Computationally Efficient Approximations For Independence Tests In Non-Parametric Regression
JOURNAL OF STATISTICAL COMPUTATION AND SIMULATION(2021)
摘要
A common assumption in non-parametric regression models is the independence of the covariate and the error. Some procedures have been suggested for testing that hypothesis. This paper considers a test, whose test statistic compares estimators of the joint and the product of the marginal characteristic functions of the covariate and the error. It is proposed to approximate the null distribution of such statistic by means of a weighted bootstrap estimator. The resulting test is able to detect any fixed alternative as well as local alternatives converging to the null at the rate n(-1/2), n denoting the sample size. The finite sample performance of this approximation is assessed by means of a simulation study, where it is also compared with other estimators. This study reveals that, from a computational point of view, the proposed approximation is very efficient. Two real data set applications are also included.
更多查看译文
关键词
Testing for independence, characteristic function, non-parametric regression models, weighted bootstrap, consistency, computational efficiency
AI 理解论文
溯源树
样例
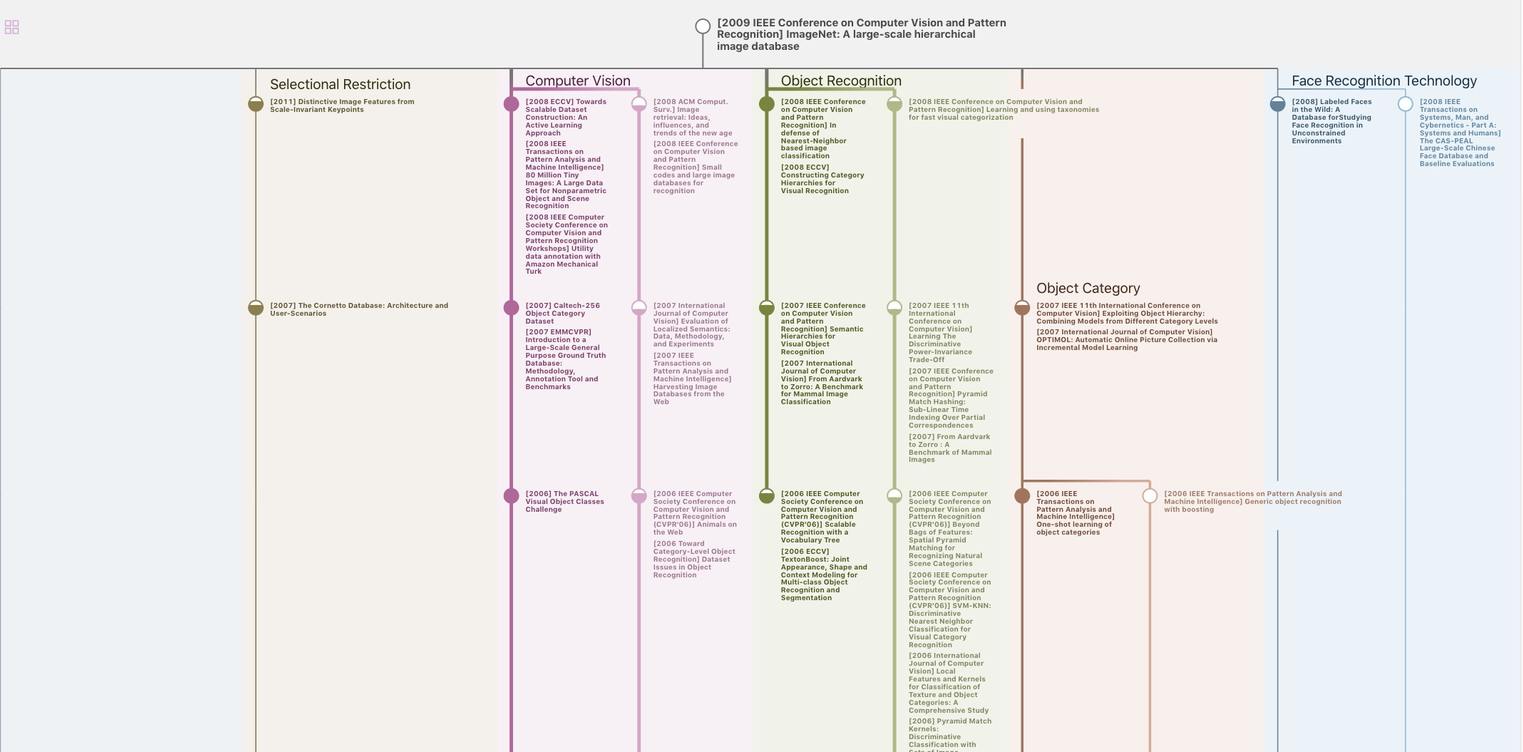
生成溯源树,研究论文发展脉络
Chat Paper
正在生成论文摘要