Deep Phase Retrieval: Analyzing Over-Parameterization In Phase Retrieval
SIGNAL PROCESSING(2021)
Abstract
Phase retrieval aims to recover the phase of a complex-valued signal from magnitude measurements. Recently, approaches based on non-convex optimization which solve the phase retrieval problem directly using gradient descent methods have become more and more popular. In this paper, we propose a non convex Deep Phase Retrieval (DeepPR) method which over-parameterizes the decision variable as a series of multiplications between over-parameterized decision variables. Gradient descent is then used to optimize the decision variables. When the step size is assumed to be fixed, theoretical analysis shows that over-parameterization does not complicate the original problem and introduces an implicit acceleration effect for optimization. Numerical tests verify this benefit by showing that our proposed model achieves a faster convergence rate compared to the benchmark algorithm. When spectral method is used to determine the initialization of gradient refinement, our simulation results show that DeepPR method enjoys higher empirical success rate than the benchmark algorithm at low sampling rate condition. (C) 2020 Elsevier B.V. All rights reserved.
MoreTranslated text
Key words
Phase retrieval, Over-parameterization, Non-convex optimization, Deep model
AI Read Science
Must-Reading Tree
Example
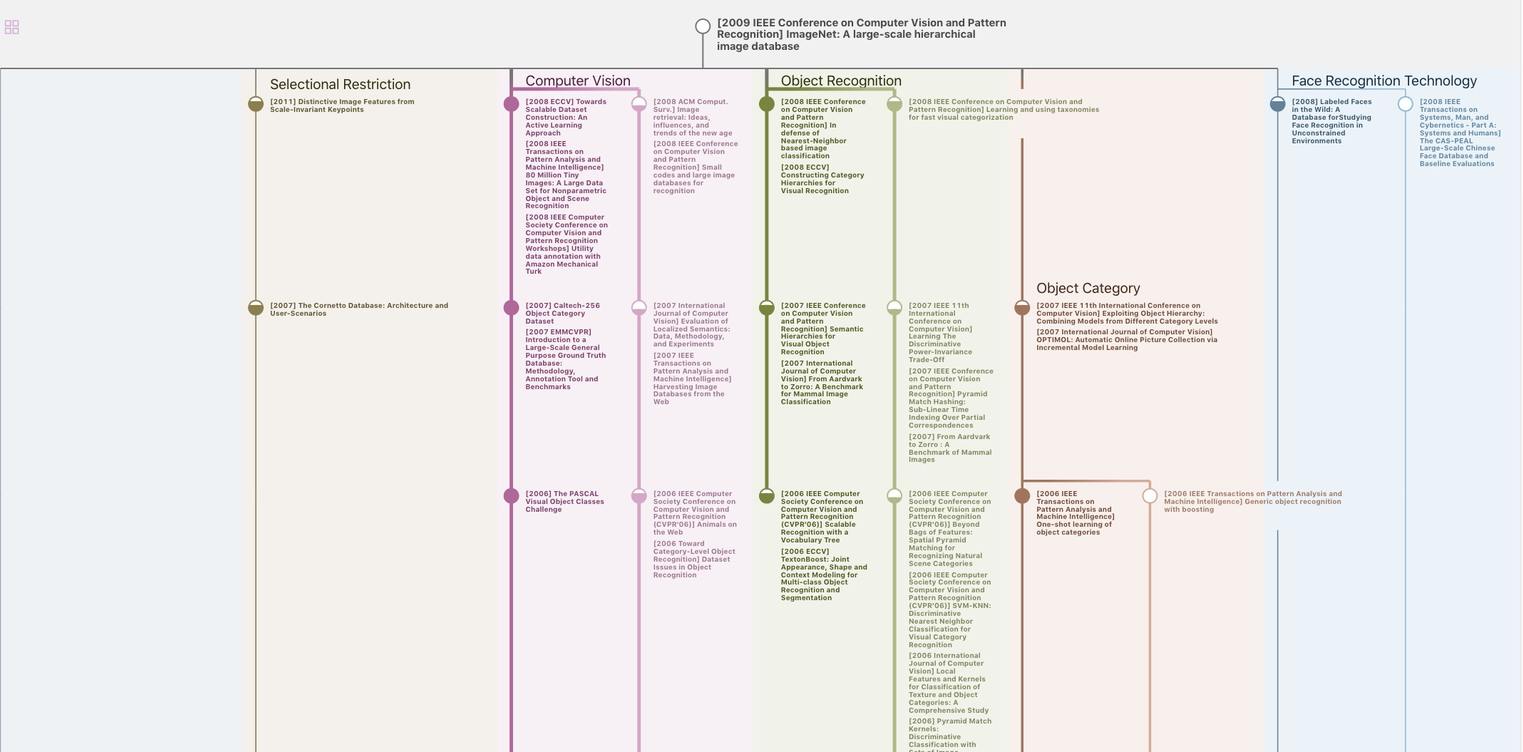
Generate MRT to find the research sequence of this paper
Chat Paper
Summary is being generated by the instructions you defined