On Making Factories Smarter through Actionable Predictions based on Time-Series Data
Procedia Manufacturing(2020)
摘要
Abstract The emergence of the Industrial Internet of Things paves the way for enhancing the real-time monitoring capabilities of contemporary factories. This can be materialised through sensors collecting data which can be further analysed. This paradigm enables the detection of indicators concerning systems’ degradation and facilitates corrective actions to be performed ahead of time. In this paper, we propose a damage prediction framework exploiting data that are coming from the core IT system in a steel industry use case. The novelty of our approach lies in the exploitation of Deep Learning techniques over streaming operational sensor data. To evaluate the framework, real-life data are collected and analysed based on daily operational activities enriched with the Remaining Useful Life (RUL). The Remaining Useful Life is automatically computed. We also periodically align the damage attribute, which is recorded in the planned activities and is performed by the maintenance engineers. We demonstrate the framework’s potential by conducting a quantitative and qualitative analysis to timely identify rare events based on historical data and predict dynamic behavioural changes in the manufacturing settings.
更多查看译文
关键词
Industry 4.0, Industrial Internet of Things Architectures, Predictive Analytics for Production Monitoring, Advanced Machine Learning and Artificial Intelligence Approaches for Industrial Use Cases
AI 理解论文
溯源树
样例
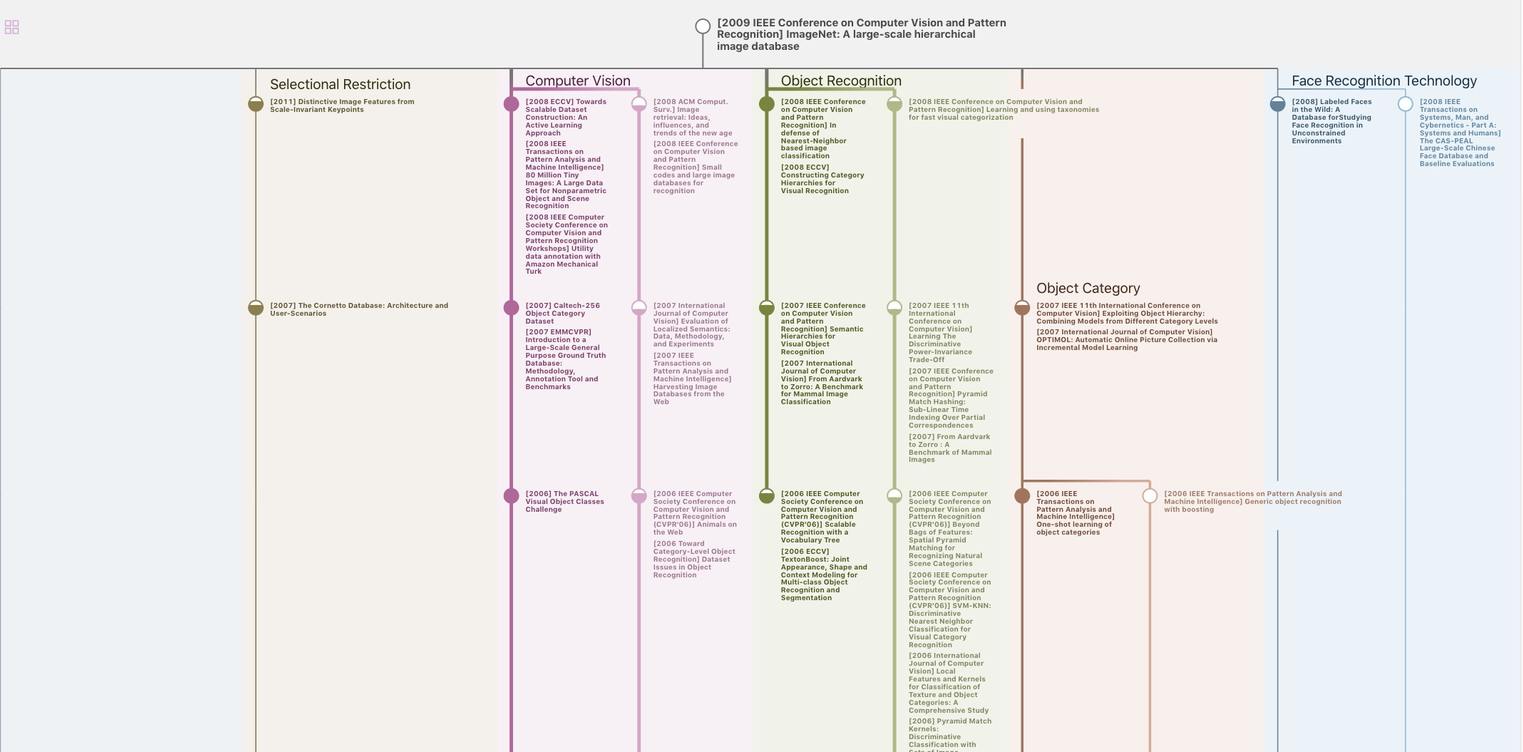
生成溯源树,研究论文发展脉络
Chat Paper
正在生成论文摘要