Regulatory Genes Identification Within Functional Genomics Experiments For Tissue Classification Into Binary Classes Via Machine Learning Techniques
JOURNAL OF THE PAKISTAN MEDICAL ASSOCIATION(2020)
摘要
Objective: The aim of this study is to filter out the most informative genes that mainly regulate the target tissue class, increase classification accuracy, reduce the curse of dimensionality, and discard redundant and irrelevant genes.Methods:This paper presented the idea of gene selection using bagging sub-forest (BSF).The proposed method provided genes importance grounded on the idea specified in the standard random forest algorithm.The new method is compared with three state-of-the art methods, i.e., Wilcoxon, masked painter and proportional overlapped score (POS).These methods were applied on 5 data sets, i.e. Colon, Lymph node breast cancer, Leukaemia, Serrated colorectal carcinomas, and Breast Cancer. Comparison was done by selecting top 20 genes by applying the gene selection methods and applying random forest (RF) and support vector machine (SVM) classifiers to assess their predictive performance on the datasets with selected genes. Classification accuracy, Brier score, and sensitivity have been used as performance measures.Results: The proposed method gave better results than the other methods using both random forest and SVM classifiers on all the datasets among all the feature selection methods.Conclusion: The proposed method showed improved performance in terms of classification accuracy, Brier score and sensitivity, and hence, could be used as a novel method for gene selection to classify tissue samples into their correct classes.
更多查看译文
关键词
Gene selection, classification, random forest, cancer, microarray gene expression
AI 理解论文
溯源树
样例
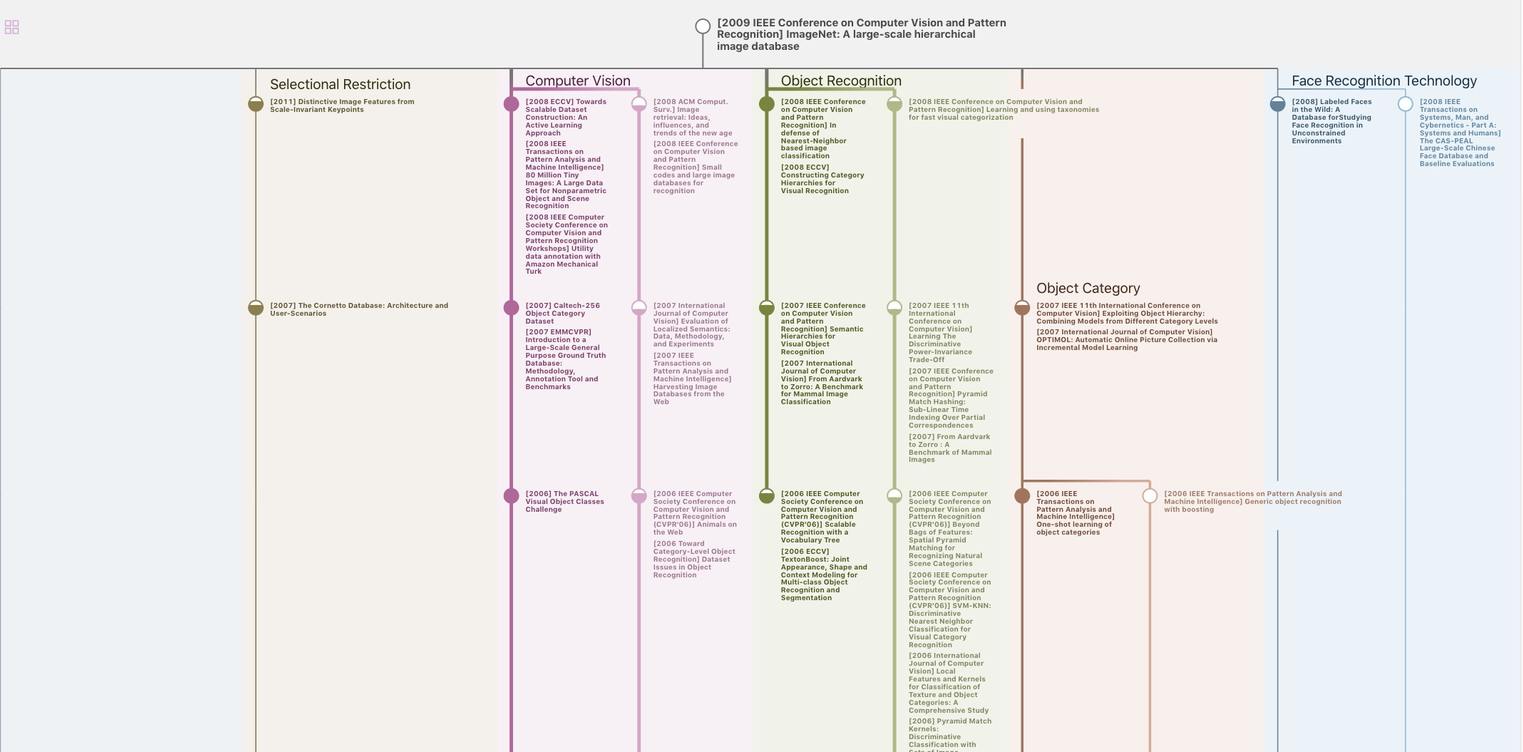
生成溯源树,研究论文发展脉络
Chat Paper
正在生成论文摘要