An interpretable deep-learning architecture of capsule networks for identifying cell-type gene expression programs from single-cell RNA-sequencing data
NATURE MACHINE INTELLIGENCE(2020)
摘要
Single-cell RNA sequencing (scRNA-seq) technologies are used to characterize the heterogeneity of cells in cell types, developmental stages and spatial positions. The rapid accumulation of scRNA-seq data has enabled single-cell-type labelling to transform single-cell transcriptome analysis. Here we propose an interpretable deep-learning architecture using capsule networks (called scCapsNet). A capsule structure (a neuron vector representing a set of properties of a specific object) captures hierarchical relations. By utilizing competitive single-cell-type recognition, the scCapsNet model is able to perform feature selection to identify groups of genes encoding different subcellular types. The RNA expression signatures, which enable subcellular-type recognition, are effectively integrated into the parameter matrices of scCapsNet. This characteristic enables the discovery of gene regulatory modules in which genes interact with each other and are closely related in function, but present distinct expression patterns.
更多查看译文
关键词
Gene expression,Machine learning,RNA sequencing,Engineering,general
AI 理解论文
溯源树
样例
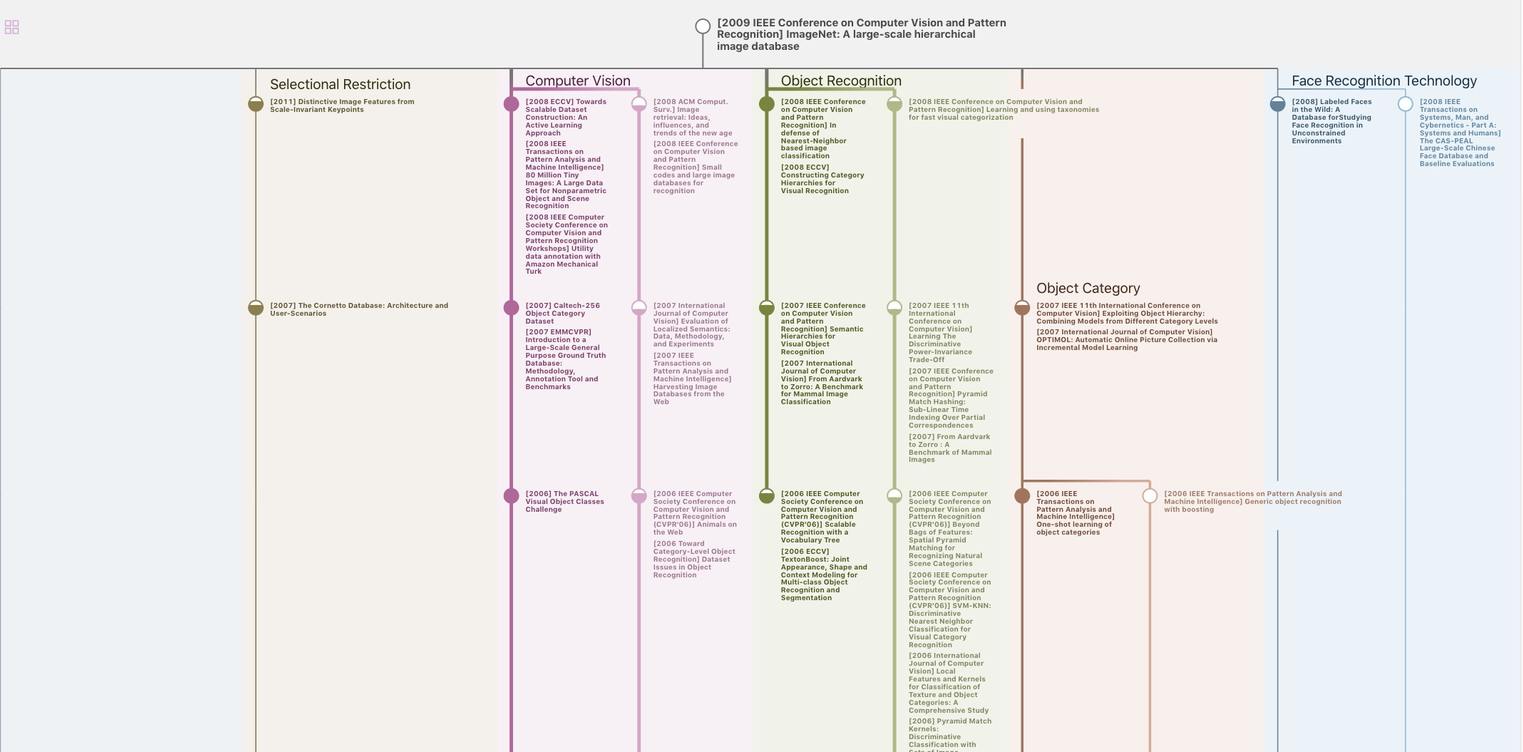
生成溯源树,研究论文发展脉络
Chat Paper
正在生成论文摘要