Knowledge-Based Segmentation To Improve Accuracy And Explainability In Non-Technical Losses Detection
ENERGIES(2020)
摘要
Utility companies have a great interest in identifying energy losses. Here, we focus on Non-Technical Losses (NTL), which refer to losses caused by utility theft or meter errors. Typically, utility companies resort to machine learning solutions to automate and optimise the identification of such losses. This paper extends an existing NTL-detection framework: by including knowledge-based NTL segmentation, we have detected some opportunities for improving the accuracy and the explanations provided to the utility company. Our improved models focus on specific types of NTL and therefore, the explanations provided are easier to interpret, allowing stakeholders to make more informed decisions. The improvements and results presented in the article may benefit other industrial frameworks.
更多查看译文
关键词
Non-Technical Losses, machine learning, supervised learning, ensemble learning, explainability
AI 理解论文
溯源树
样例
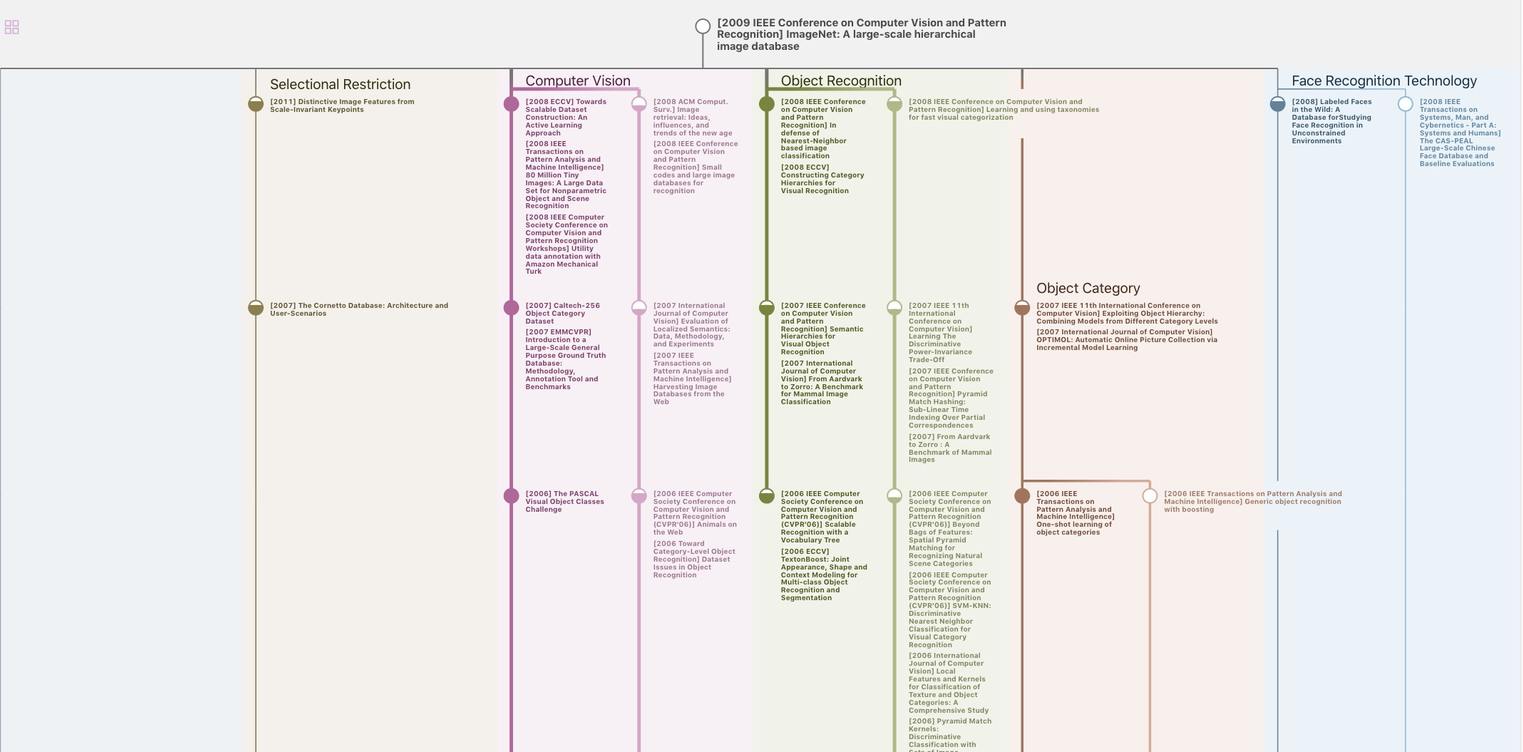
生成溯源树,研究论文发展脉络
Chat Paper
正在生成论文摘要