A Layer-Wise Information Reinforcement Approach to Improve Learning in Deep Belief Networks.
international conference on artificial intelligence and soft computing(2020)
Abstract
With the advent of deep learning, the number of works proposing new methods or improving existent ones has grown exponentially in the last years. In this scenario, "very deep" models were emerging, once they were expected to extract more intrinsic and abstract features while supporting a better performance. However, such models suffer from the gradient vanishing problem, i.e., backpropagation values become too close to zero in their shallower layers, ultimately causing learning to stagnate. Such an issue was overcome in the context of convolution neural networks by creating "shortcut connections" between layers, in a so-called deep residual learning framework. Nonetheless, a very popular deep learning technique called Deep Belief Network still suffers from gradient vanishing when dealing with discriminative tasks. Therefore, this paper proposes the Residual Deep Belief Network, which considers the information reinforcement layer-by-layer to improve the feature extraction and knowledge retaining, that support better discriminative performance. Experiments conducted over three public datasets demonstrate its robustness concerning the task of binary image classification.
MoreTranslated text
Key words
learning,deep,networks,layer-wise
AI Read Science
Must-Reading Tree
Example
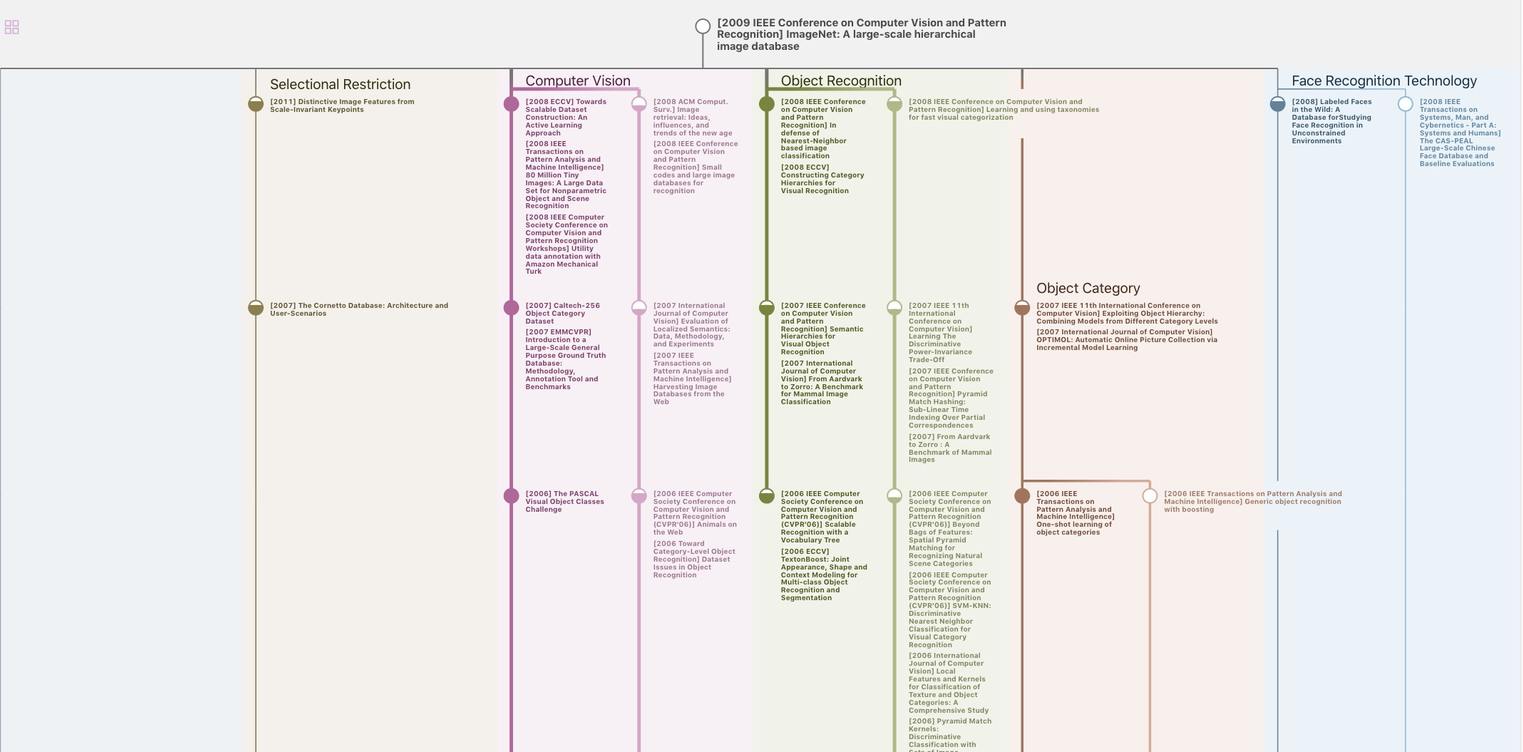
Generate MRT to find the research sequence of this paper
Chat Paper
Summary is being generated by the instructions you defined