On the connection between Lagrangian and Eulerian metrics for recurrent particulate flows
PHYSICS OF FLUIDS(2020)
Abstract
Data analysis and recently developed data-assisted simulations of particulate flows often require assessing the similarity of their spatial structure at different times. To that end, various metrics have been defined in the literature, either of Eulerian, field-based or of Lagrangian, particle-position-based nature. We demonstrate the equivalence of a broad class of these distance functions for sufficiently recurrent states analytically and numerically on simulation data of a small-scale, flat fluidized bed consisting of N-p = 50 000 grains. The investigated Eulerian and Lagrangian metrics led to consistent dynamic properties. Both identified a correlation dimension of about D-corr less than or similar to 20, which is orders of magnitude smaller than the number of microscopic degrees of freedom. Similarly, the prediction time massively exceeded the mean free duration between particle collisions. Both observations were caused by the formation of mesoscopic structures. Complementary simulations of a fully 3D bed showed that the agreement of the metrics also holds for more complex motion. To calculate Lagrangian distances, we employed the Hungarian algorithm with complexity O(Np3) for which we investigated different approximations leading to significant speed ups. In particular, we tracked randomly selected subsets of grains down to 10% of their total number and evaluated their mutual distance with only minor deviations from results of the full system. Our study clearly demonstrates that fundamental dynamic properties of granular matter are widely independent from the type of distance function used to investigate them. The final choice may be made based upon performance considerations or any specific information provided by different kinds of metrics.
MoreTranslated text
Key words
recurrent particulate,eulerian metrics
AI Read Science
Must-Reading Tree
Example
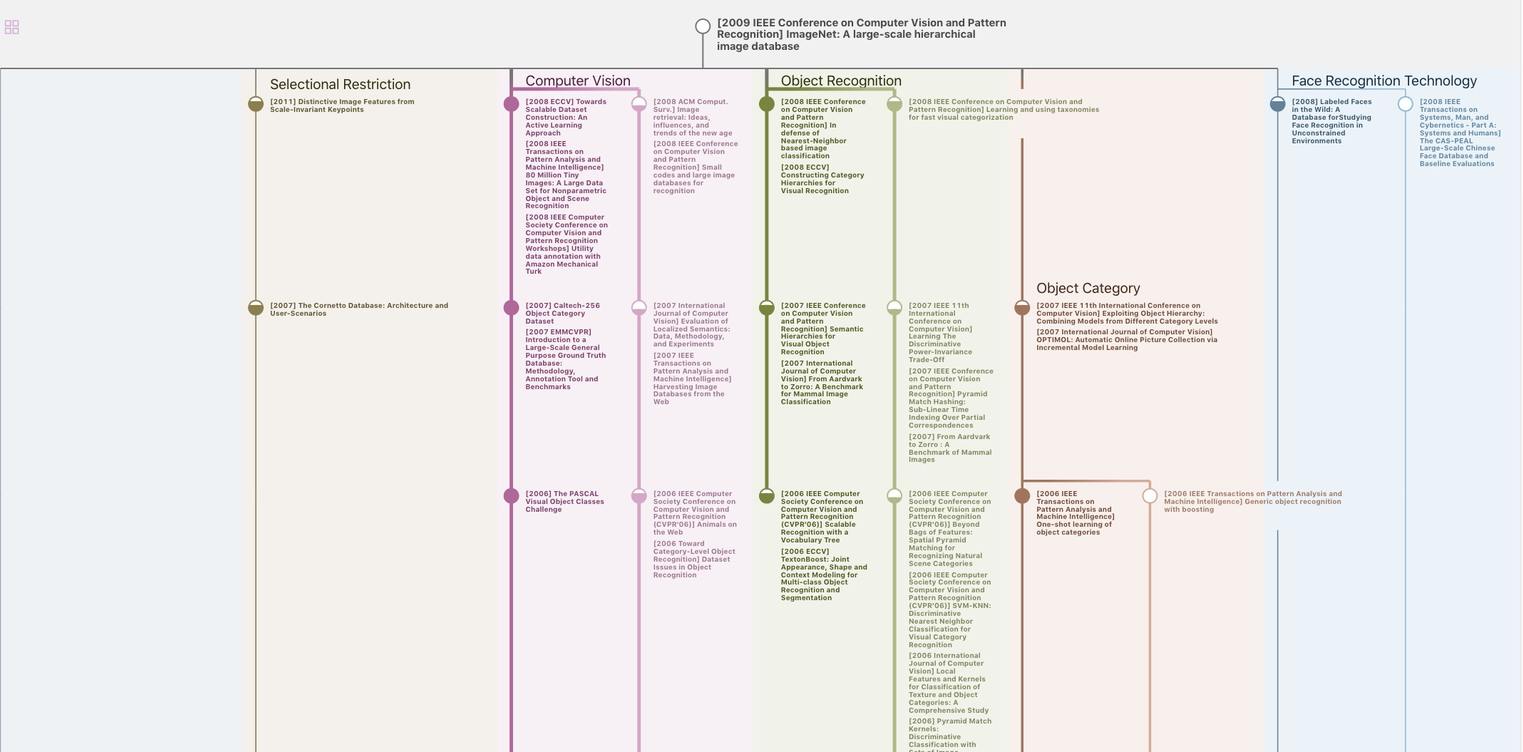
Generate MRT to find the research sequence of this paper
Chat Paper
Summary is being generated by the instructions you defined