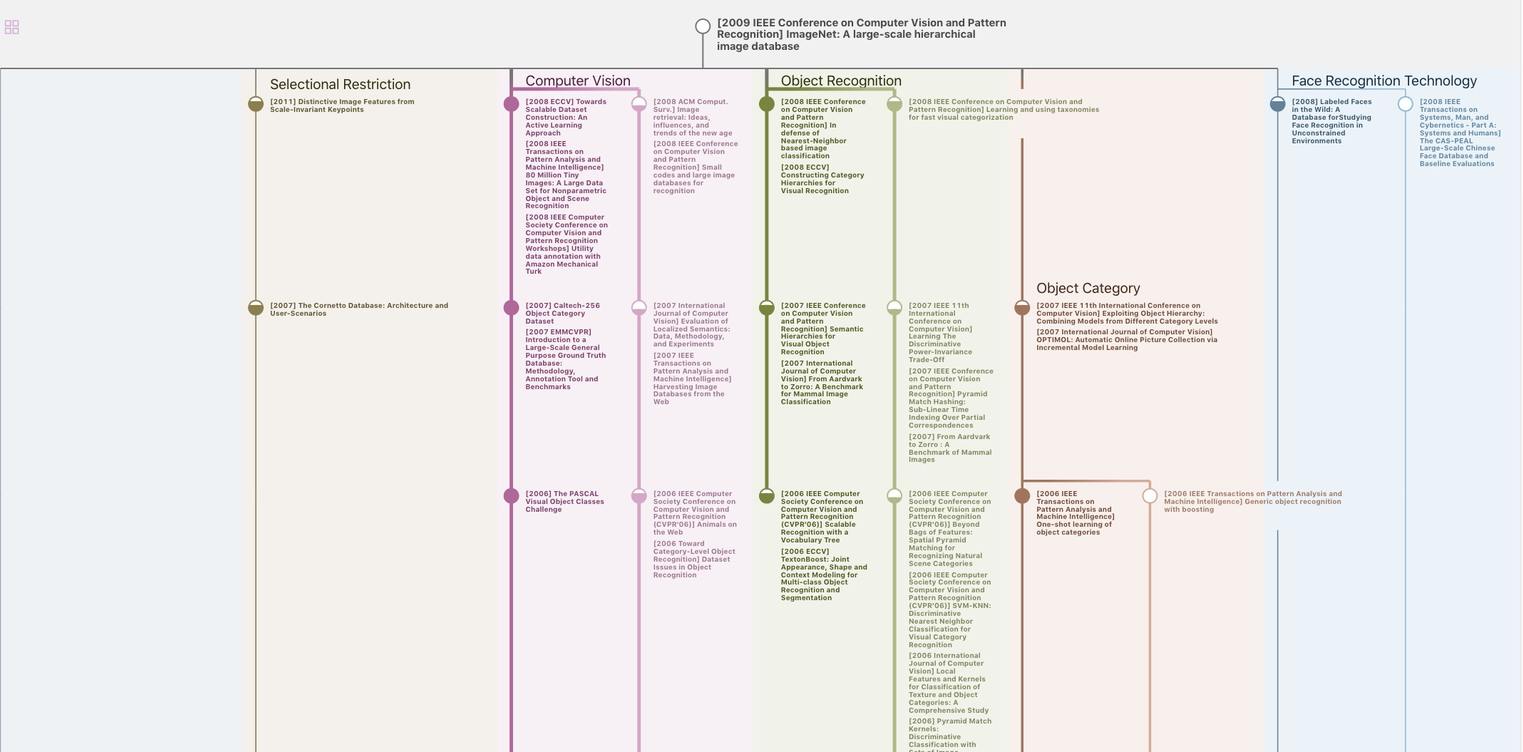
生成溯源树,研究论文发展脉络
Chat Paper
正在生成论文摘要