Prediction Of Seasonal Urban Thermal Field Variance Index Using Machine Learning Algorithms In Cumilla, Bangladesh
SUSTAINABLE CITIES AND SOCIETY(2021)
Abstract
The intensity and formation of urban heat island (UHI) phenomena are closely related to land use/land cover (LULC) and land surface temperature (LST) change. The effect of UHI can be described quantitatively by urban thermal field variance index (UTFVI). For measuring urban health and ensuring sustainable development, the analysis of LST and UTFVI are receiving boosted attention. This study predicted LULC, seasonal (summer & winter) LST, and UTFVI variations using machine learning algorithms (MLAs) in Cumilla City Corporation (CCC), Bangladesh. Landsat 4-5 TM and Landsat 8 OLI satellite images were used for 1999, 2009, and 2019 to predict future scenarios for 2029 and 2039. MLAs such as Cellular Automata (CA) and Artificial Neural Network (ANN) methods were used to predict the future change in LULC, LST, and UTFVI. The result suggests that, in the year 2029 and 2039, the urban area will likely to be increased by around 8 % and 11 %, where significant decrease will be taken place in green cover by 9 % and 14 %. If the rapid urban growth continues, more than 30 % of the CCC area will likely to be experienced more than 33 degrees C temperature and strongest UTFVI effect in the year 2029 and 2039. In addition, an average 4 degrees C higher LST was recorded in the urban area compared with vegetation cover. In urban construction practice, avoiding concentrated impermeable layers (built-up areas) and increasing green covers, are effective ways of mitigating the effect of UTFVI. This study will contribute in achieving sustainable development and provide useful insights to understand the complex relationship among different elements of urban environments and promotion of city competence.
MoreTranslated text
Key words
Urban heat island, Land cover change, Urban field variance index, Artificial neural network, Cellular automata
AI Read Science
Must-Reading Tree
Example
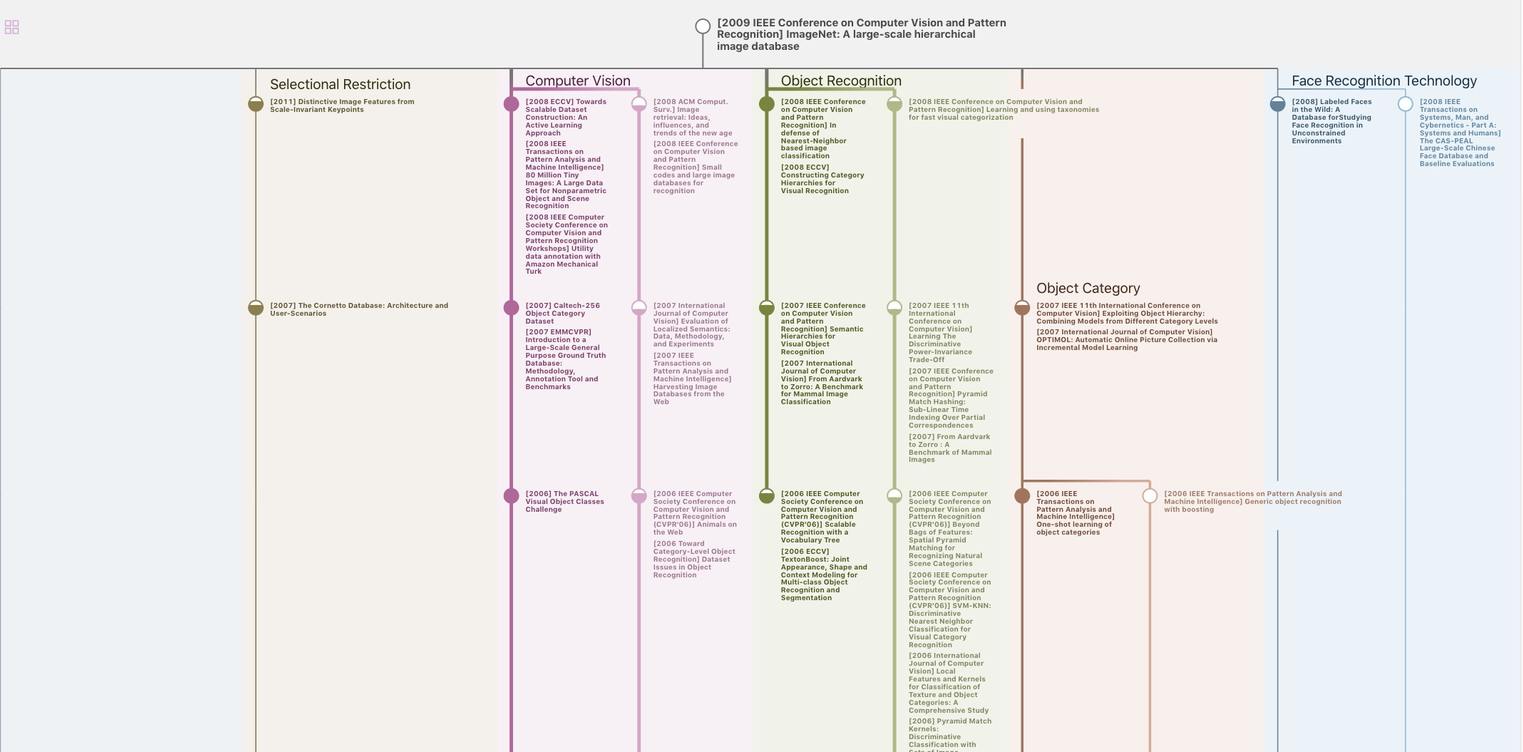
Generate MRT to find the research sequence of this paper
Chat Paper
Summary is being generated by the instructions you defined