Identifying Spatiotemporal Clusters by Means of Agglomerative Hierarchical Clustering and Bayesian Regression Analysis with Spatiotemporally Varying Coefficients: Methodology and Application to Dengue Disease in Bandung, Indonesia
Geographical Analysis(2020)
AI 理解论文
溯源树
样例
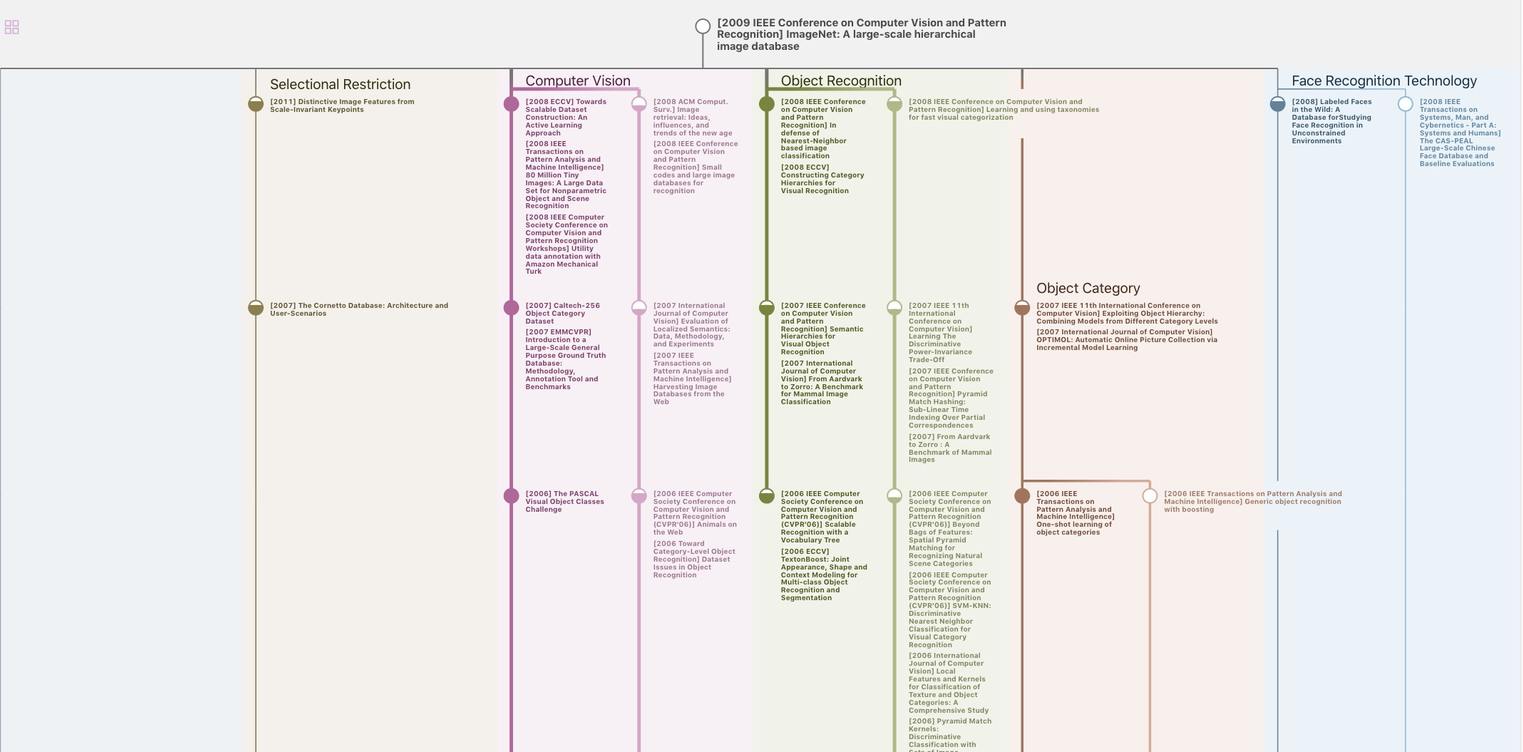
生成溯源树,研究论文发展脉络
Chat Paper
正在生成论文摘要