PART-GAN: Privacy-Preserving Time-Series Sharing.
ICANN (1)(2020)
摘要
In this paper, we provide a practical privacy-preserving generative model for time series data augmentation and sharing, called PART-GAN. Our model enables the local data curator to provide a freely accessible public generative model derived from original data for cloud storage. Compared with existing approaches, PART-GAN has three key advantages: It enables the generation of an unlimited amount of synthetic time series data under the guidance of a given classification label and addresses the incomplete and temporal irregularity issues. It provides a robust privacy guarantee that satisfies differential privacy to time series data augmentation and sharing. It addresses the trade-offs between utility and privacy by applying optimization strategies. We evaluate and report the utility and efficacy of PART-GAN through extensive empirical evaluations of real-world health/medical datasets. Even at a higher level of privacy protection, our method outperforms GAN with ordinary perturbation. It achieves similar performance with GAN without perturbation in terms of inception score, machine learning score similarity, and distance-based evaluations.
更多查看译文
关键词
Generative model, Data sharing, Privacy-preserving, Deep learning, Differential privacy
AI 理解论文
溯源树
样例
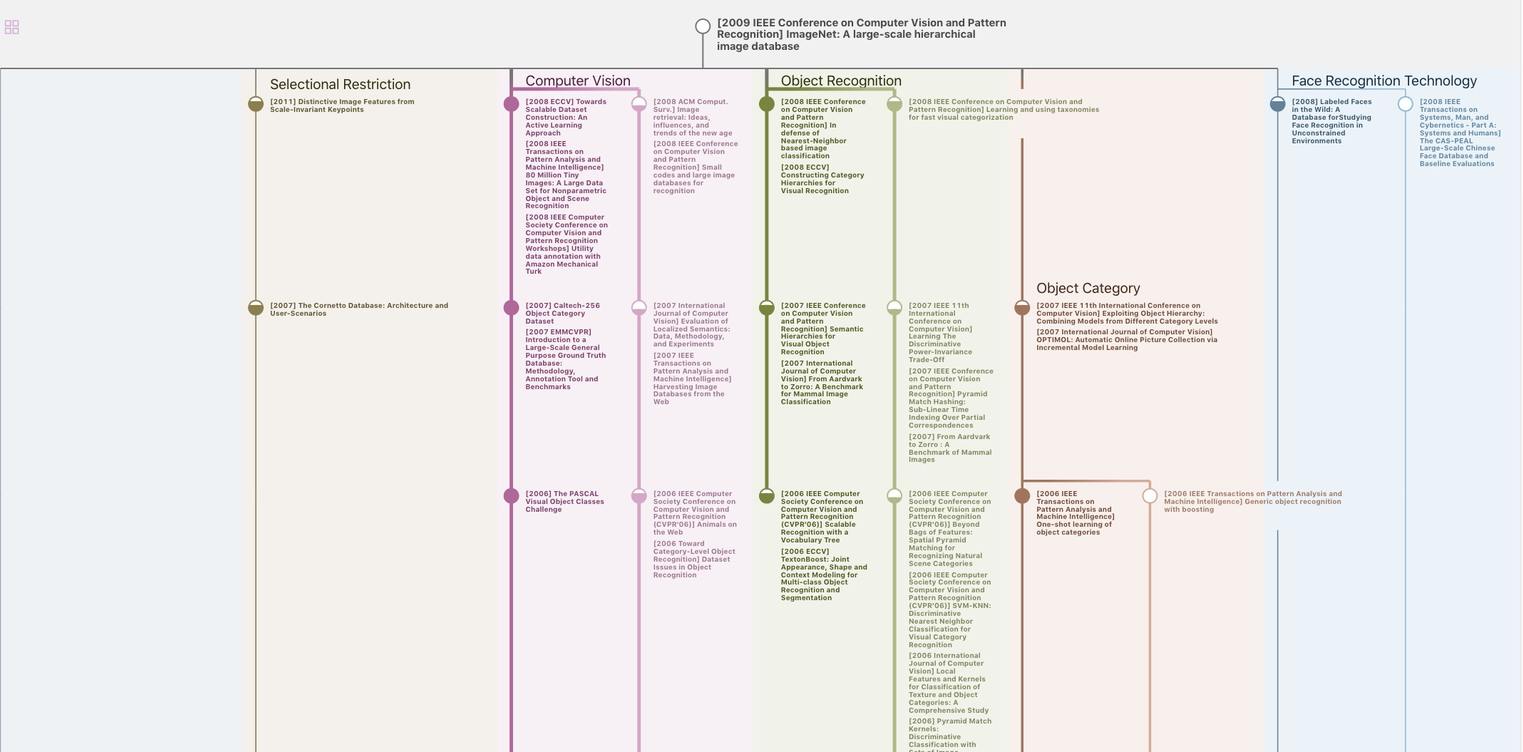
生成溯源树,研究论文发展脉络
Chat Paper
正在生成论文摘要