RX Equalization for a High-Speed Channel based on Bayesian Active Learning using Dropout
2020 IEEE 29th Conference on Electrical Performance of Electronic Packaging and Systems (EPEPS)(2020)
摘要
Determining optimal equalization settings in highspeed bus design simulations is becoming more important due to increased complexity and data rates of current server systems, but it is also time and resource consuming. In this paper, a probabilistic machine learning technique, Bayesian Active Learning using Dropout (BAL-DO), is utilized to perform RX equalization and optimization to address this issue. Largest HEYE opening and corresponding equalization settings are obtained with high prediction accuracy without performing extensive time-domain analysis, thereby significantly reducing the cost of engineering time and computational resources.
更多查看译文
关键词
Dropout,optimal equalization settings,probabilistic machine learning technique,Bayesian active learning,RX equalization,equalization settings,time-domain analysis,high-speed channel,high-speed bus design simulations
AI 理解论文
溯源树
样例
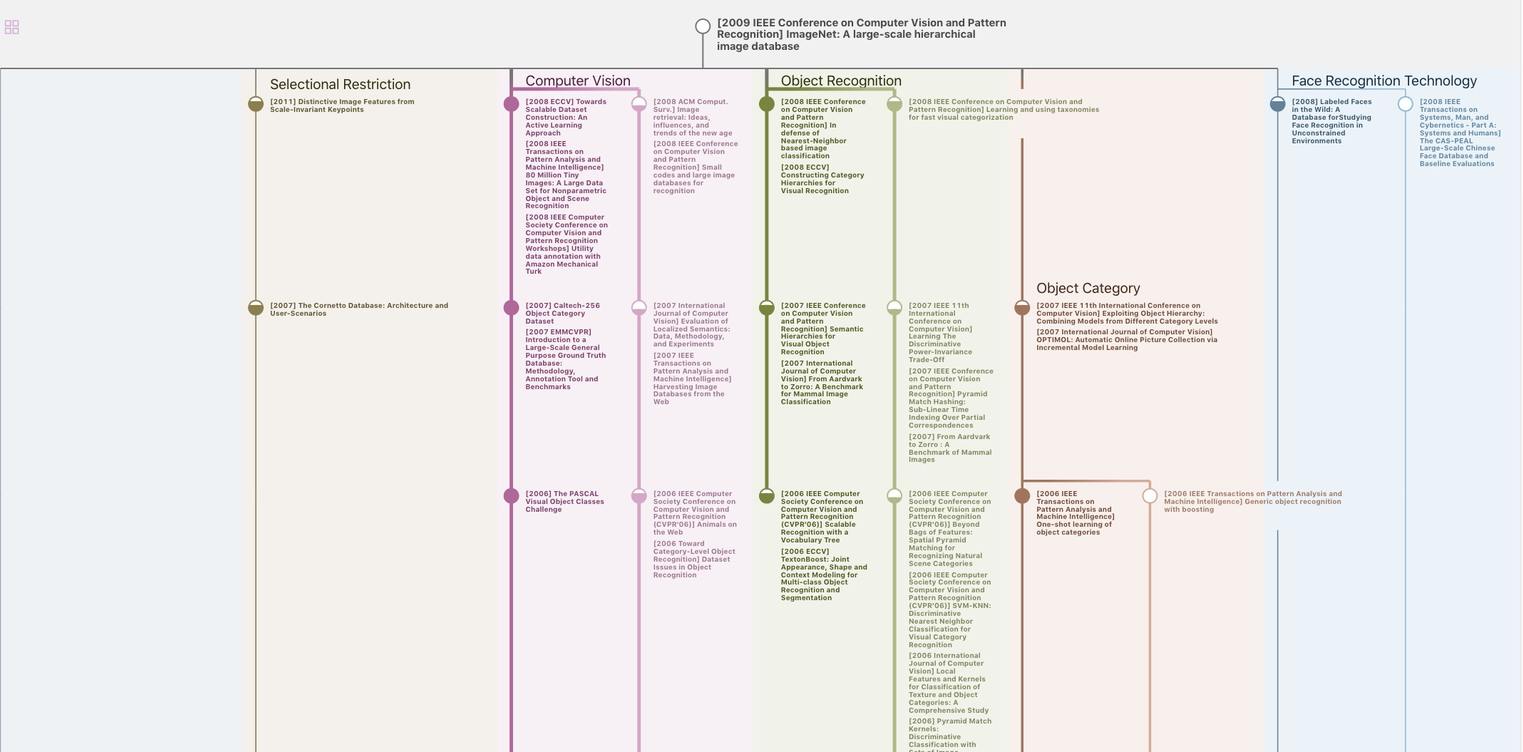
生成溯源树,研究论文发展脉络
Chat Paper
正在生成论文摘要