Prediction of CO2 and ethylene produced in‐packaged apricot under cold plasma treatment by machine learning approach
Journal of Food Process Engineering(2023)
摘要
Abstract In this study, the fresh and in‐packaged apricots were treated with dielectric barrier discharge (DBD) cold plasma for 5, 10, and 15 min and then stored at 21°C for 12 days to simulate the shelf life of the apricot. The effects of DBD treatment on the main quality attributes of apricot such as physicochemical traits (mass loss, pH, soluble solid content, titratable acidity, and skin color), mechanical properties (Young's modulus, tangent modulus, and bioyield stress), in‐package gas composition and ethylene production were investigated during the storage time. In addition, the bruise susceptibility (BS) of control and treated samples at the microscale was evaluated by using pendulum test and scanning electron microscopy. The results of the mass loss, pH, soluble solid content, titratable acidity, skin color, and bioyield stress have been applied for input parameters of the developed artificial neural network (ANN) and support vector regression (SVR) models to predict the CO 2 and ethylene production. The statistical data showed the performance of the developed ANN to predict the CO 2 ( R 2 = 0.983, root mean square error [RMSE] = 0.486) and ethylene production ( R 2 = 0.933, RMSE = 5.376) was superior to SVR (CO 2 : R 2 = 0.894, RMSE = 6.077 and ethylene: R 2 = 0.759, RMSE = 14.117). These results indicated that intelligent methods are effective and robust tools for predicting the quality parameters of fresh fruits in the postharvest process. Practical Applications Advancement of technology and artificial intelligence leads to the tendency of many food industry engineers and researchers in the R&D department to use this technology to classify and predict various food industry processes. In the process of packaging products, there are challenges such as the amount of produced carbon dioxide and ethylene inside the package, which affects the shelf life of fresh agricultural products. In this research, artificial neural networks and support vector machine methods have been used to predict the two mentioned parameters. This approach can be used for the digitalization of the packaging process of agricultural and horticultural products and reduce the number of experimental activities. In addition, it is possible to improve the digitalization process by the fusion of smart sensors with machine learning methods.
更多查看译文
关键词
apricot,ethylene,cold plasma treatment,machine learning,machine learning approach
AI 理解论文
溯源树
样例
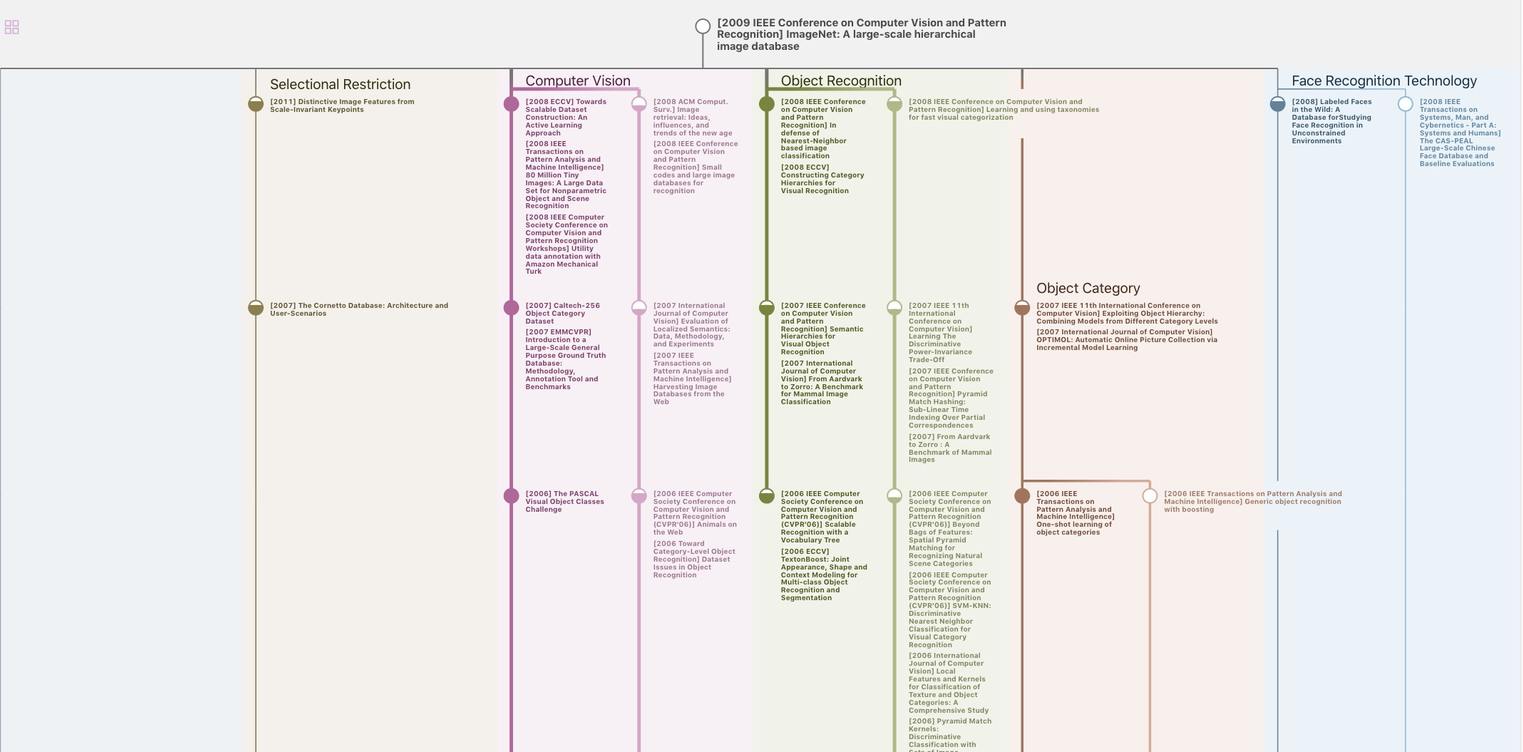
生成溯源树,研究论文发展脉络
Chat Paper
正在生成论文摘要