Social media rumor refutation effectiveness: Evaluation, modelling and enhancement.
Inf. Process. Manag.(2021)
Abstract
Motivated by the practical needs of enhancing social media rumor refutation effectiveness, this paper is dedicated to develop a proper rumor refutation effectiveness index (REI), identify key factors influencing REI and propose decision making suggestions for rumor refutation platforms. 298,118 pieces of comments and 185,209 pieces of the reposters’ verification status of 248 rumor refutation microblogs on Sina Weibo (the Chinese equivalent of Twitter) are collected during a 1-year period using a web crawler. To extract the text characteristics and analyze the sentiment of the rumor refutation microblogs, Natural Language Processing (NLP) approaches are applied. To explore the relationship between REI and the content and contextual factors of the rumor refutation microblogs, four regression models based on the collected data are established, namely linear regression model, Support Vector regression model (SVR), Extreme Gradient Boosting regression model (XGBoostRegressor) and Light Gradient Boosting Machine regression model (LGBMRegressor). The LGBMRegressor has the best goodness-of-fit among the compared regression models. Then, SHapley Additive exPlanations (SHAP) is employed to visualize and explain the LGBMRegressor results. Decision making suggestions for rumor refutation platforms on how to organize rumor refutation microblogs under different situations such as rumor category, author’s influence and heat of topics are proposed.
MoreTranslated text
Key words
Rumor refutation effectiveness,Natural Language Processing,Big data of social media,LGBMRegressor
AI Read Science
Must-Reading Tree
Example
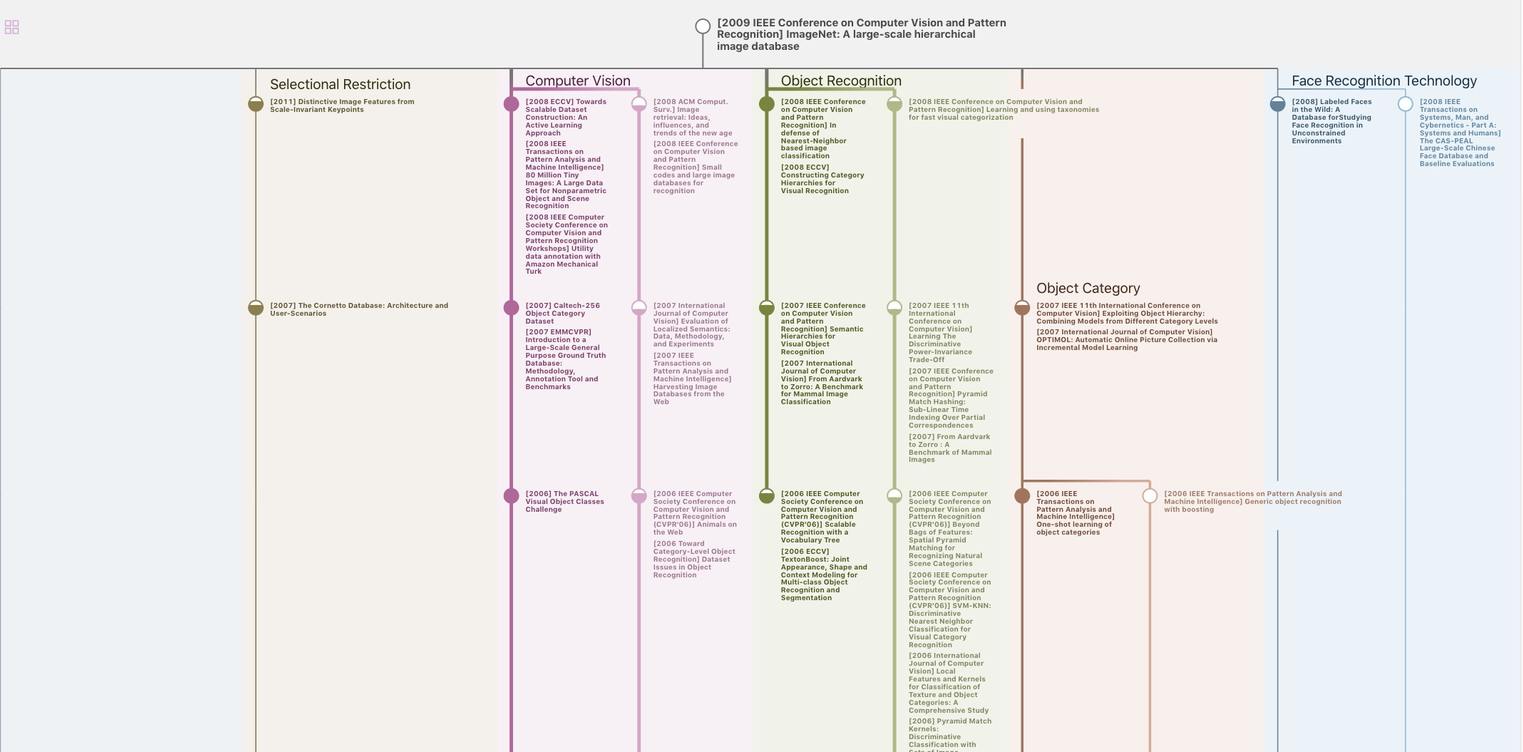
Generate MRT to find the research sequence of this paper
Chat Paper
Summary is being generated by the instructions you defined