Dsunet: A New Network Structure For Detection And Segmentation Of Ultrasound Breast Lesions
JOURNAL OF MEDICAL IMAGING AND HEALTH INFORMATICS(2020)
摘要
Breast cancer is one of the leading causes of death among the women worldwide. The clinical medical system urgently needs an accurate and automatic breast segmentation method in order to detect the breast ultrasound lesions. Recently, some studies show that deep learning methods based on fully convolutional network, have demonstrated a competitive performance in breast ultrasound segmentation. However, some features are missed in the Unet in case of down-sampling that results in a low segmentation accuracy. Furthermore, there is a semantic gap between the feature maps of decoder and encoder in Unet, so the simple fusion of high and low level features is not conducive to the semantic classification of pixels. In addition, the poor quality of breast ultrasound also affects the accuracy of image segmentation. To solve these problems, we propose a new end-toend network model called Dense skip Unet (DsUnet), which consists of the Unet backbone, short skip connection and deep supervision. The proposed method can effectively avoid the missing of feature information caused by down-sampling and implement the fusion of multilevel semantic information. We used a new loss function to optimize the DsUnet, which is composed of a binary cross-entropy and dice coefficient. We employed the True Positive Fraction (TPF), False Positives per image (FPs) and F-measure as performance metrics for evaluating various methods. In this paper, we adopted the UDIAT 212 dataset and the experimental results validate that our new approach achieved better performance than other existing methods in detecting and segmenting the ultrasound breast lesions. When we used the DsUnet model and new loss function (binary cross-entropy + dice coefficient), the best performance indexes can be achieved, i.e., 0.87 in TPF, 0.13 in FPs/image and 0.86 in F-measure.
更多查看译文
关键词
Lesion Detection,Ultrasound,Breast Cancer,Semantic Segmentation,Deep Learning,DsUnet
AI 理解论文
溯源树
样例
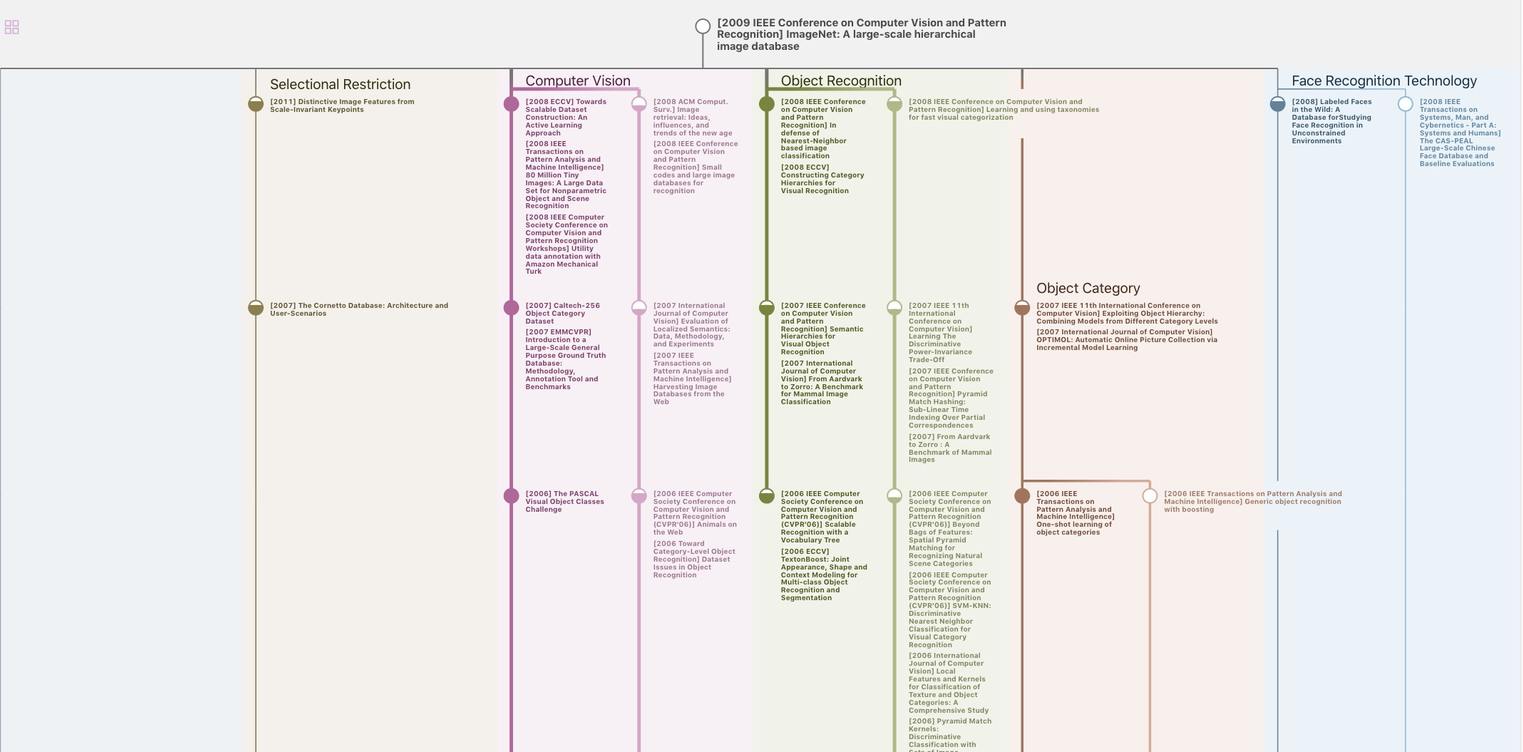
生成溯源树,研究论文发展脉络
Chat Paper
正在生成论文摘要