InPHYNet: Leveraging attention-based multitask recurrent networks for multi-label physics text classification
Knowledge-Based Systems(2021)
摘要
The ability to create and sustain educational infrastructure is a major challenge to nations across the world. Today, information technology is increasingly being used to alleviate this problem by bridging the gap between learners and the textual materials by automating the process of teaching and learning. Due to this, there has been a steep rise in the information need for pedagogical content in recent years. Although there is increasing interest in building question-answering systems, there is a scarcity of intelligent tutoring systems, particularly, in physics education that can aid both students and teachers in secondary education. In this paper, we introduce a novel method for multi-label classification of paragraphs, where the paragraphs are chosen from physics subject of 6th to 12th grades from the curriculum of Central Board of Secondary Education (CBSE), India. This curriculum is common across India. For this purpose, we have constructed an attention-based recurrent interleaved multi-task learning (MTL) network, namely InPHYNet that can be used for any general purpose multi-label classification task related to the educational domain. The proposed solution is contextual and scalable. Although related to physics education, it is generalizable as an approach for other subjects. We perform experiments (i) to verify and validate the labels of data collected, and (ii) to conduct robust analysis of the proposed InPHYNet network. It is observed to yield significant accuracy on the dataset and can be used for any education-based text classification/annotation or as a module within the educational question-answering systems to enhance its quality.
更多查看译文
关键词
Architectures for educational technology system,AI in physics education,Attention-based multitask recurrent network,Multi-label classification
AI 理解论文
溯源树
样例
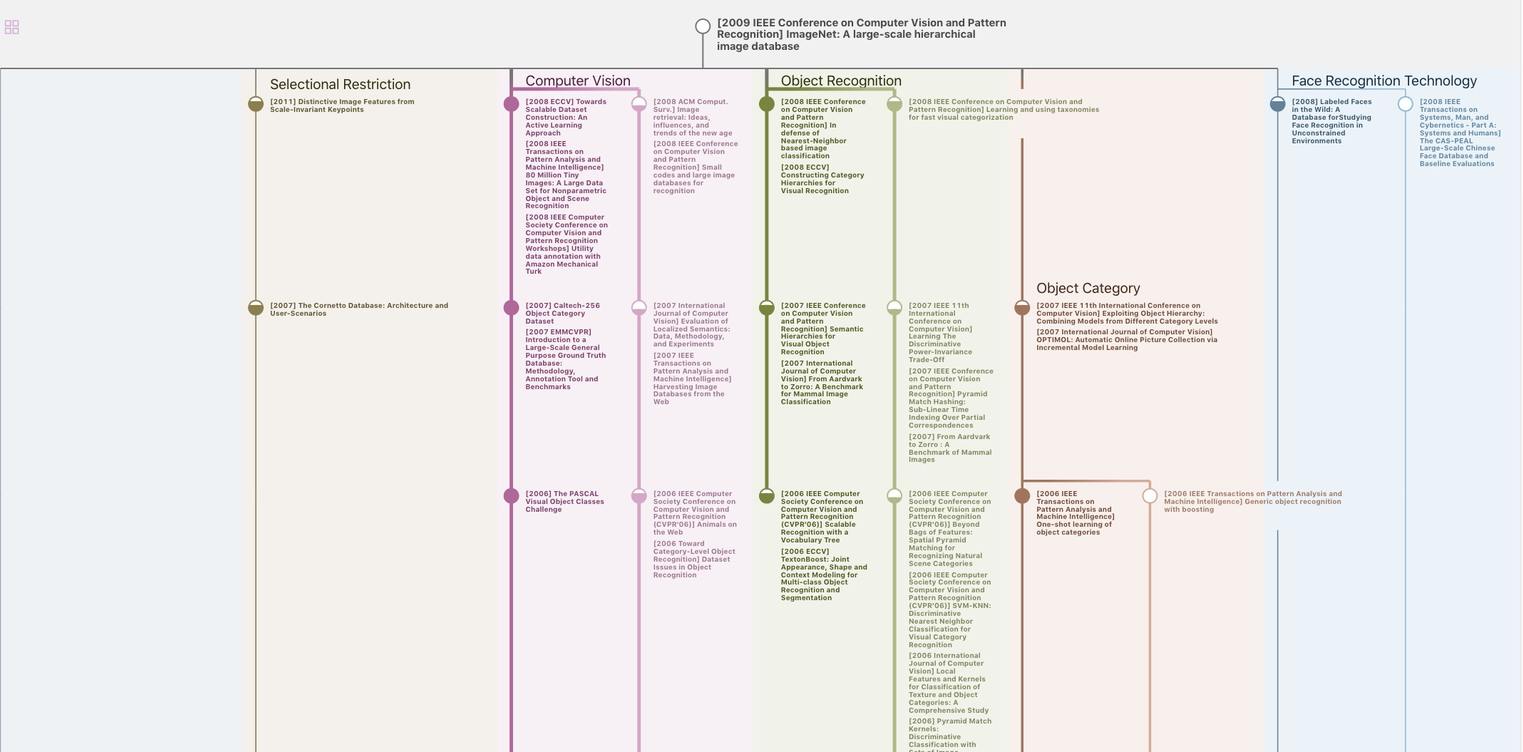
生成溯源树,研究论文发展脉络
Chat Paper
正在生成论文摘要