Gradient as a Foundation for Building a Loss Function
2020 VI INTERNATIONAL CONFERENCE ON INFORMATION TECHNOLOGY AND NANOTECHNOLOGY (IEEE ITNT-2020)(2020)
摘要
Deep neural networks have achieved a tremendous success in a variety of applications across many disciplines. Yet, training a neural network comes with several challenges that have to be solved. The performance of a deep learning models relies not only on the network architecture but also on the choice of a loss function. Cross-entropy loss found to be the most common choice for the classification problem. But its main downside is that it cannot handle data with severe class imbalance. In order to tackle this problem, the focal loss has been proposed. In this paper we investigate the reasons behind its good performance. We find several properties of the focal loss' gradient that can be applied for building new loss functions and propose a few of them. We also show an experimental evidence of the validity of the proposed functions.
更多查看译文
关键词
convolution, neural networks, convolutional network, computer vision, object recognition, class imbalance, focal loss, gradient
AI 理解论文
溯源树
样例
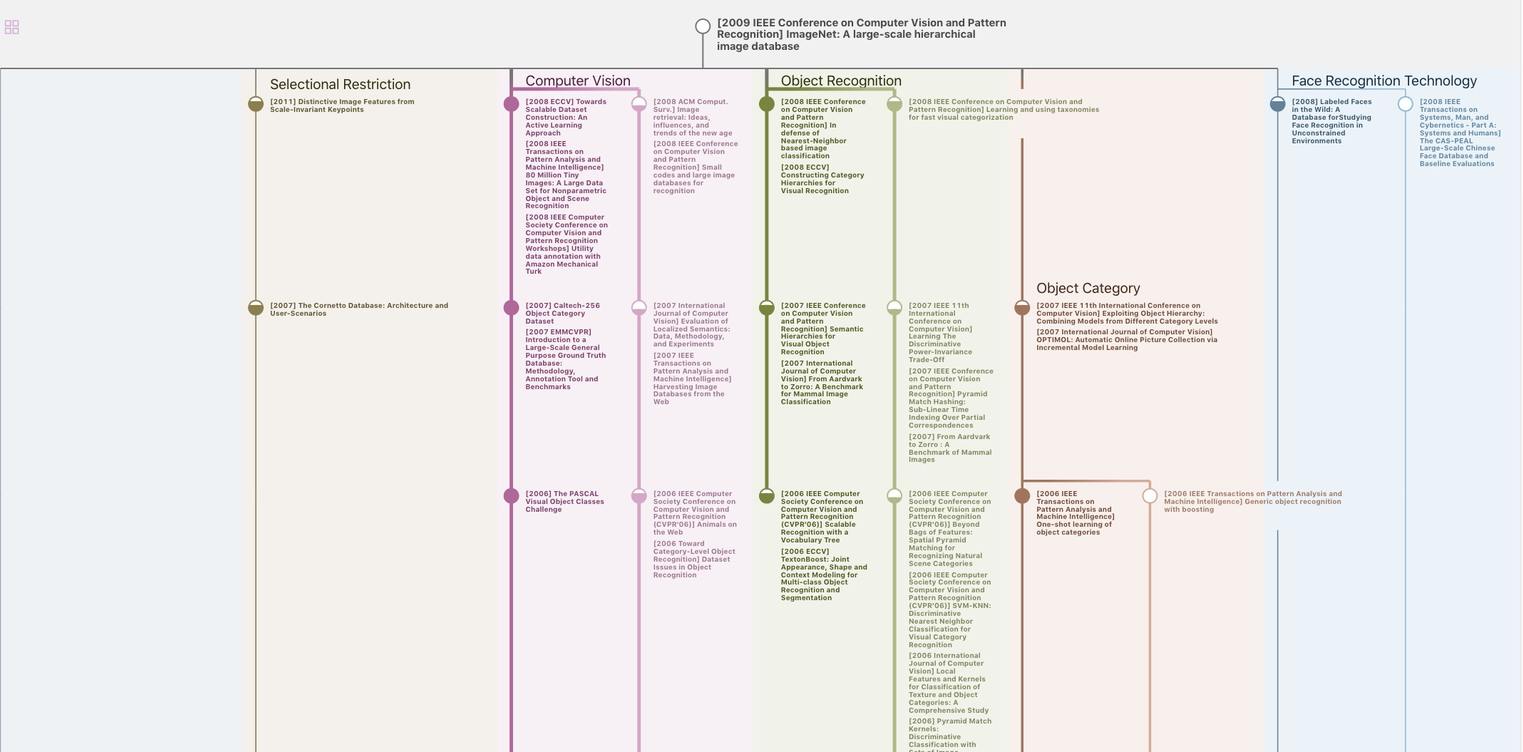
生成溯源树,研究论文发展脉络
Chat Paper
正在生成论文摘要