Efficient Machine Learning Models For Prediction Of Concrete Strengths
CONSTRUCTION AND BUILDING MATERIALS(2021)
摘要
In this study, an efficient implementation of machine learning models to predict compressive and tensile strengths of high-performance concrete (HPC) is presented. Four predictive algorithms including support vector regression (SVR), multilayer perceptron (MLP), gradient boosting regressor (GBR), and extreme gradient boosting (XGBoost) are employed. The process of hyperparameter tuning is based on random search that results in trained models with better predictive performances. In addition, the missing data is handled by filling with the mean of the available data which allows more information to be used in the training process. The results on two popular datasets of compressive and tensile strengths of high performance concrete show significant improvement of the current approach in terms of both prediction accuracy and computational effort. The comparative studies reveal that, for this particular prediction problem, the trained models based on GBR and XGBoost perform better than those of SVR and MLP. (C) 2020 Elsevier Ltd. All rights reserved.
更多查看译文
关键词
High performance concrete, Ensemble learning, Support vector machine, Multi-layer Perceptron, Tree-based algorithms
AI 理解论文
溯源树
样例
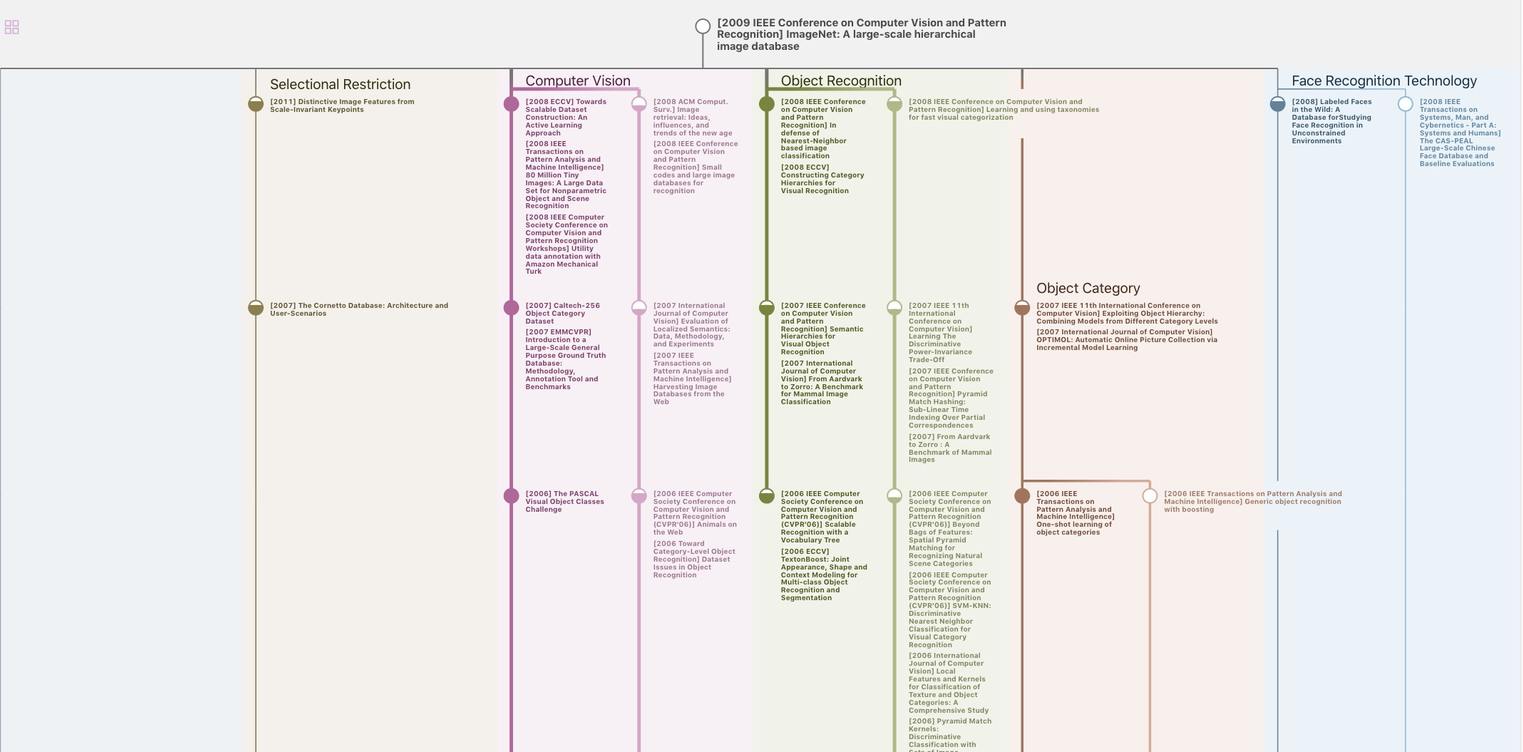
生成溯源树,研究论文发展脉络
Chat Paper
正在生成论文摘要