A Deep Time Series Forecasting Method Integrated with Local-Context Sensitive Features.
ICONIP (3)(2020)
摘要
Time series forecasting predicts values in future timestamps based on historically observed series information. Algorithms based on deep neural networks such as Temporal Convolution Network(TCN) have outperformed traditional methods such as Autoregressive Integrated Moving Average Model(ARIMA). However, most existing deep learning approaches suffer from the insufficient ability to capture the seasonality features in series adequately since the network structure ignores the fact that the importance of points the series varies a lot. The local context that reflects a sub-segment of seasonality can indicate potential patterns of the series based on the periodicity. Therefore, we tend to exploit local information from historical records. To this end, we develop a novel strategy to extract local context sensitivity information and integrate them into the current state-of-the-art TCN model, namely LS-TCN. This information enables an improvement in capturing the series pattern and fluctuation, as well as providing transferable guidance for forecasting in the next steps. Experiments conducted on three different real-world series datasets demonstrate that our method significantly outperforms the state-of-the-art models, especially in autocorrelation series corpus.
更多查看译文
关键词
Time series forecasting, Temporal convolution network, Point context similarity
AI 理解论文
溯源树
样例
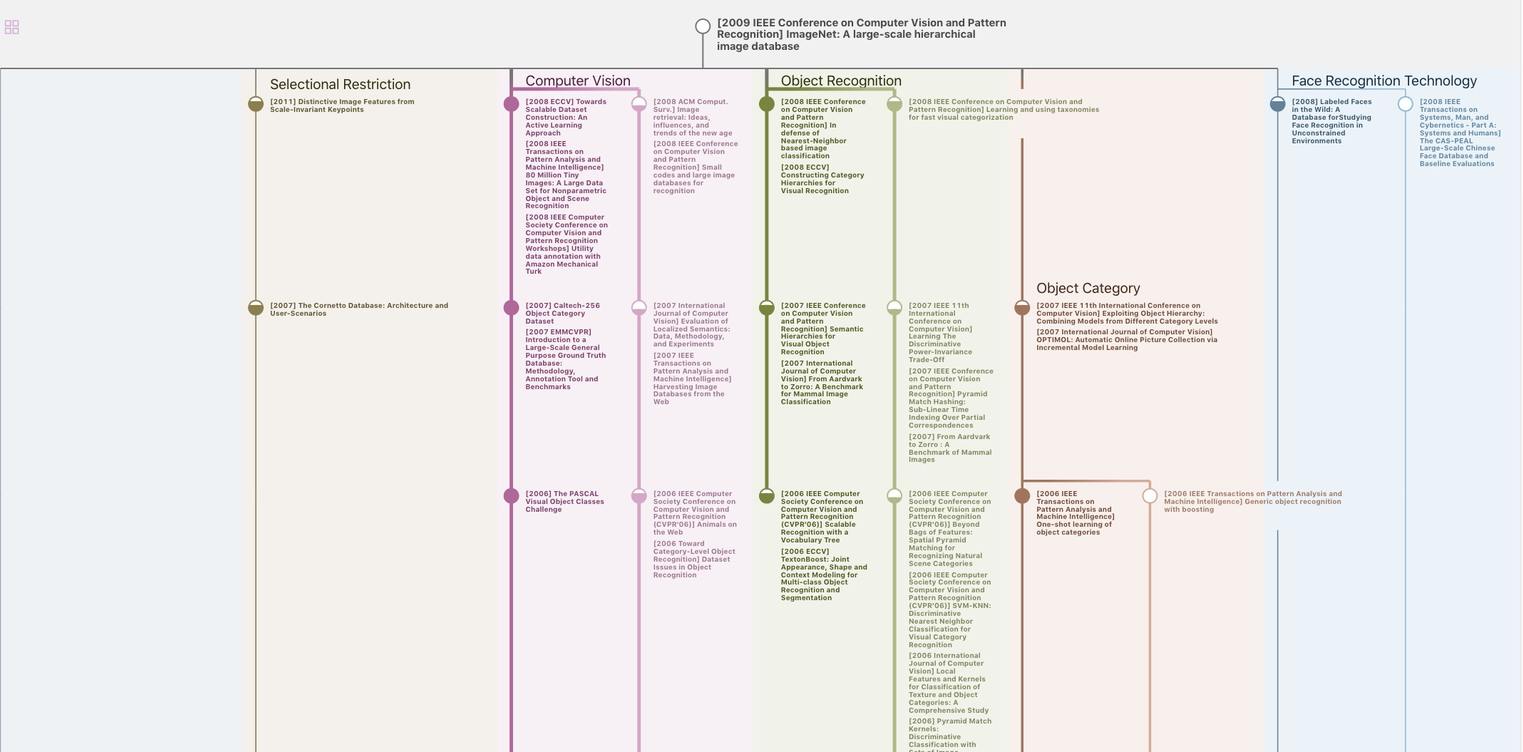
生成溯源树,研究论文发展脉络
Chat Paper
正在生成论文摘要