FTR-NAS: Fault-Tolerant Recurrent Neural Architecture Search.
ICONIP (5)(2020)
摘要
With the popularity of the applications equipped with neural networks on edge devices, robustness has become the focus of researchers. However, when deploying the applications onto the hardware, environmental noise is unavoidable, in which errors may cause applications crash, especially for the safety-critic applications. In this paper, we propose FTR-NAS to optimize recurrent neural architectures to enhance the fault tolerance. First, according to real deployment scenarios, we formalize computational faults and weight faults, which are simulated with Multiply-Accumulate (MAC)-independent and identically distributed (i.i.d) Bit-Bias (MiBB) model and Stuck-at-Fault (SAF) model, respectively. Next, we establish a multi-objective NAS framework powered by the fault models to discover high-performance and fault-tolerant recurrent architectures. Moreover, we incorporate fault-tolerant training (FTT) in the search process to further enhance the fault tolerance of the recurrent architectures. Experimentally, C-FTT-RNN and W-FTT-RNN we discovered on PTB dataset have promising fault tolerance for computational and weight faults. Besides, we further demonstrate the usefulness of the learned architectures by transferring it to WT2 dataset well.
更多查看译文
关键词
Recurrent neural network,Neural architecture search,Fault tolerance
AI 理解论文
溯源树
样例
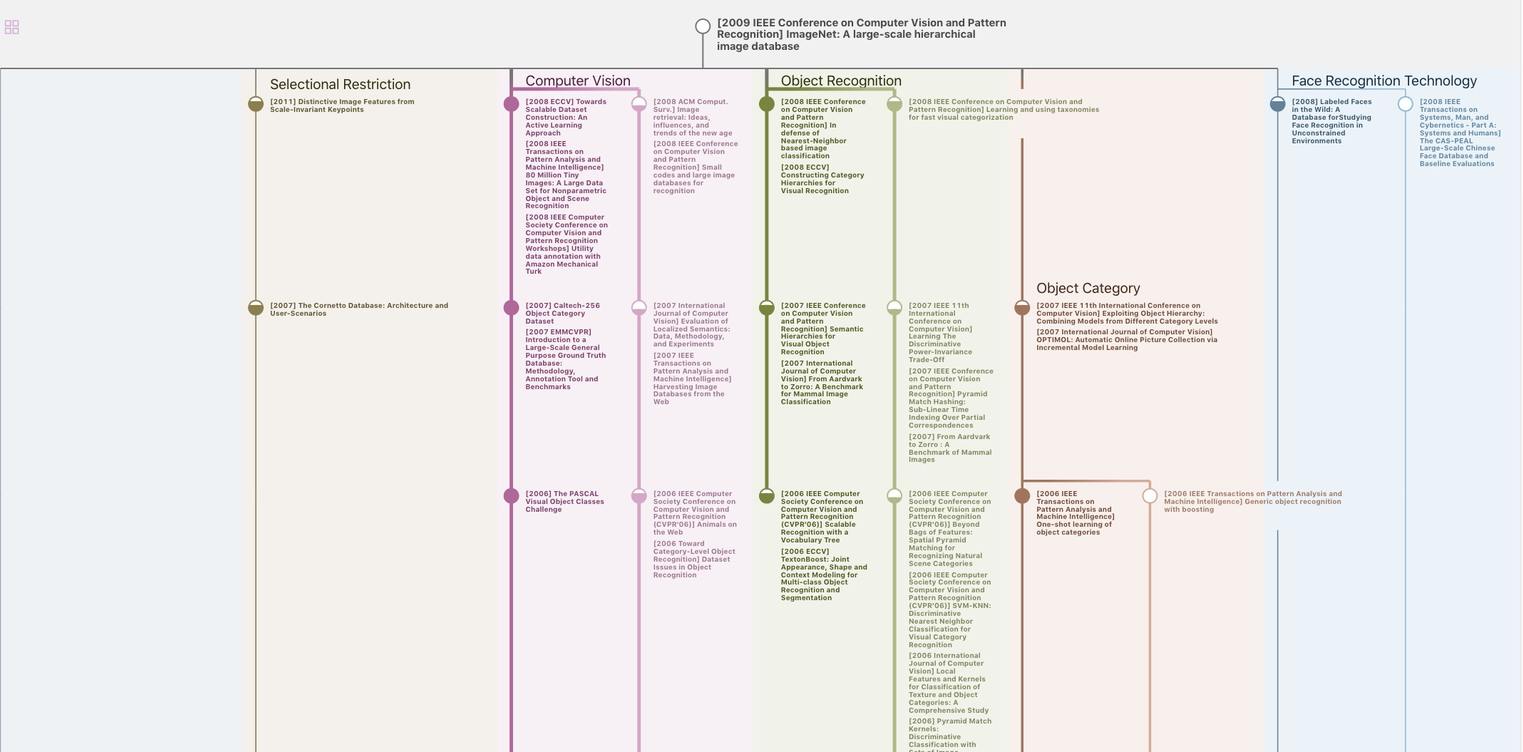
生成溯源树,研究论文发展脉络
Chat Paper
正在生成论文摘要