Automatic defects detection and classification of low carbon steel WAAM products using improved remanence/magneto-optical imaging and cost-sensitive convolutional neural network
Measurement(2021)
Abstract
Wire arc additive metal manufacturing (WAAM) is one of the most revolutionary and popular manufacturing processes. However, the poor quality is an important factor restricting the development of this technology. In particular, it is difficult to detect the small defects on the surface and subsurface of the manufactured products. To cope with this issue, we propose a new method for automatic defects detection and classification of low carbon steel WAAM products using improved remanence/magneto-optical imaging and cost-sensitive convolutional neural network. The improved remanence/magneto-optical imaging is used to obtain clear magneto-optical images. A convolutional neural network model is then deployed to detect the defects in magnetooptical images. The proposed method is effective in automatic detection of the surface defects of low-carbon steel WAAM products.
MoreTranslated text
Key words
Wire arc additive manufacturing,Magneto-optical imaging,Image enhancement,Convolutional neural network,Defect classification
AI Read Science
Must-Reading Tree
Example
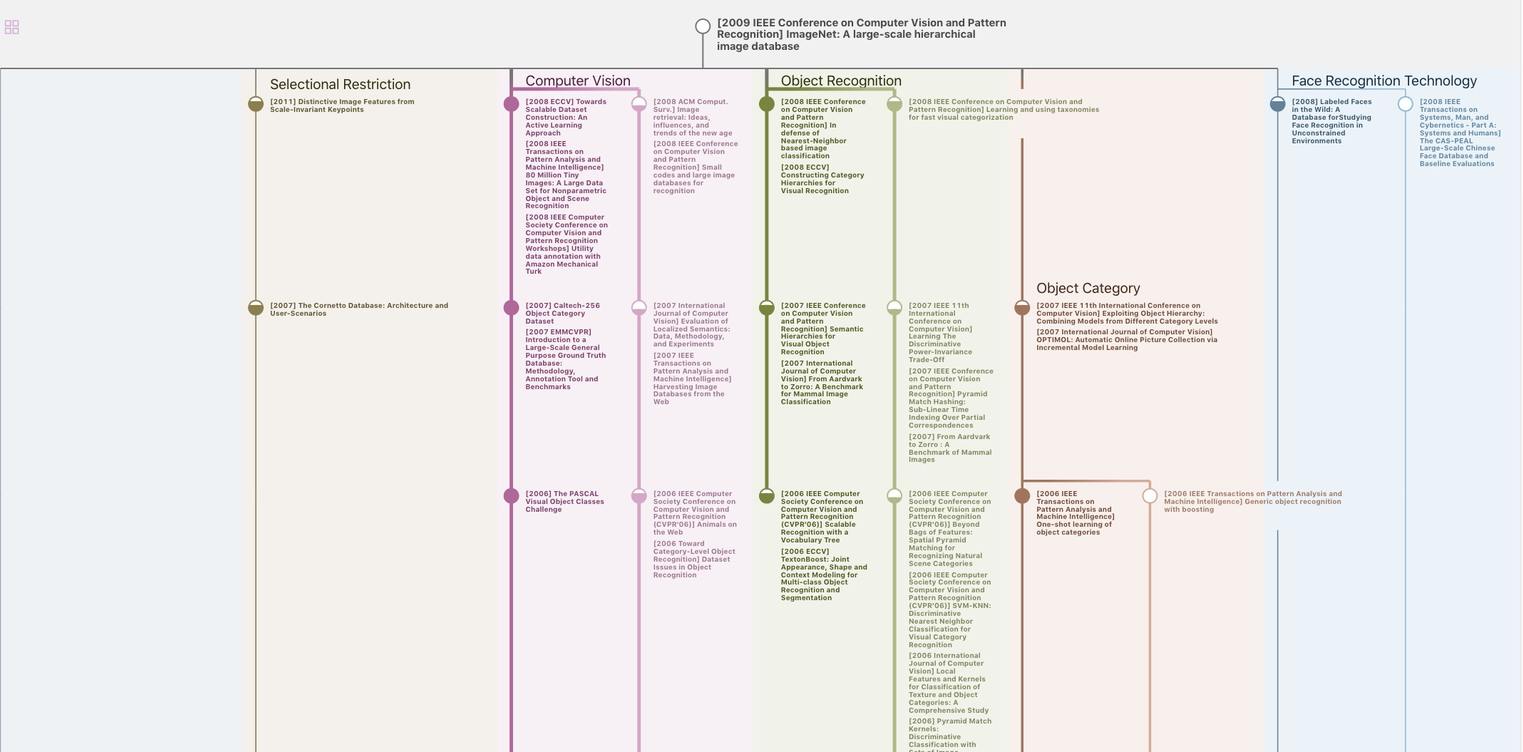
Generate MRT to find the research sequence of this paper
Chat Paper
Summary is being generated by the instructions you defined