A multi-channel anomaly detection method with feature selection and multi-scale analysis
Computer Networks(2021)
摘要
This paper proposes a novel multi-channel network traffic anomaly detection method combined with the idea of multi-scale decomposition, feature selection and fusion. Our method includes a feature selection and fusion module, which extracts the most important features from redundancy traffic features and fuses the selected features, a multi-scale decomposition module, and a multi-channel Generalized Likelihood Ratio Test (GLRT) detection module, for anomaly detection and decision-making. The advantage of our method is that, first, it only considers the selected features and reduces the amount of computation. Second, compared with traditional anomaly detection methods that usually work on each scale independently thus mainly focused on temporally correlated traffic, our method fully explores the internal frequency–time correlations within multiple scales. It can be verified with experiments that this method performs better than other traditional methods, thus gives a new sight on the anomaly detection with different types of traffic data.
更多查看译文
关键词
Network traffic,Multi-channel GLRT,Anomaly detection,EEMD,Feature selection
AI 理解论文
溯源树
样例
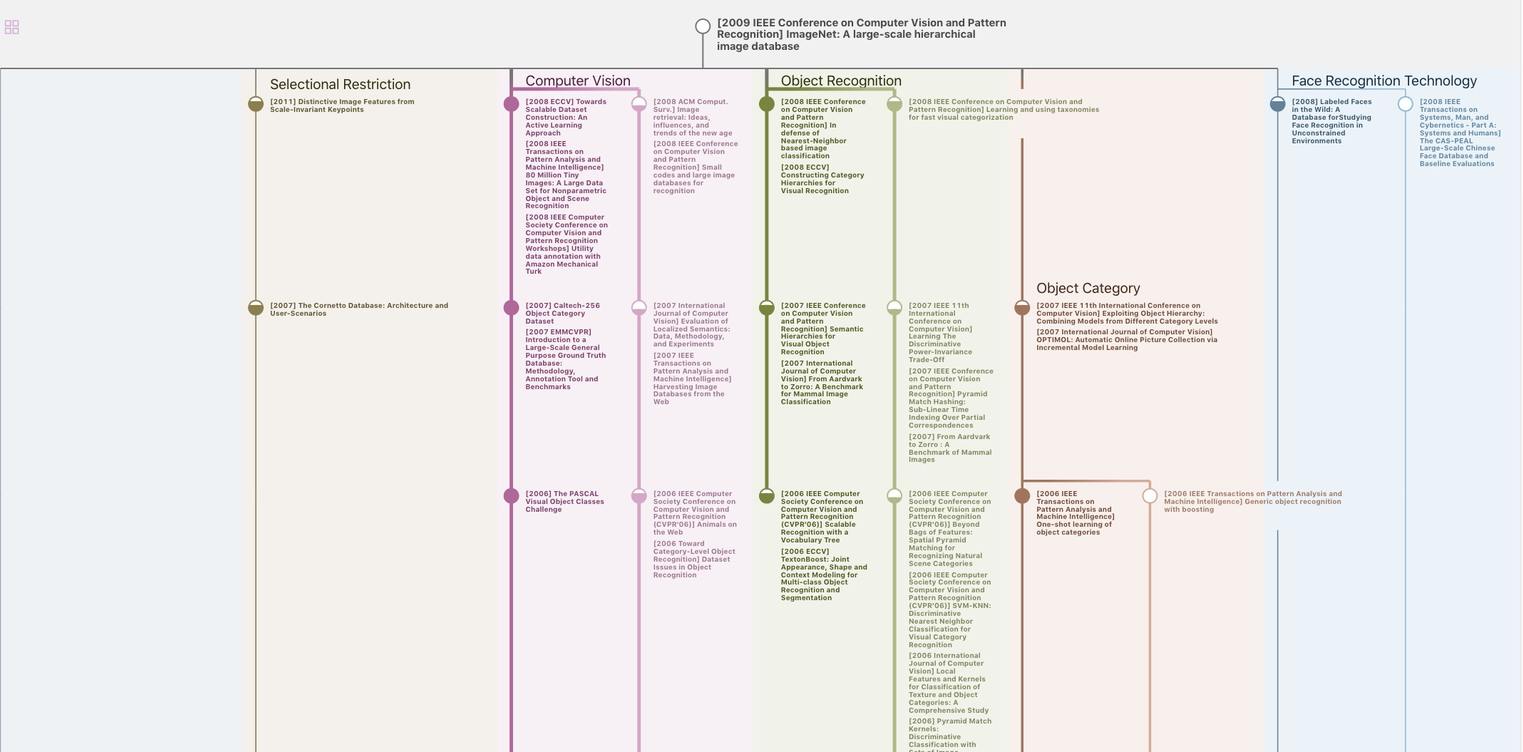
生成溯源树,研究论文发展脉络
Chat Paper
正在生成论文摘要