Extrapolation of drug induced liver injury responses from cancer cell lines using machine learning approaches
Computational Toxicology(2021)
Abstract
Currently, computational tools able to predict drug-induced liver injury (DILI) based on gene expression data from in vitro assays remain a challenge. Here we introduce a Qualitative Gene expression Activity Relationship (QGexAR) approach to assess the risk of DILI for any chemical. Using gene expression profiles of 276 chemicals tested on breast cancer cell line (MCF7) and prostate cancer cell line (PC3), we developed classification models based on Support Vector Machine (SVM) and Random Forest (RF) aiming at predicting the risk of DILI. With the combination of tissue expression filtering and a pathway enrichment fingerprint approach, a classification model with an accuracy reaching 0.72 in the training set and 0.95 in a validation set was obtained. Genes and pathways involved in liver metabolism contributed the most to the performance of the models. Notably, FoxO signalling pathway, alanine, aspartate and glutamate metabolism, drug metabolism and cytochrome P450 are highlighted, giving some indication to the mechanisms of action leading to DILI.
MoreTranslated text
Key words
Toxicogenomics,Chemical risk assessment,Drug-induced-liver injury,QGexAR,Support Vector Machine,Liver toxicity
AI Read Science
Must-Reading Tree
Example
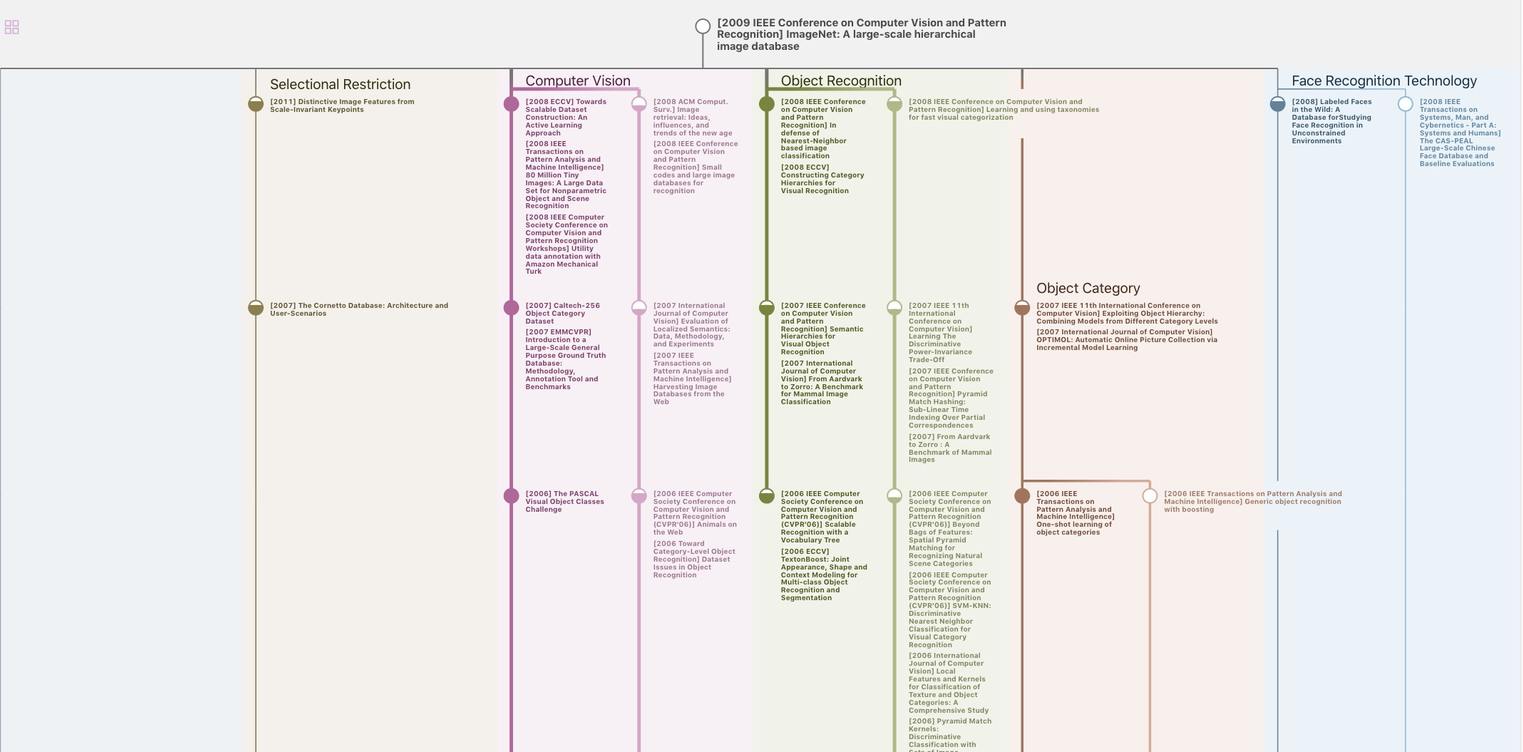
Generate MRT to find the research sequence of this paper
Chat Paper
Summary is being generated by the instructions you defined