A Dynamic Filtering Df-Rnn Deep-Learning-Based Approach For Eeg-Based Neurological Disorders Diagnosis
IEEE ACCESS(2020)
摘要
Filtering of unwanted signals has a great impact on the performance of EEG signal processing applied to neurological disorders diagnosis. It is so difficult to remove undesirable noises using static filtering approaches as the performance of such techniques is strongly relying on specific EEG signal sub-bands, whose locations differ from one subject to another. In this paper, we present a novel dynamic filtering approach, which makes use of Finite and Infinite Impulse Response (FIR and IIR) filters along with a Recurrent Neural Networks using a Gated-Recurrent Unit (RNN-GRU), to identify and preprocess the most informative sub-bands pertaining to a particular neurological disorder. This combination of RNN with GRU requires more hidden layers than for conventional NN structures, and therefore offers much higher capacity to learn fitting and extract features from highly complex EEG data recording to afford better harmonization of the diagnosis process. Followed by an Independent Component Analysis (ICA) algorithm, all extracted features become independent to facilitate classification of clinical disorders using Convolutional Neural Network (CNN). The proposed diagnosis system achieves an average of 100% classification accuracy for epilepsy according to an offline diagnosis process using Bonn and MIT datasets, and when the same system is applied to autism provides an average accuracy of 99.5% using KAU dataset. The presented dynamic deep-learning approach applied to EEG classification pipeline, which includes artifact removal, feature extraction and classification, leads to significant improvements in the accuracy of the diagnosis classification regarding the targeted neurological pathologies.
更多查看译文
关键词
Electroencephalography, Feature extraction, Autism, Epilepsy, Neurological diseases, Time-frequency analysis, Medical diagnostic imaging, Electroencephalography (EEG), deep learning, recurrent neural networks (RNN), convolutional neural network (CNN), dynamic filtering
AI 理解论文
溯源树
样例
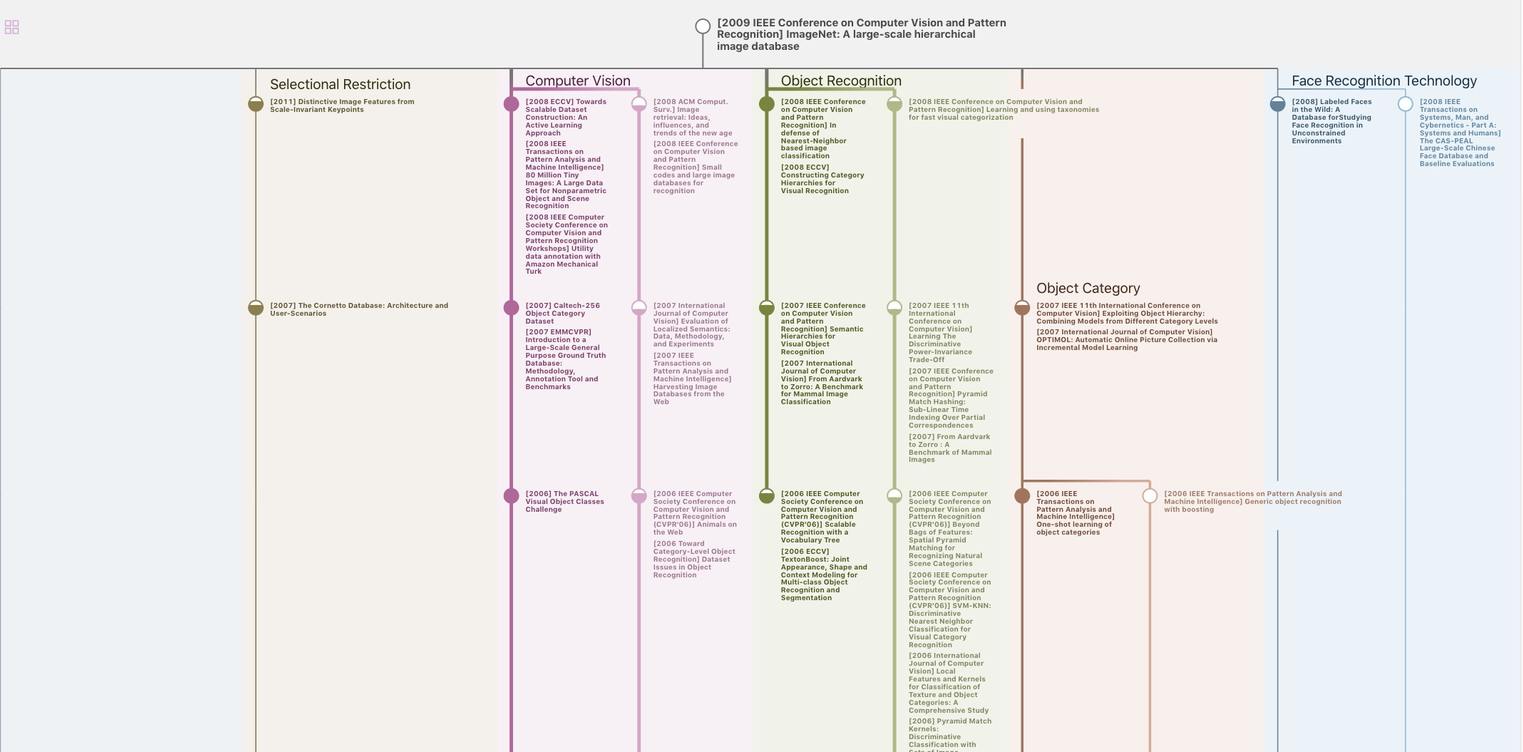
生成溯源树,研究论文发展脉络
Chat Paper
正在生成论文摘要