Analysis Of Population Functional Connectivity Data Via Multilayer Network Embeddings
NETWORK SCIENCE(2021)
摘要
Population analyses of functional connectivity have provided a rich understanding of how brain function differs across time, individual, and cognitive task. An important but challenging task in such population analyses is the identification of reliable features that describe the function of the brain, while accounting for individual heterogeneity. Our work is motivated by two particularly important challenges in this area: first, how can one analyze functional connectivity data over populations of individuals, and second, how can one use these analyses to infer group similarities and differences. Motivated by these challenges, we model population connectivity data as a multilayer network and develop the multi-node2vec algorithm, an efficient and scalable embedding method that automatically learns continuous node feature representations from multilayer networks. We use multi-node2vec to analyze resting state fMRI scans over a group of 74 healthy individuals and 60 patients with schizophrenia. We demonstrate how multilayer network embeddings can be used to visualize, cluster, and classify functional regions of the brain for these individuals. We furthermore compare themultilayer network embeddings of the two groups. We identify significant differences between the groups in the default mode network and salience network-findings that are supported by the triple network model theory of cognitive organization. Our findings reveal that multi-node2vec is a powerful and reliable method for analyzing multilayer networks. Data and publicly available code are available at https://github.com/jdwilson4/multi-node2vec.
更多查看译文
关键词
multilayer networks, network embedding, node2vec, Skip-gram, functional connectivity, imaging, network neuroscience
AI 理解论文
溯源树
样例
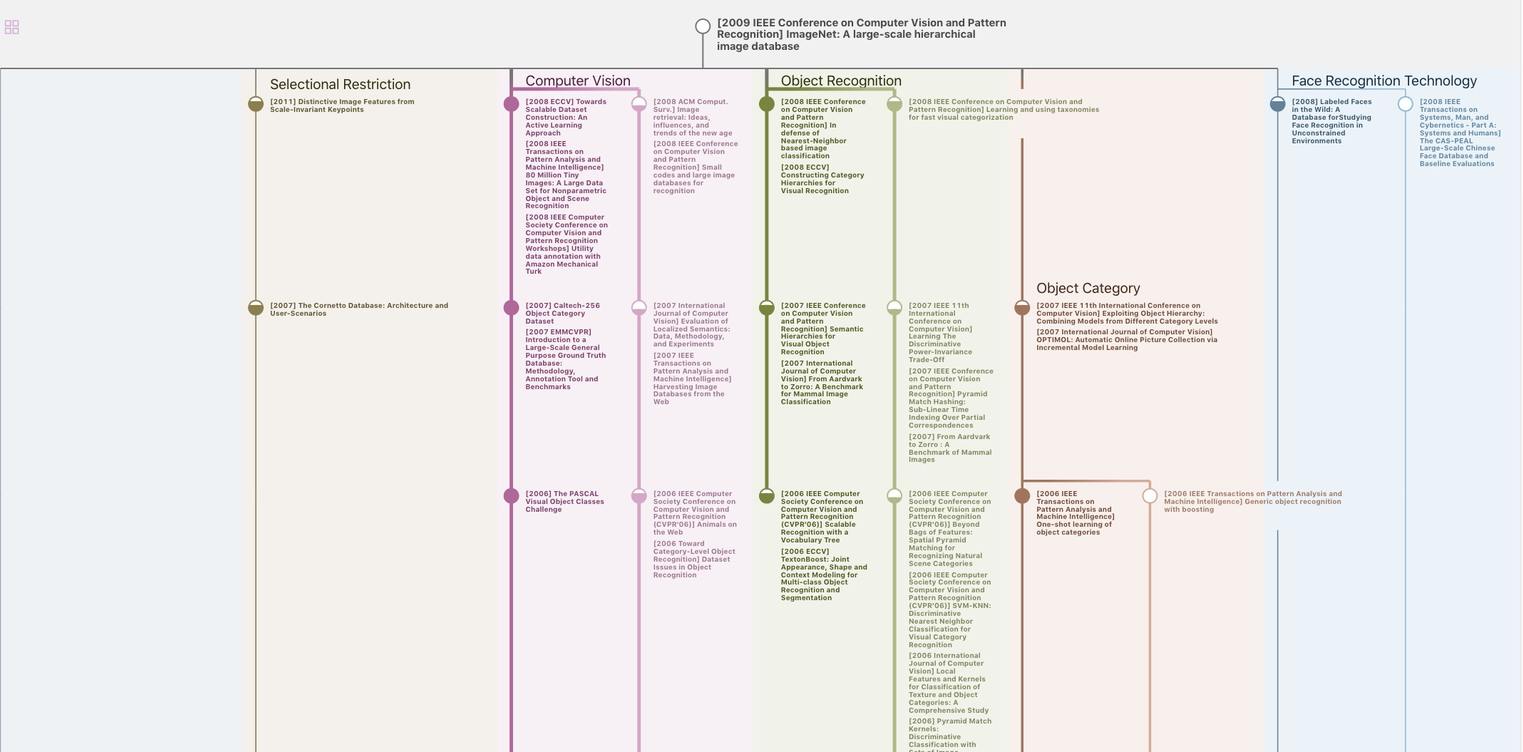
生成溯源树,研究论文发展脉络
Chat Paper
正在生成论文摘要