Traffic Flow Prediction By An Ensemble Framework With Data Denoising And Deep Learning Model
PHYSICA A-STATISTICAL MECHANICS AND ITS APPLICATIONS(2021)
摘要
Accurate traffic flow data is important for traffic flow state estimation, real-time traffic management and control, etc. Raw traffic flow data collected from inductive detectors may be contaminated by different noises (e.g., sharp data increase/decrease, trivial anomaly oscillations) under various unexpected interference (caused by roadway maintenance, loop detector damage, etc.). To address the issue, we introduced data denoising schemes (i.e., Empirical Mode Decomposition (EMD), Ensemble Empirical Mode Decomposition (EEMD) and Wavelet (WL)) to suppress the potential data outliers. After that, the Long Short-Term Memory (LSTM) neural network was introduced to fulfill the traffic flow prediction task. We have tested the proposed framework performance on three traffic flow datasets, which were downloaded from Caltrans Performance Measurement System (PeMS). The experimental results showed that the LSTM+EEMD scheme obtained higher accuracy considering that the average Root Mean Square Error (RMSE), Mean Absolute Error (MAE) and Mean Absolute Percentage Error (MAPE) are 0.79, 0.60 and 2.14. (C) 2020 Published by Elsevier B.V.
更多查看译文
关键词
Traffic flow prediction, Ensemble framework, Data quality control, LSTM network
AI 理解论文
溯源树
样例
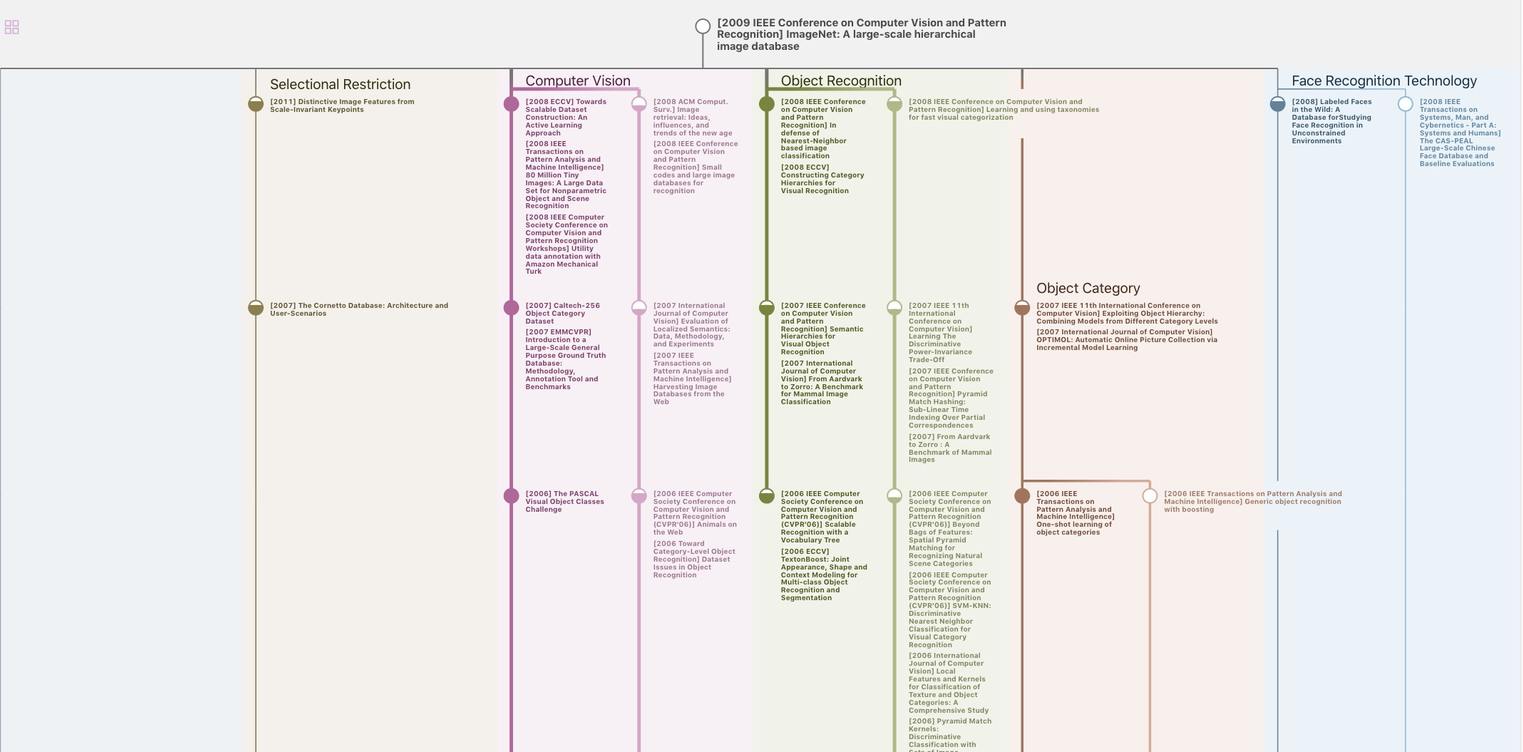
生成溯源树,研究论文发展脉络
Chat Paper
正在生成论文摘要