How big should this object be? Perceptual influences on viewing-size preferences
Cognition(2022)
摘要
When viewing objects depicted in a frame, observers prefer to view large objects like cars in larger sizes and smaller objects like cups in smaller sizes. That is, the visual size of an object that “looks best” is linked to its typical physical size in the world. Why is this the case? One intuitive possibility is that these preferences are driven by semantic knowledge: For example, when we recognize a sofa, we access our knowledge about its real-world size, and this influences what size we prefer to view the sofa within a frame. However, might visual processing play a role in this phenomenon—that is, do visual features that are related to big and small objects look better at big and small visual sizes, respectively, even when observers do not have explicit access to semantic knowledge about the objects? To test this possibility, we used “texform” images, which are synthesized versions of recognizable objects, which critically retain local perceptual texture and coarse contour information, but are no longer explicitly recognizable. To test for visual size preferences, we first used a size adjustment task, and the results were equivocal. However, clear results were obtained using a two-interval forced choice task, in which each texform was presented at the preferred visual size of its corresponding original image, and a visual size slightly bigger or smaller. Observers consistently selected the texform presented at the canonical visual size as the more aesthetically pleasing one. An additional control experiment ruled out alternative explanations related to size priming effects. These results suggest that the preferred visual size of an object depends not only on explicit knowledge of its real-world size, but also can be evoked by mid-level visual features that systematically covary with an object's real-world size.
更多查看译文
关键词
Aesthetic preferences,Size,Mid-level visual features,Texture,Object recognition
AI 理解论文
溯源树
样例
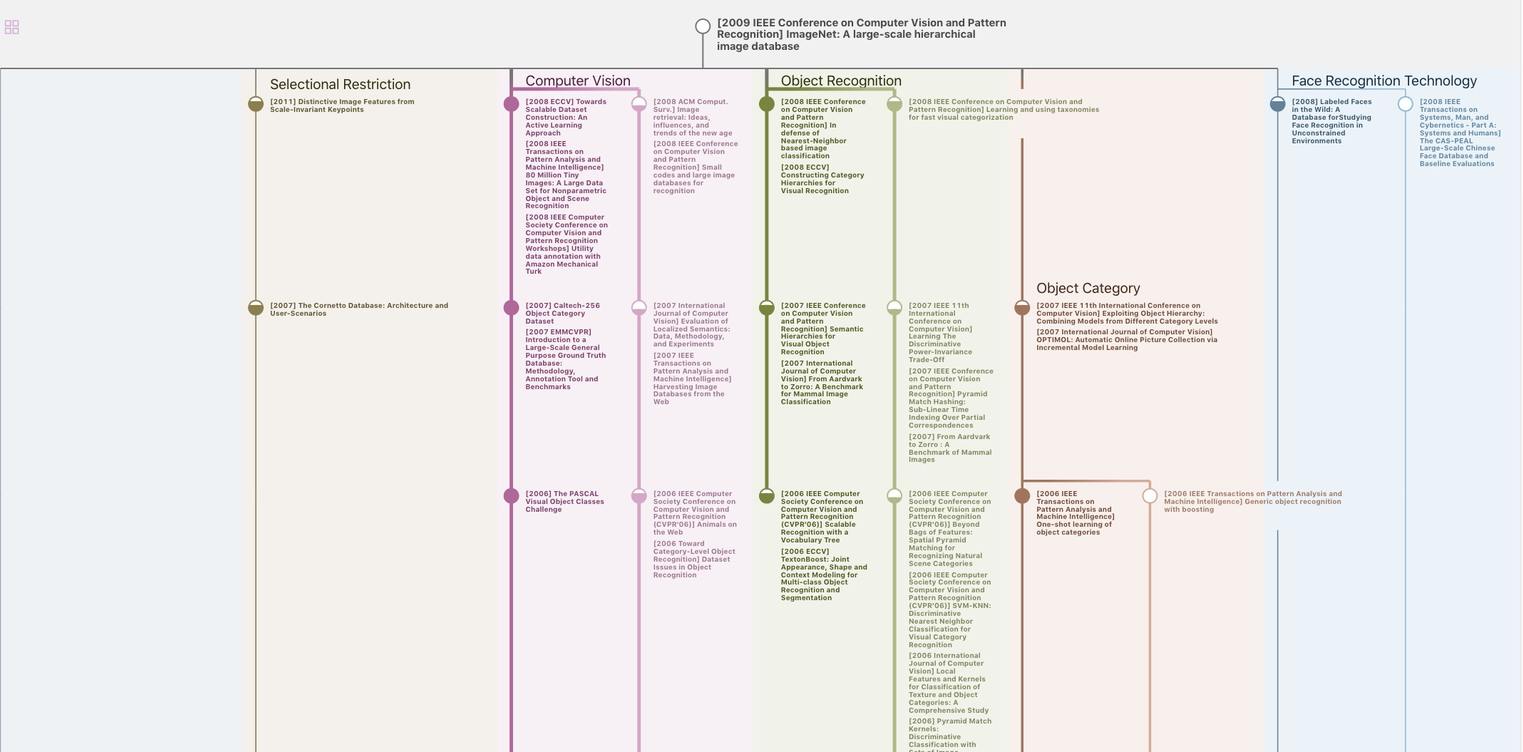
生成溯源树,研究论文发展脉络
Chat Paper
正在生成论文摘要