Deep Learning Survival Analysis For Brain Metastases Treated With Stereotactic Radiosurgery
INTERNATIONAL JOURNAL OF RADIATION ONCOLOGY BIOLOGY PHYSICS(2020)
Abstract
Modeling survival outcomes for patients with brain metastasis remains a clinical challenge. Current methods, like the Graded Prognostic Assessment (GPA), may be limited because they 1) employ only clinical predictors and 2) are derived from generalized linear models which assume a constant change in a predictor leads to a constant change in survival. Deep learning represents a potentially more effective method for outcome prediction because of the ability to analyze pixel-level imaging predictors to create non-linear survival models. We hypothesized that a deep learning model derived from quantitative imaging predictors would be more effective than traditional models of survival in patients with brain metastases. We analyzed 220 patients with a single brain metastasis treated with primary stereotactic radiosurgery (SRS) at our institution between 2000-2018. The primary outcome of interest was overall survival following SRS treatment. Two types of quantitative imaging features were extracted from T1 post contrast MRI images of each brain metastasis and used as predictors of survival: 1) 851 3D radiomic features and 2) 256 features extracted from a trained ResNet-LSTM deep learning model. Minimum redundancy maximum relevance was used for dimensionality reduction. Relevant features were then trained on a proportional hazard neural network architecture to model overall survival. The disease specific GPA which uses age, Karnofsky performance status, presence of extracranial metastases, and disease specific molecular characteristics for survival predictions was used as our traditional model. Discriminatory ability of each model was assessed with calculated concordance indices (c-index) using 100 bootstrapped samples of 176 patients and evaluated for statistical significance in difference from the GPA with two-sample t-tests. For all 220 patients, median overall survival was 24 months, median age was 62 years, and the most common primary sites were NSCLC (43.6%), melanoma (19.5%), breast (8.6%), renal (7.3%), GI (6.4%) and SCLC (5.5%). Both deep learning models using quantitative imaging features had superior discriminatory ability compared to the GPA (Table 1). Model performance was similar when comparing radiomic features (c-index: 0.694) and ResNet-LSTM features (c-index: 0.685). Compared to the GPA (c-index: 0.410), both deep learning models using quantitative imaging features showed significantly improved performance (p<0.001). Deep learning models using quantitative imaging features showed a greater ability to model survival in patients with brain metastases compared to traditional linear models using clinical predictors. These models represent promising methods to improve survival prognostication for patients diagnosed with brain metastases.Abstract 1102; TableModelC-Index95% CIGPA0.410[0.365, 0.448]Deep Learning ResNet-LSTM0.685[0.619, 0.735]Deep Learning Radiomic0.694[0.624, 0.747] Open table in a new tab
MoreTranslated text
Key words
Brain Metastases,Prognostic Assessment,Stereotactic Body Radiation Therapy,Tumor Staging
AI Read Science
Must-Reading Tree
Example
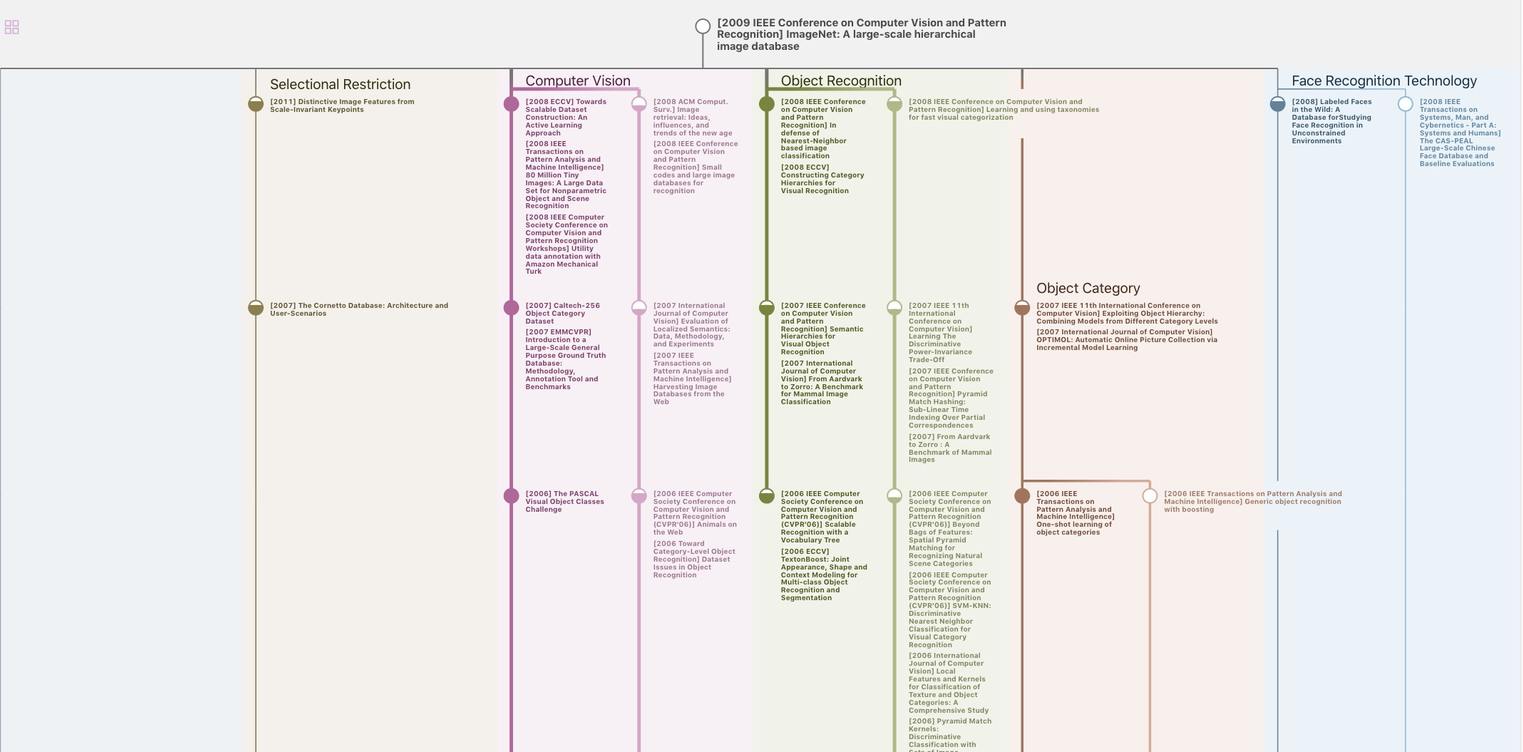
Generate MRT to find the research sequence of this paper
Chat Paper
Summary is being generated by the instructions you defined